Documents
Poster
2D Human Pose Estimation Calibration and Keypoint Visibility Classification
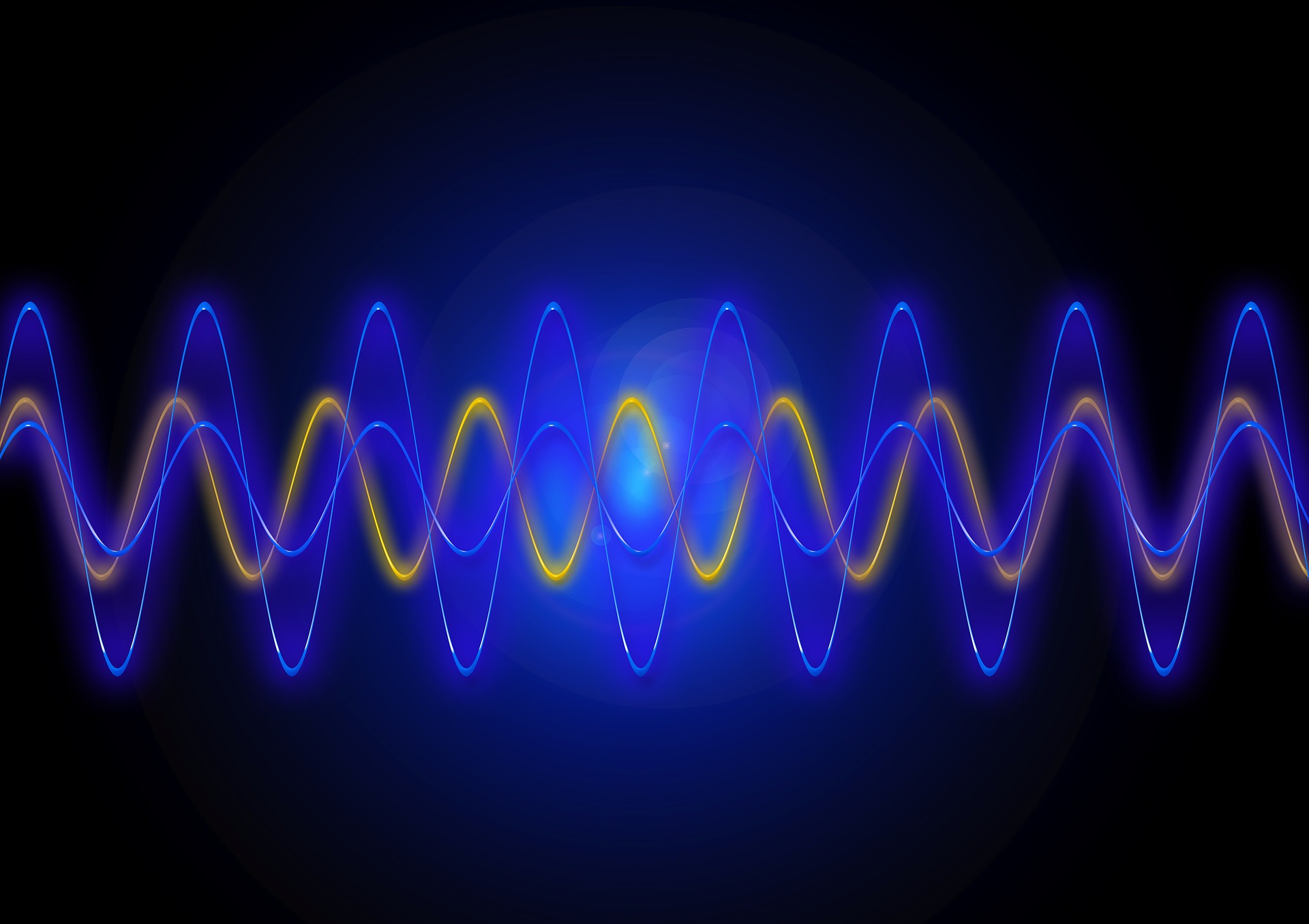
- Citation Author(s):
- Submitted by:
- Zhongyu Jiang
- Last updated:
- 7 April 2024 - 5:50pm
- Document Type:
- Poster
- Categories:
- Log in to post comments
The confidence scores of 2D pose estimation are widely utilized in various fields, including multi-view 3D human pose estimation, skeleton-based human tracking, human action recognition, human re-identification, etc. Despite widespread use, confidence scores from 2D pose estimation methods are unreliable in indicating the accuracy of estimation results, particularly in occlusion situations, i.e., keypoints with high confidence scores may have low accuracy and vice versa. To address this issue, we propose a new 2D human pose estimation calibration method in this paper. Our method not only enhances the accuracy of 2D pose estimation but also aligns the confidence scores with the quality and visibility of keypoints. We achieve 77.6 mAP in the COCO val dataset, compared with 76.5 mAP of the original HRNet. For keypoint visibility prediction, we can reach 89.4% accuracy, 87.6% precision, and 97.0% recall in the COCO val dataset.