Documents
Presentation Slides
3D Point Cloud Enhancement using Graph-Modelled Multiview Depth Measurements
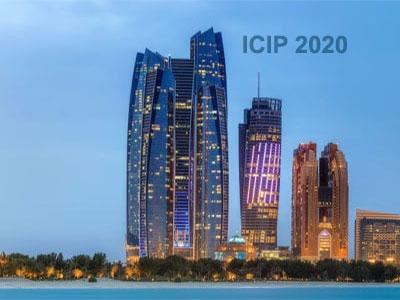
- Citation Author(s):
- Submitted by:
- Xue Zhang
- Last updated:
- 2 November 2020 - 11:51am
- Document Type:
- Presentation Slides
- Document Year:
- 2020
- Event:
- Categories:
- Log in to post comments
A 3D point cloud is often synthesized from depth measurements collected by sensors at different viewpoints. The acquired measurements are typically both coarse in precision and corrupted by noise. To improve quality, previous works denoise a synthesized 3D point cloud a posteriori, after projecting the imperfect depth data onto the 3D space. Instead, we enhance depth measurements on the sensed images a priori, exploiting inherent 3D geometric correlation across views, before synthesizing a 3D point cloud from the improved measurements. By enhancing closer to the actual sensing process, we benefit from optimization targeting specifically the depth image formation model, before subsequent processing steps that can further obscure measurement errors. Mathematically, for each pixel row in a pair of rectified viewpoint depth images, we first construct a graph reflecting inter-pixel similarities via metric learning using data in previous enhanced rows. To optimize left and right viewpoint images simultaneously, we write a non-linear mapping function from left pixel row to the right based on 3D geometry relations. We formulate a MAP optimization problem, which, after suitable linear approximations, results in an unconstrained convex and differentiable objective, solvable using fast gradient method (FGM). Experimental results show that our method noticeably outperforms recent denoising algorithms