Documents
Poster
Poster
MULTI-SCENARIO DEEP LEARNING FOR MULTI-SPEAKER SOURCE SEPARATION
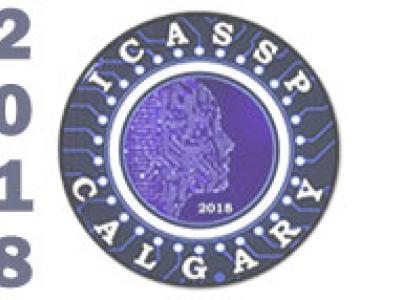
- Citation Author(s):
- Submitted by:
- Jeroen Zegers
- Last updated:
- 13 April 2018 - 4:58am
- Document Type:
- Poster
- Document Year:
- 2018
- Event:
- Presenters:
- Jeroen Zegers
- Paper Code:
- 4287
- Categories:
- Log in to post comments
Research in deep learning for multi-speaker source separation has received a boost in the last years. However, most studies are restricted to mixtures of a specific number of speakers, called a specific scenario. While some works included experiments for different scenarios, research towards combining data of different scenarios or creating a single model for multiple scenarios have been very rare. In this work it is shown that data of a specific scenario is relevant for solving another scenario. Furthermore, it is concluded that a single model, trained on different scenarios is capable of matching performance of scenario specific models.