Documents
Presentation Slides
Patch-based Fully Convolutional Neural Network With Skip Connections For Retinal Blood Vessel Segmentation
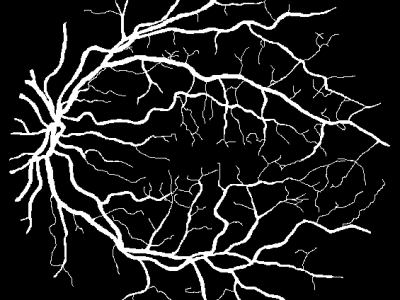
- Citation Author(s):
- Submitted by:
- Zhongwei Feng
- Last updated:
- 14 September 2017 - 4:07am
- Document Type:
- Presentation Slides
- Document Year:
- 2017
- Event:
- Paper Code:
- 2120
- Categories:
- Log in to post comments
Automated segmentation of retinal blood vessels plays an important role in the computer aided diagnosis of retinal diseases. The paper presents a new formulation of patch-based fully Convolutional Neural Networks (CNNs) that allows accurate segmentation of the retinal blood vessels. A major modification in this retinal blood vessel segmentation task is to improve and speed-up the patch-based fully CNN training by local entropy sampling and a skip CNN architecture with class-balancing loss. The proposed method is experimented on DRIVE dataset and achieves strong performance and significantly outperforms the-state-of-the-art for retinal blood vessel segmentation with 78.11% sensitivity, 98.39% specificity, 95.60% accuracy, 87.36% precision and 97.92% AUC score respectively.
icip2017.pdf
