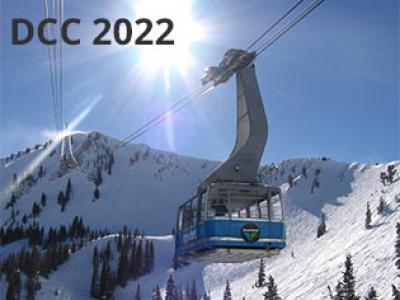
- Read more about Analysis on Compressed Domain: A Multi-Task Learning Approach
- 2 comments
- Log in to post comments
Image compression approaches based on deep learning have achieved remarkable success.
Existing studies mainly focus on human vision and machine analysis tasks taking reconstructed images as input.
- Categories:
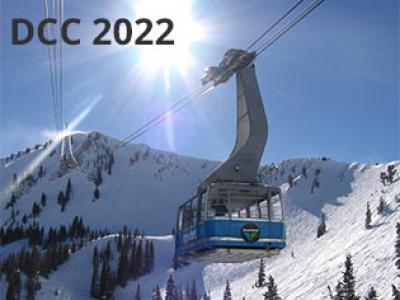
- Read more about Entropy Modeling via Gaussian Process Regression for Learned Image Compression
- Log in to post comments
- Categories:
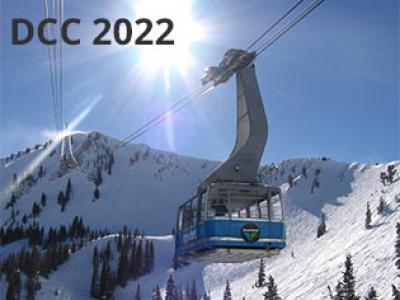
Block-based compressive imaging enables new video acquisition methodology while reducing raw data size, theoretically eliminating the need for complex coding algorithms. When transmitting raw data, however, the redundancy associated with random projection remains. This paper takes a fresh look at raw data structure by viewing it as cube made up of multiple downsampled images rather than a vector. As a result, we can view each individual data point as a pixel, allowing us to code more flexibly and versatility than state-of-the-art works.
- Categories:
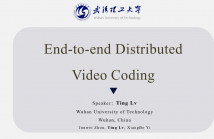
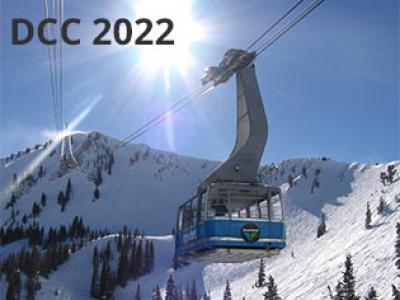
- Read more about Rate Distortion Characteristic Modeling for Neural Image Compression
- Log in to post comments
- Categories:
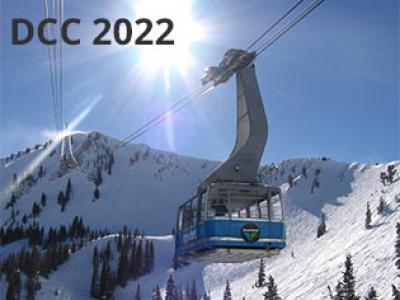
- Read more about Fast Partition Mode Decision via a Plug-in Fully Connected Network for Video Coding
- Log in to post comments
FCN-Pre.pdf

- Categories:
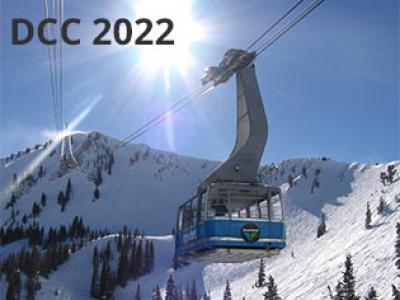
- Read more about Hyperspectral remote sensing data compression with neural networks
- Log in to post comments
We propose a novel approach to compress hyperspectral remote sensing images using convo- lutional neural networks, aimed at producing compression results competitive with common lossy compression standards such as JPEG 2000 and CCSDS 122.1-B-1 with a system far less complex than equivalent neural-network codecs used for natural images. Our method consists of a collection of smaller networks which compress the image band-by-band taking advantage of the very high similarity between bands on certain intervals.
- Categories:
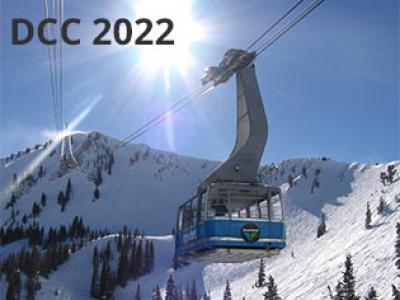
- Read more about Interpretable Learned Image Compression: A Frequency Transform Decomposition Perspective
- 1 comment
- Log in to post comments
Image compression is a key problem in this age of information explosion. With the help of machine learning, recent studies have shown that learning-based image compression methods tend to surpass traditional codecs. Image compression can be split into three steps: transform, quantization, and entropy estimation.
- Categories: