Documents
Poster
Texture-Unet: A Texture-Aware Network for Bone Marrow Smear Whole-Slide Image Region of Interest Segmentation
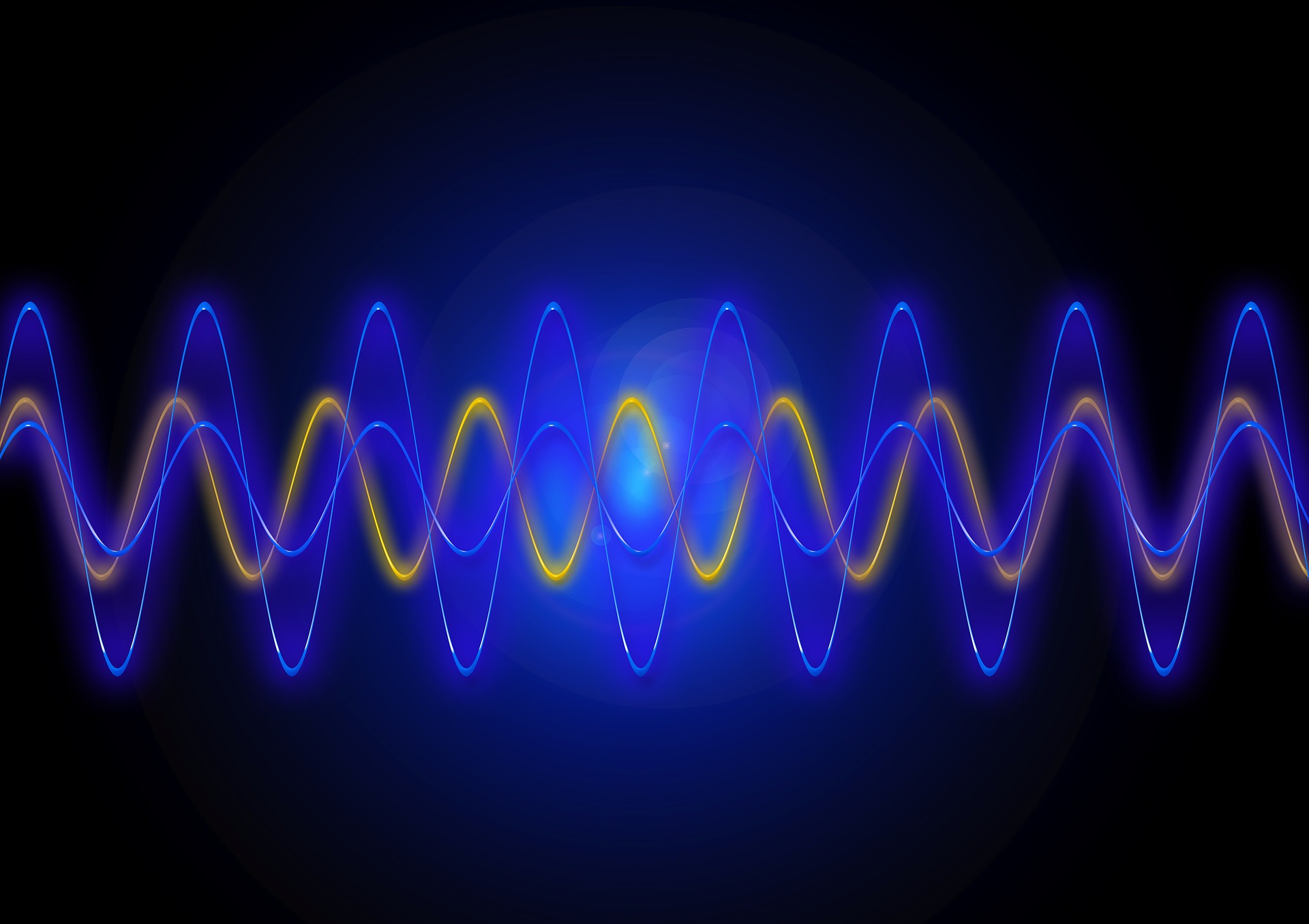
- DOI:
- 10.60864/9ynv-mx88
- Citation Author(s):
- Submitted by:
- Xinrun Chen
- Last updated:
- 6 June 2024 - 10:27am
- Document Type:
- Poster
- Categories:
- Log in to post comments
Bone marrow smear cytology involves observing and analyzing the morphological features of bone marrow cells, and identifying regions of interest (ROI) where the cells are morphologically clear and evenly distributed is a crucial part of this process. However, existing deep learning methods for selecting ROI in whole-slide images (WSI) of bone marrow smears have overlooked the unique characteristics of the smears, particularly the texture information, resulting in inadequate performance. To overcome this issue, this paper proposes a texture-aware region of interest segmentation model (Texture-Unet) for WSI of bone marrow smears. To enhance the network’s ability to extract and perceive texture information, we specifically designed two modules, the Texture Extraction Module (TEM) and the Texture Deep Supervision Module (TDSM). We evaluated our method using a self-constructed dataset. The experimental results indicated a 4.64% improvement in terms of Intersection over Union (IoU), and a 3.04% improvement in terms of the Dice coefficient, compared to the baseline. The experimental results confirm that Texture-Unet performs well in terms of accuracy and efficiency for ROI segmentation in bone marrow smear WSI.
chenjian.pdf
