- Acoustic Modeling for Automatic Speech Recognition (SPE-RECO)
- General Topics in Speech Recognition (SPE-GASR)
- Large Vocabulary Continuous Recognition/Search (SPE-LVCR)
- Lexical Modeling and Access (SPE-LEXI)
- Multilingual Recognition and Identification (SPE-MULT)
- Resource constrained speech recognition (SPE-RCSR)
- Robust Speech Recognition (SPE-ROBU)
- Speaker Recognition and Characterization (SPE-SPKR)
- Speech Adaptation/Normalization (SPE-ADAP)
- Speech Analysis (SPE-ANLS)
- Speech Coding (SPE-CODI)
- Speech Enhancement (SPE-ENHA)
- Speech Perception and Psychoacoustics (SPE-SPER)
- Speech Production (SPE-SPRD)
- Speech Synthesis and Generation, including TTS (SPE-SYNT)
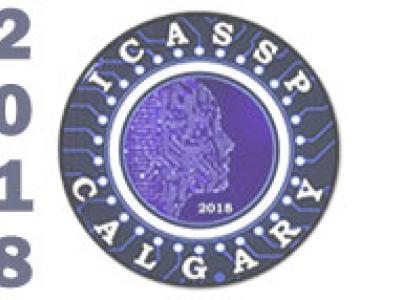
We propose a novel adversarial multi-task learning scheme, aiming at actively curtailing the inter-talker feature variability while maximizing its senone discriminability so as to enhance the performance of a deep neural network (DNN) based ASR system. We call the scheme speaker-invariant training (SIT). In SIT, a DNN acoustic model and a speaker classifier network are jointly optimized to minimize the senone (tied triphone state) classification loss, and simultaneously mini-maximize the speaker classification loss.
- Categories:
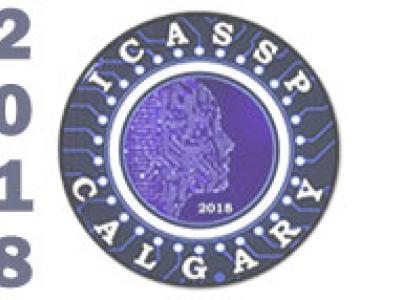
- Read more about Adversarial Teacher-Student Learning for Unsupervised Adaptation
- Log in to post comments
The teacher-student (T/S) learning has been shown effective in unsupervised domain adaptation ts_adapt. It is a form of transfer learning, not in terms of the transfer of recognition decisions, but the knowledge of posteriori probabilities in the source domain as evaluated by the teacher model. It learns to handle the speaker and environment variability inherent in and restricted to the speech signal in the target domain without proactively addressing the robustness to other likely conditions. Performance degradation may thus ensue.
- Categories:
- Read more about A PLLR and Multi-stage Staircase Regression Framework for Speech-based Emotion Prediction
- Log in to post comments
Continuous prediction of dimensional emotions (e.g. arousal and valence) has attracted increasing research interest recently. When processing emotional speech signals, phonetic features have been rarely used due to the assumption that phonetic variability is a confounding factor that degrades emotion recognition/prediction performance. In this paper, instead of eliminating phonetic variability, we investigated whether Phone Log-Likelihood Ratio (PLLR) features could be used to index arousal and valence in a pairwise low/high framework.
- Categories:
- Categories:
- Read more about Automatic Speech Emotion Recognition Using Recurrent Neural Networks with Local Attention
- Log in to post comments
Automatic emotion recognition from speech is a challenging task which relies heavily on the effectiveness of the speech features used for classification. In this work, we study the use of deep learning to automatically discover emotionally relevant features from speech. It is shown that using a deep recurrent neural network, we can learn both the short-time frame-level acoustic features that are emotionally relevant, as well as an appropriate temporal aggregation of those features into a compact utterance-level representation.
icassp2017.pdf

- Categories:
- Read more about An Initial Study of Indonesian Semantic Role Labelingand Its Application on Event Extraction
- Log in to post comments
Semantic role labeling (SRL) is a task to as- sign semantic role labels to sentence elements. This pa- per describes the initial development of an Indonesian semantic role labeling system and its application to extract event information from Tweets. We compare two feature types when designing the SRL systems: Word-to-Word and Phrase-to-Phrase. Our experiments showed that the Word- to-Word feature approach outperforms the Phrase-to-Phrase approach. The application of the SRL system to an event extraction problem resulted overlap-based accuracy of 0.94 for the actor identification.
- Categories:
- Read more about An Investigation of Adaptation Techniques for Building Acoustic Models for Hearing-impaired Children in a CAPT Application
- Log in to post comments
- Categories:
- Read more about Speaker Diarization System for Autism Children’s Real-Life Audio Data
- Log in to post comments
- Categories:
- Read more about Perceptual Evaluation of Natural and Synthesized Speech with Prosodic Focus in Mandarin Production of American Learners
- Log in to post comments
Natural and synthesized speech in L2 Mandarin produced by American English learners was evaluated by native Mandarin speakers to identify focus status and rate the naturalness of the speech. The results reveal that natural speech was recognized and rated better than synthesized speech, early learners’ speech better than late learners’ speech, focused sentences better than no-focus sentences, and initial focus and medial focus better than final focus. Tones of in-focus words interacted with focus status of the sentence and speaker group.
- Categories: