Documents
Presentation Slides
Improving Universal Sound Separation Using Sound Classification Presentation
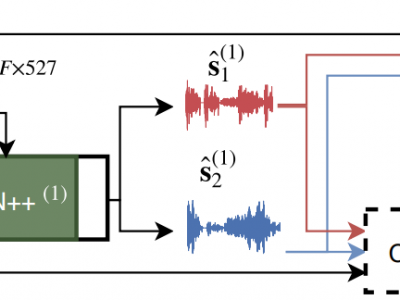
- Citation Author(s):
- Submitted by:
- Efthymios Tzinis
- Last updated:
- 3 May 2020 - 10:09pm
- Document Type:
- Presentation Slides
- Document Year:
- 2020
- Event:
- Presenters:
- Efthymios Tzinis
- Paper Code:
- WE2.L2.2
- Categories:
- Log in to post comments
Deep learning approaches have recently achieved impressive performance on both audio source separation and sound classification. Most audio source separation approaches focus only on separating sources belonging to a restricted domain of source classes, such as speech and music. However, recent work has demonstrated the possibility of "universal sound separation", which aims to separate acoustic sources from an open domain, regardless of their class. In this paper, we utilize the semantic information learned by sound classifier networks trained on a vast amount of diverse sounds to improve universal sound separation. In particular, we show that semantic embeddings extracted from a sound classifier can be used to condition a separation network, providing it with useful additional information. This approach is especially useful in an iterative setup, where source estimates from an initial separation stage and their corresponding classifier-derived embeddings are fed to a second separation network. By performing a thorough hyperparameter search consisting of over a thousand experiments, we find that classifier embeddings from clean sources provide nearly one dB of SNR gain, and our best iterative models achieve a significant fraction of this oracle performance, establishing a new state-of-the-art for universal sound separation.