Documents
Presentation Slides
SEA-NET: SQUEEZE-AND-EXCITATION ATTENTION NET FOR DIABETIC RETINOPATHY GRADING
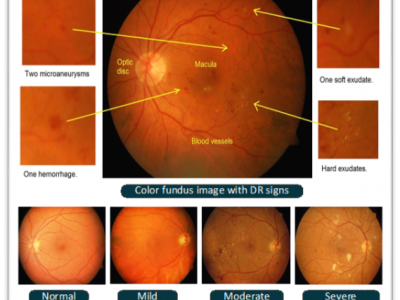
- Citation Author(s):
- Submitted by:
- ZIYUAN ZHAO
- Last updated:
- 20 January 2021 - 7:51am
- Document Type:
- Presentation Slides
- Document Year:
- 2020
- Event:
- Presenters:
- Ziyuan Zhao
- Paper Code:
- 2630
- Categories:
- Log in to post comments
Diabetes is one of the most common disease in individuals. Diabetic retinopathy (DR) is a complication of diabetes, which could lead to blindness. Automatic DR grading based on retinal images provides a great diagnostic and prognostic value for treatment planning. However, the subtle differences among severity levels make it difficult to capture important features using conventional methods. To alleviate the problems, a new deep learning architecture for robust DR grading is proposed, referred to as SEA-Net, in which, spatial attention and channel attention are alternatively carried out and boosted with each other, improving the classification performance. In addition, a hybrid loss function is proposed to further maximize the inter-class distance and reduce the intra-class variability. Experimental results have shown the effectiveness of the proposed architecture.