Documents
Presentation Slides
SINGLE CHANNEL SPEECH SEPARATION WITH CONSTRAINED UTTERANCE LEVEL PERMUTATION INVARIANT TRAINING USING GRID LSTM
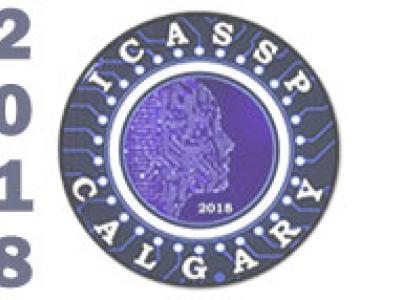
- Citation Author(s):
- Submitted by:
- Chenglin Xu
- Last updated:
- 20 April 2018 - 12:38am
- Document Type:
- Presentation Slides
- Document Year:
- 2018
- Event:
- Presenters:
- CHENGLIN XU
- Paper Code:
- AASP-L1.2
- Categories:
- Log in to post comments
Utterance level permutation invariant training (uPIT) tech- nique is a state-of-the-art deep learning architecture for speaker independent multi-talker separation. uPIT solves the label ambiguity problem by minimizing the mean square error (MSE) over all permutations between outputs and tar- gets. However, uPIT may be sub-optimal at segmental level because the optimization is not calculated over the individual frames. In this paper, we propose a constrained uPIT (cu- PIT) to solve this problem by computing a weighted MSE loss using dynamic information (i.e., delta and acceleration). The weighted loss ensures the temporal continuity of output frames with the same speaker. Inspired by the heuristics (i.e., vocal tract continuity) in computational auditory scene analy- sis, we then extend the model by adding a Grid LSTM layer, that we name it as cuPIT-Grid LSTM, to automatically learn both temporal and spectral patterns over the input magnitude spectrum simultaneously. The experimental results show 9.6% and 8.5% relative improvements on WSJ0-2mix dataset under both closed and open conditions comparing with the uPIT baseline.