Documents
Presentation Slides
General Speech Restoration Using Two-stage Generative Adversarial Networks (slides)
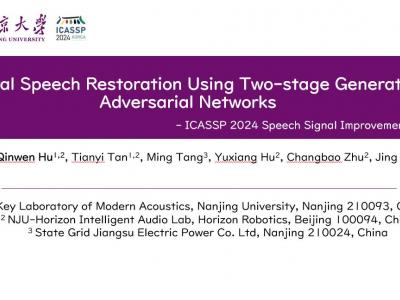
- DOI:
- 10.60864/nq3w-6j44
- Citation Author(s):
- Submitted by:
- qinwen hu
- Last updated:
- 6 June 2024 - 10:27am
- Document Type:
- Presentation Slides
- Categories:
- Log in to post comments
General speech restoration is a challenging task, which requires removing multiple types of distortions within a single system. The prevailing methods for general speech restoration largely rely on generative models, leveraging their ability to generate speech components based on prior knowledge of clean speech characteristics. Our approach adopts a two-stage processing scheme, comprising a speech restoration module and a speech enhancement module. The restoration module utilizes dilated convolutional networks and is trained using LSGAN losses. In contrast, the speech enhancement module employs a convolutional-recurrent network and is trained using metric-GAN losses. The proposed system achieves an overall opinion score (MOS) of 2.944 and a final score of 0.6805, ranking 3rd in Track 1 of the ICASSP 2024 Speech Signal Improvement Challenge (SIG-2)