Documents
Poster
Poster
Mutual-Information-Private Online Gradient Descent Algorithm
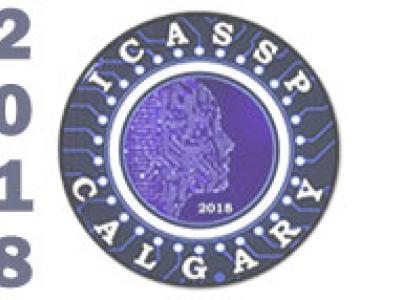
- Citation Author(s):
- Submitted by:
- Ruochi Zhang
- Last updated:
- 23 April 2018 - 1:52am
- Document Type:
- Poster
- Event:
- Categories:
- Log in to post comments
A user implemented privacy preservation mechanism is proposed for the online gradient descent (OGD) algorithm. Privacy is measured through the information leakage as quantified by the mutual information between the usersʼ outputs and learnerʼs inputs. The input perturbation mechanism proposed can be implemented by individual users with a space and time complexity that is independent of the horizon T. For the proposed mechanism, the information leakage is shown to be bounded by the Gaussian channel capacity in the full information setting. The regret bound of the privacy preserving learning mechanism is identical to the non private OGD with only differing in constant factors.
newfile5.pdf
