Documents
Poster
Reducing motion artifacts in brain MRI using vision transformers and self-supervised learning
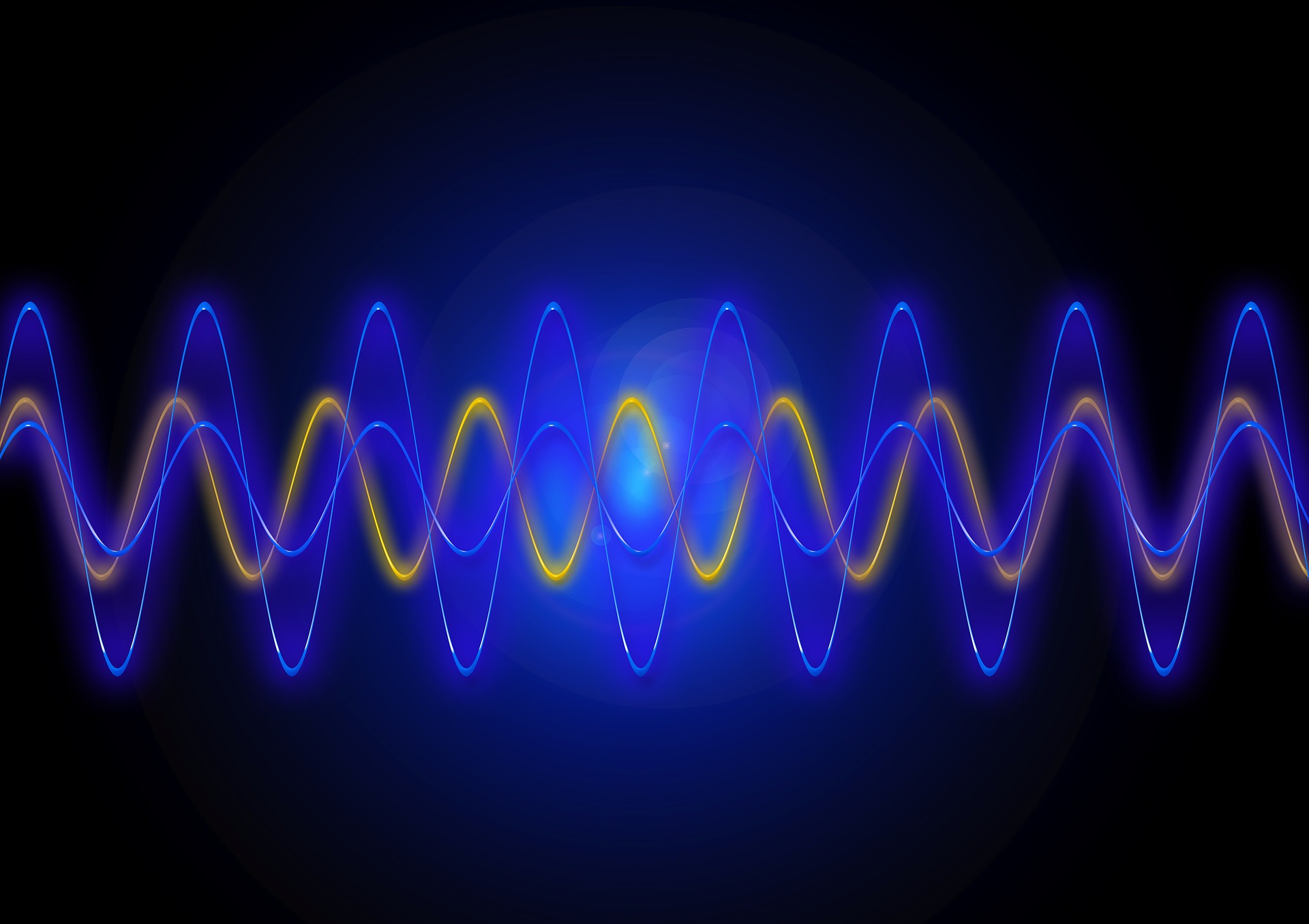
- DOI:
- 10.60864/7at2-av96
- Citation Author(s):
- Submitted by:
- Lei Zhang
- Last updated:
- 8 November 2024 - 1:06pm
- Document Type:
- Poster
- Categories:
- Log in to post comments
Vision Transformer (ViT) has become a state-of-art in many vision tasks, owing to its great scalability and promising performance. In Magnetic Resonance Imaging (MRI), motion continues to be a major problem, which degrades the image quality and the corresponding disease assessment. The purpose of this work is to assess a ViT-based MRI motion correction method. Self-supervised learning was further incorporated to enhance the motion correction effects. Training image pairs were generated starting with in-house MRI data of high quality, from which simulated images with artifacts were generated using a k-space resampling algorithm based on real head movements. We randomly mask 50% patches of the input image and reconstruct the missing pixels as self-supervised pre-training to boost performance of the ViT model. The output images of the proposed method from motion-corrupted data had significantly improved image quality compared with the original corrupted images and are better than the results of a previous deep learning-based motion correction algorithm: the UNet-based MC-Net and a baseline ViT based method in terms of quantitative metrics. This study offers a practical approach for elimination of motion artifacts from brain MRI, using self-supervised learning and ViT.
Comments
This is the poster presented
This is the poster presented in the ICIP 2024 conference. If you find this poster is useful, please cite our paper.