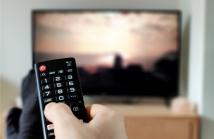
- Read more about Keyframe Insertion for Fast Channel Switching & Packet-Loss Repair in Low-Delay Live Streaming
- Log in to post comments
Keyframe insertion is a solution for fast channel switching and packet-loss repair in low-delay live streaming.
This work lists the requirements of keyframe insertion in three generations of video coding standards (H.264/AVC, H.265/HEVC, and H.266/VVC), and analyzes the quality impact.
- Categories:
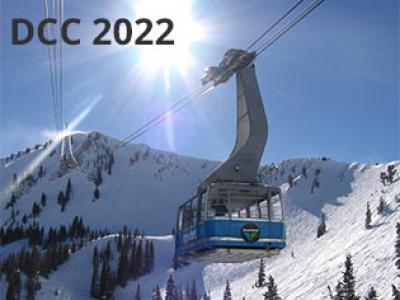
- Read more about An Improved Multi-reference Frame Loop Filter Algorithm Based on Transformer for VVC
- Log in to post comments
Deep learning methods have been achieving good results at the in-loop filtering stage in Versatile Video Coding(VVC). The Multi-Frame In-Loop Filter of HEVC (MIF) algorithm is one of the networks that effectively utilizes the multiple reference frames to enhance the quality of reconstruction. However, it has the disadvantages of low efficiency of the reference frame selection, and its quality enhancement network does not fully utilize the inter-frame correlations of the video sequence and has large redundancy.
- Categories:
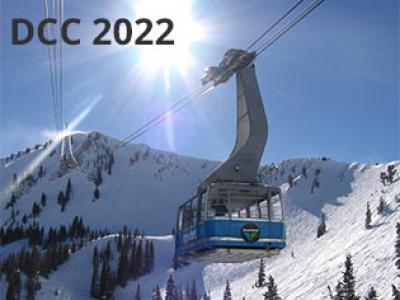
- Read more about A fast geometric prediction merge mode decision algorithm based on CU gradient for VVC
- Log in to post comments
Geometric prediction merge mode (GPM) is a new tool introduced in the inter-prediction of Versatile Video Coding (VVC), which uses non-rectangular block partitions for coding unit (CU) partition to improve coding performance. To address the problem of large computational redundancy in the geometric prediction merge mode with motion vector refinement (GPM with MMVD), in this paper, a new decision algorithm is proposed based on CU gradient.
- Categories:
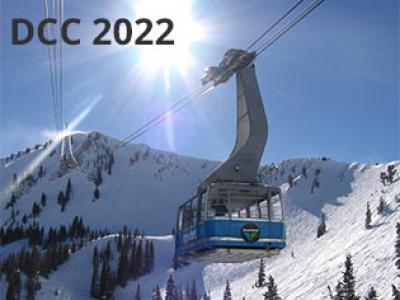
- Read more about A Fast Multi-tree Partition Algorithm Based on Spatial-temporal Correlation for VVC
- Log in to post comments
The quadtree nested multi-type trees (MTT) partitioning scheme is adopted by the latest generation of video coding standard H.266/VVC. While the performance of the encoder is improved, the complexity has increased by 2.2-5.6 times. It has been found that in the inter-coding mode, blocks with intense motion or complex texture tend to be further divided by the encoder. However, in the existing multi-tree partition algorithms, only the inter-frame motion information or intra-frame texture information has been utilized during partition.
- Categories:
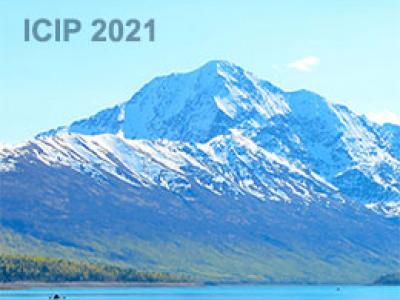
- Read more about An Efficient Image Compression Method Based On Neural Network: An Overfitting Approach
- Log in to post comments
Over the past decade, nonlinear image compression techniques based on neural networks have been rapidly developed to achieve more efficient storage and transmission of images compared with conventional linear techniques. A typical non-linear technique is implemented as a neural network trained on a vast set of images, and the latent representation of a target image is transmitted. In contrast to the previous nonlinear techniques, we propose a new image compression method in which a neural network model is trained exclusively on a single target image, rather than a set of images.
slides.pdf

- Categories:
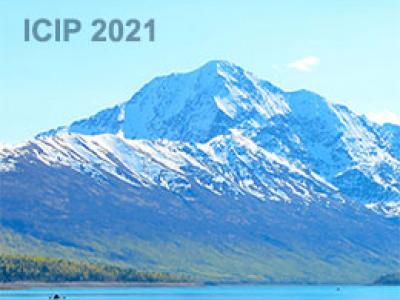
- Read more about Deep Video Compression for Interframe Coding
- Log in to post comments
A typical learning-based video compression scheme consists of motion coding and residual coding. In this paper, our deep video compression features a motion predictor and a refinement networks for interframe coding. To save the bits for transmitting motion information, our scheme performs local motion prediction and sends only the differential motion vectors to the decoder. In the residual coding, we couple the residual decoder with the refine-net to reduce residual signal bits.
- Categories:
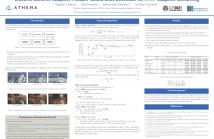
- Read more about Efficient Content-Adaptive Feature-based Shot Detection for HTTP Adaptive Streaming
- Log in to post comments
Video delivery over the Internet has been becoming a commodity in recent years, owing to the widespread use of Dynamic Adaptive Streaming over HTTP(DASH). The DASH specification defines a hierarchical data model for Media Presentation Descriptions (MPDs) in terms of segments. This paper focuses on segmenting video into multiple shots for encoding in Video on Demand (VoD) HTTP Adaptive Streaming (HAS) applications.
- Categories:
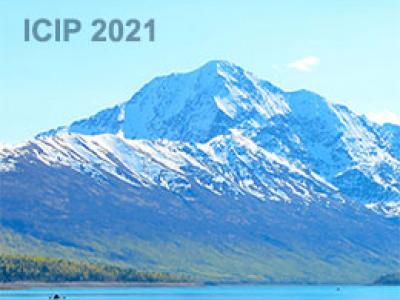
- Read more about INTERFRAME-DEPENDENT RATE-QP-DISTORTION MODEL FOR VIDEO CODING AND TRANSMISSION
- Log in to post comments
- Categories:
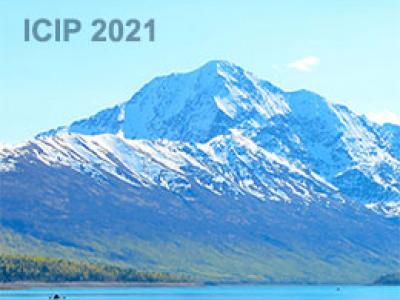
- Read more about INTERFRAME-DEPENDENT RATE-QP-DISTORTION MODEL FOR VIDEO CODING AND TRANSMISSION
- Log in to post comments
- Categories: