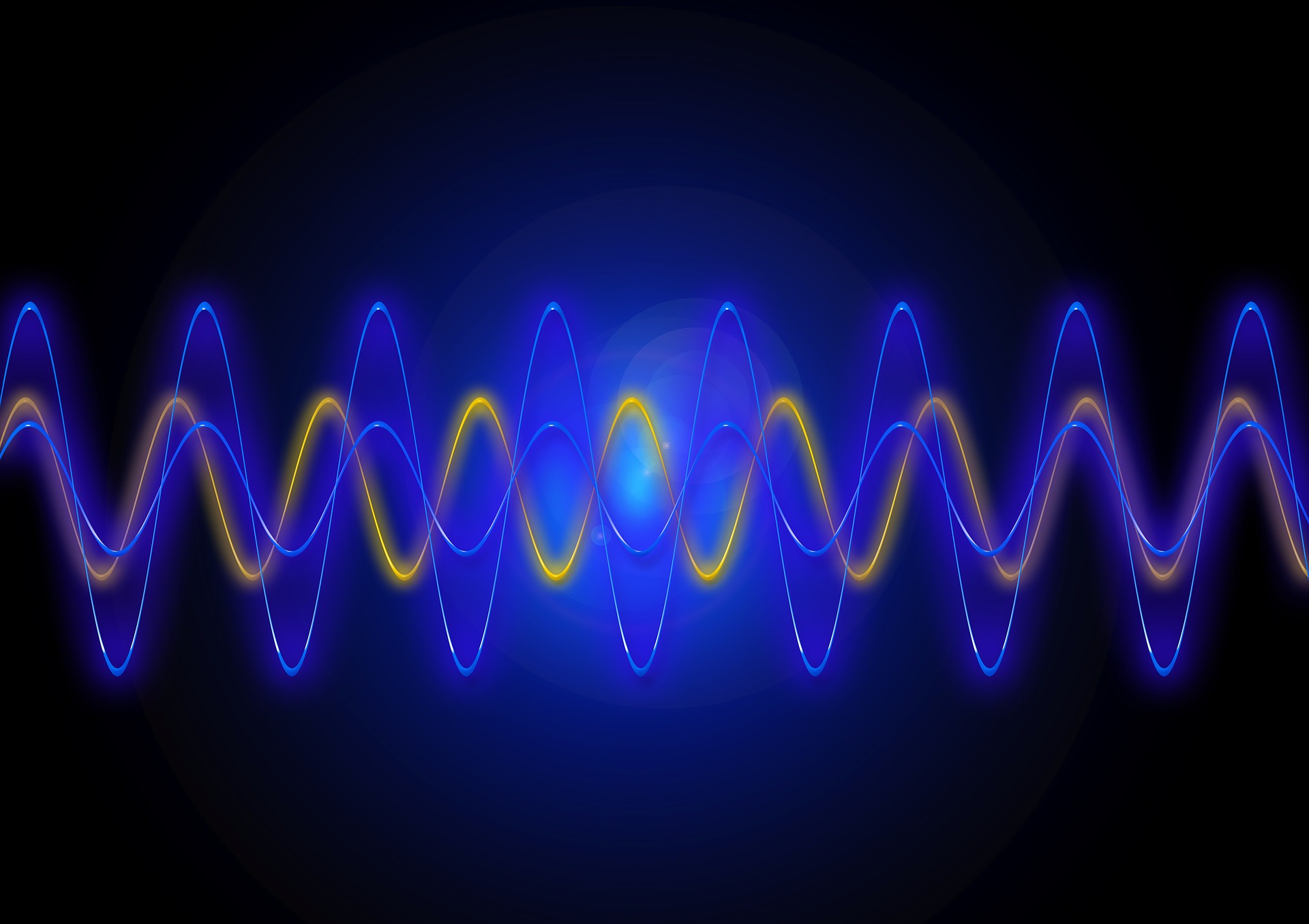
- Read more about Supplementary Materials for F-PTQ
- Log in to post comments
This is the Supplementary Material for the manuscript titled as "F-LBQ: Fine-grained Low Bit Quantization for Efficient and Accurate Object Detection" in submission to ICIP 2025.
The pdf contains implementation details and auxiliary experimental discussions.
- Categories:
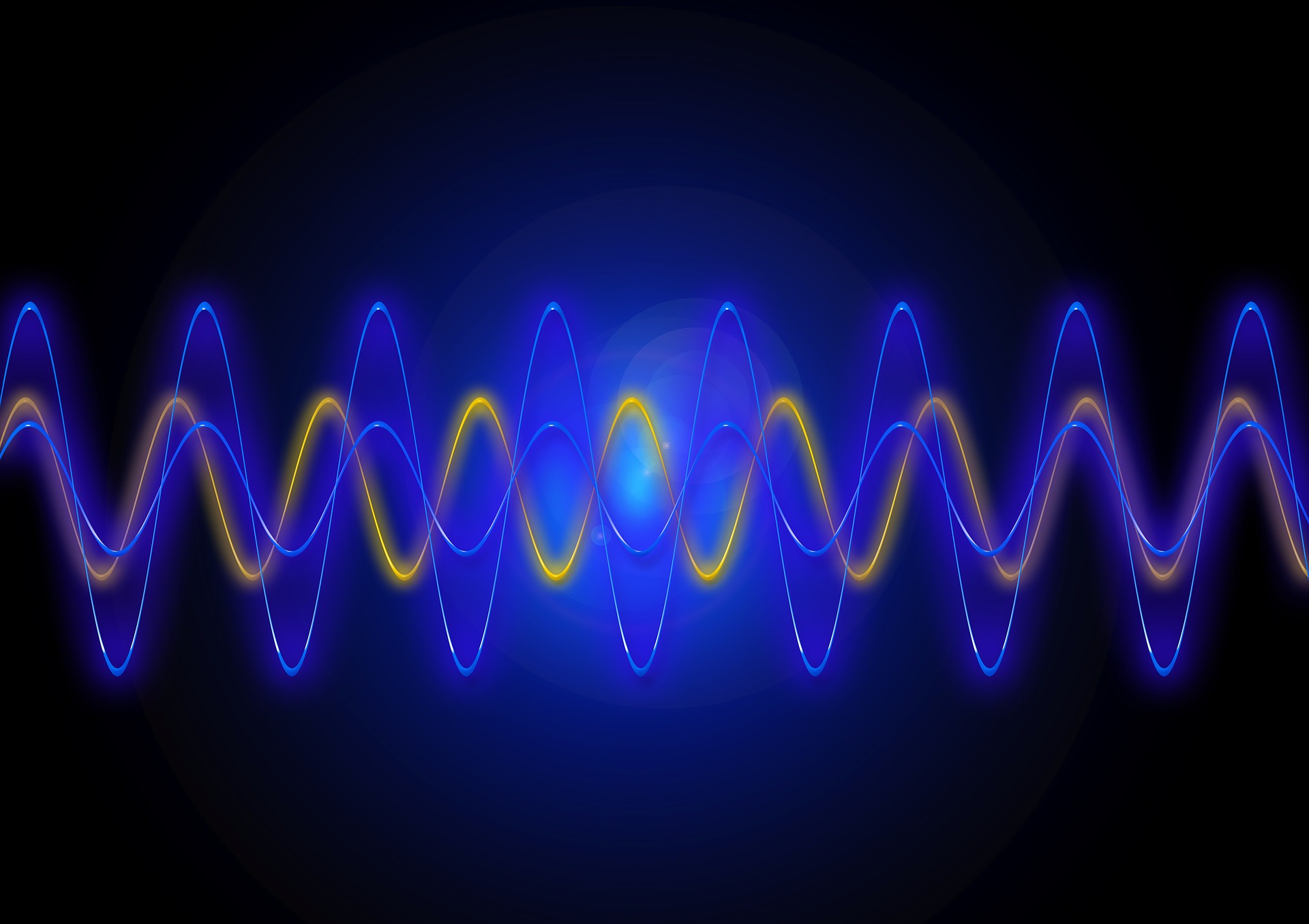
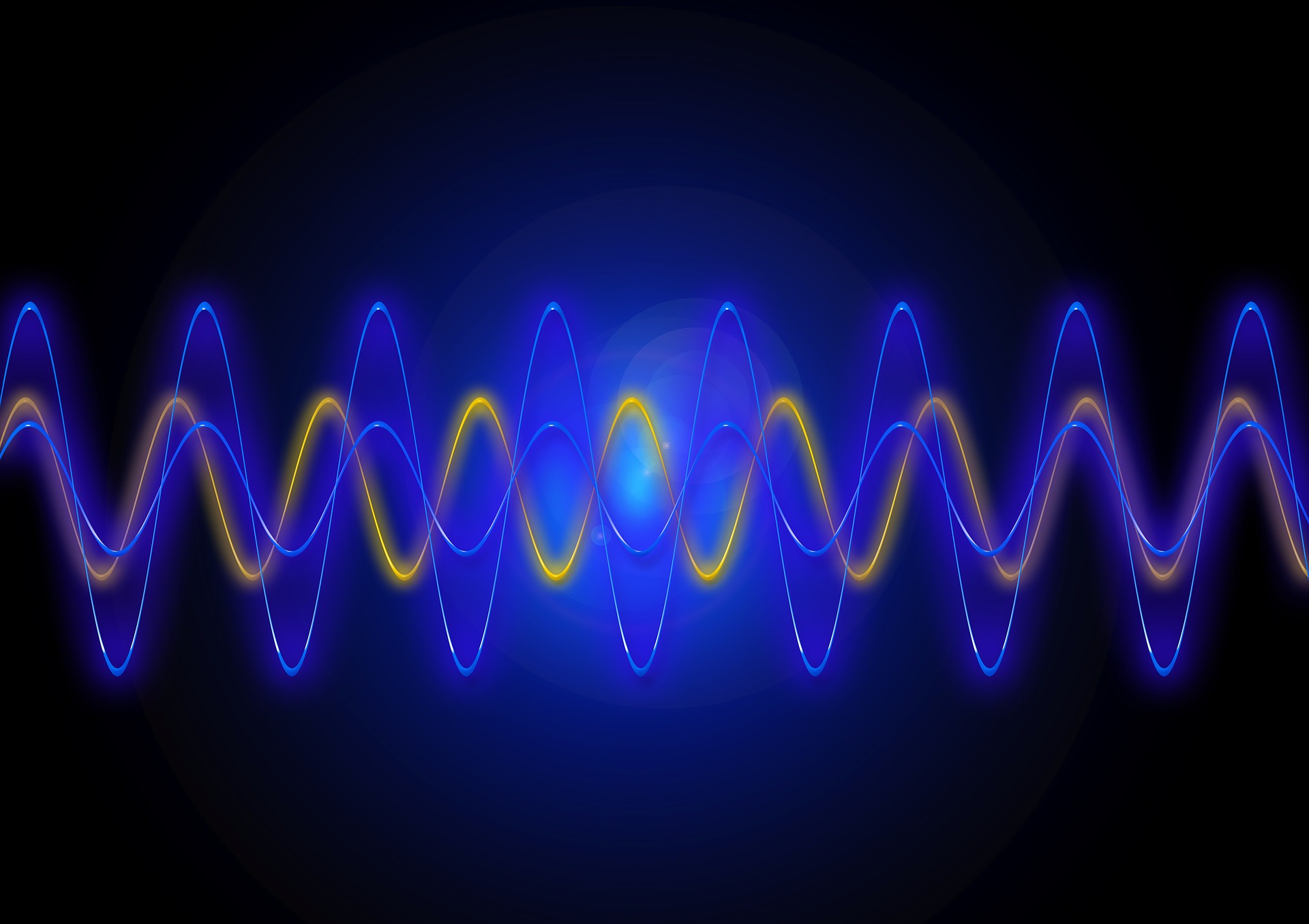
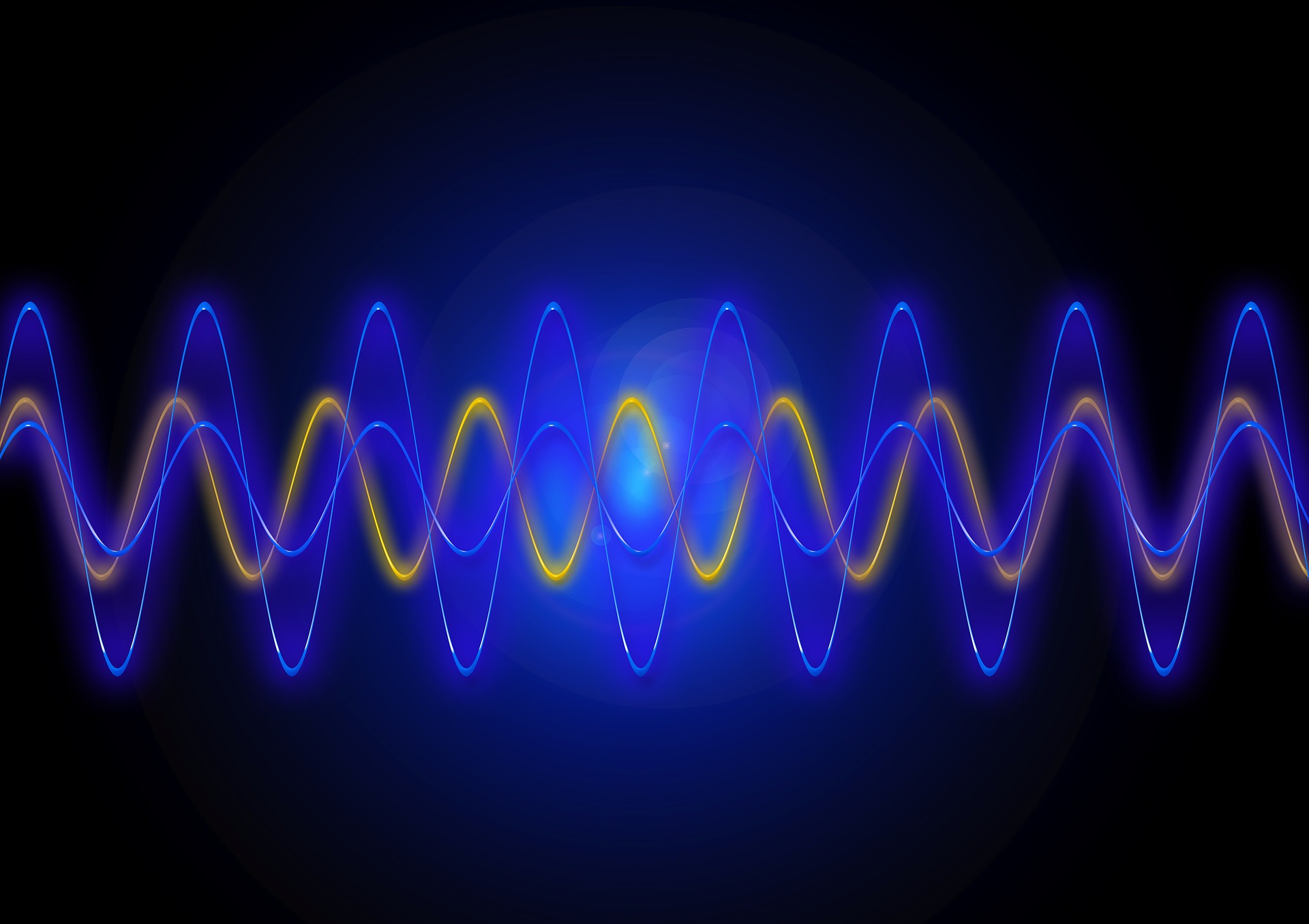
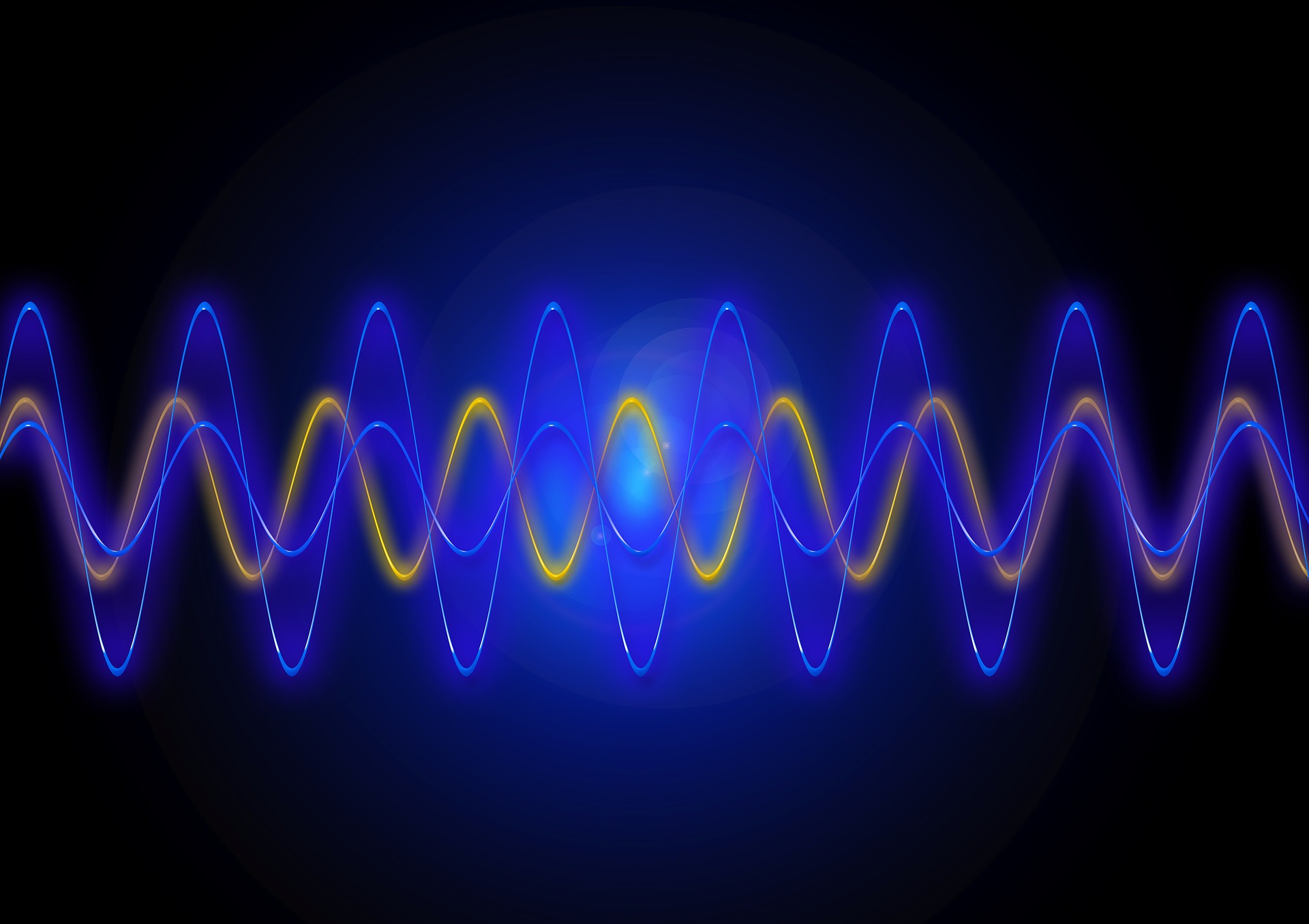
- Read more about FACE QUALITY TRANSFORMER: A FACE QUALITY ASSESSMENT AND ENHANCEMENT FRAMEWORK
- Log in to post comments
The quality of image generation has reached impressive levels.
Advanced text-to-image models have become
amazingly good at creating objects, depicting actions with high precision.
However, despite significant progress in image generation,
the quality of generated faces remains a
critical factor for users. Even the most advanced text-to-image
diffusion models struggle to generate high quality faces consistently. This
highlights the importance of estimation of face quality in generated images as one of
- Categories:
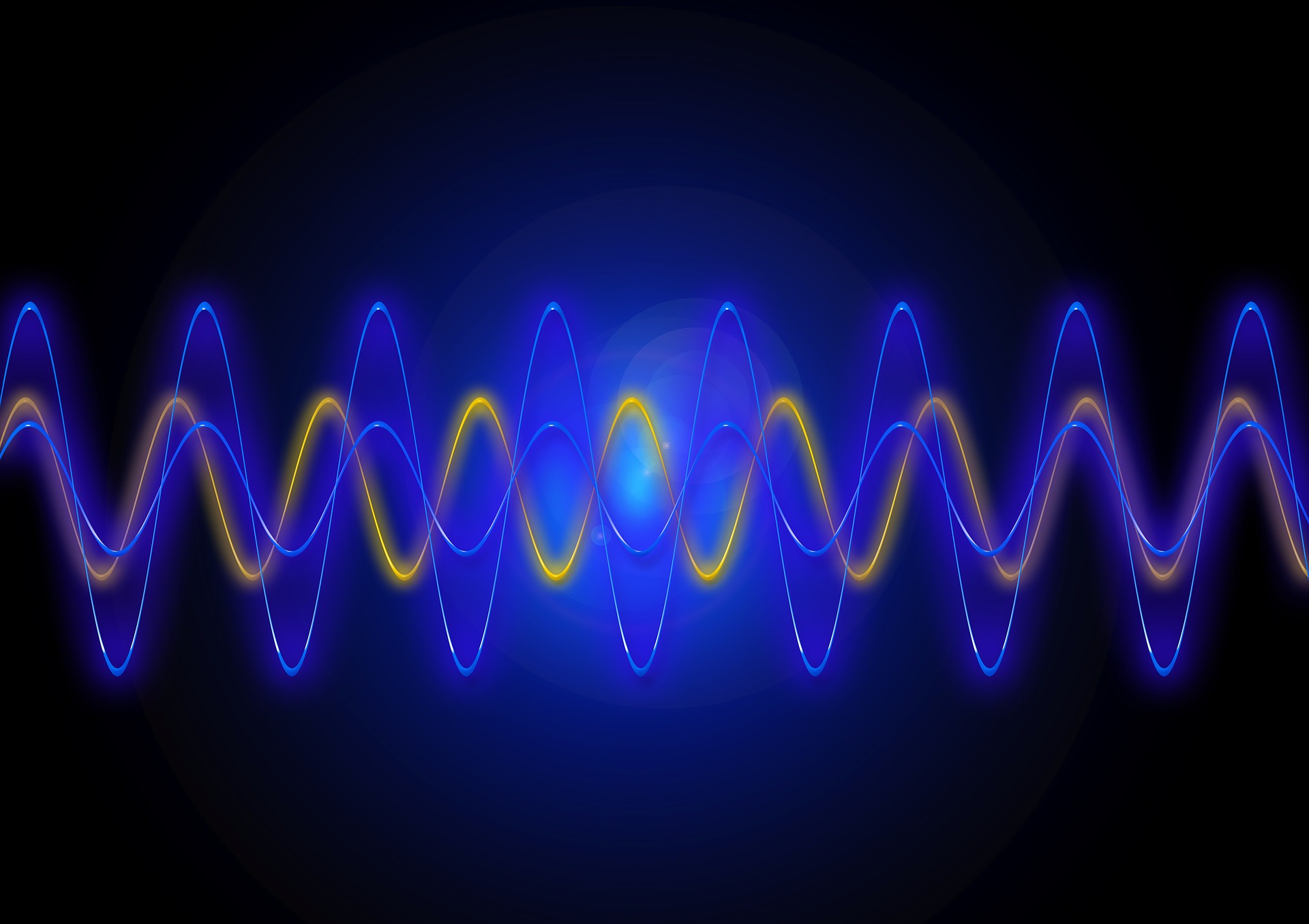
- Read more about MEASURING DISTORTION STRENGTH WITH DEWARPING DIFFUSION MODELS IN ANOMALY DETECTION
- Log in to post comments
Surface anomaly detection aims to localize abnormal regions in images. A representative approach is the reconstruction-based method, which detect defects via reconstruction errors using generative models trained on normal images. However, these methods cannot directly estimate local distortion levels, which are commonly used for products made of metal or resin. To address this issue, we propose DiffuDewarp, a novel method that directly estimates local distortions. Our approach defines a pseudo-deformation defect generation process as a new diffusion process based on localized warping.
- Categories:
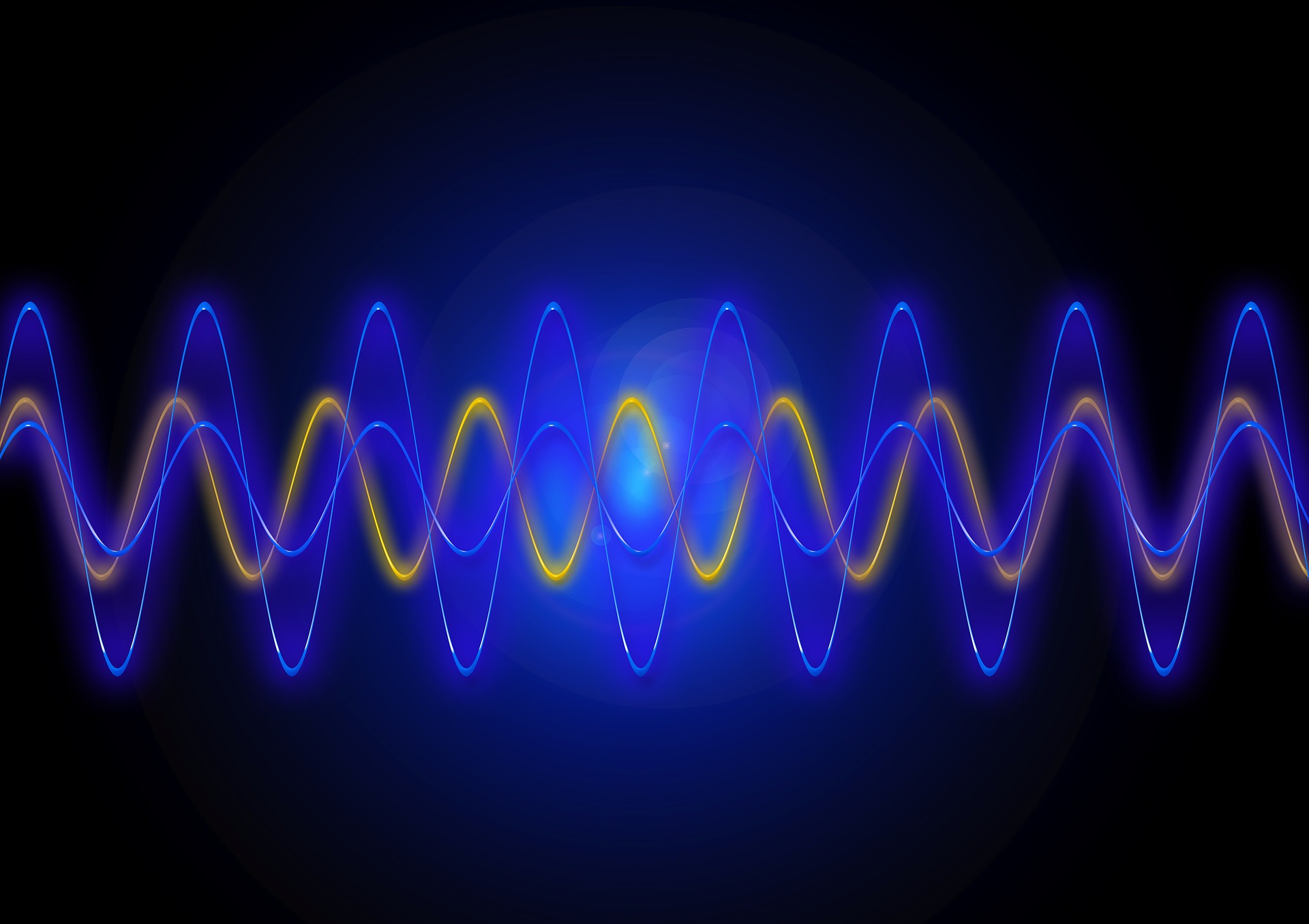
- Read more about Supplementary material
- Log in to post comments
Automatic facial stroke and palsy assessment systems based on computer vision have strong benefits in their application. We propose a framework that exploits facial graphs with temporal connections and analyses them through a graph attention-based model. The temporal facial graph captures structural and motion cues from the facial image sequence, while the graph attention mechanism effectively analyses the interrelation between facial regions in close proximity.
- Categories:
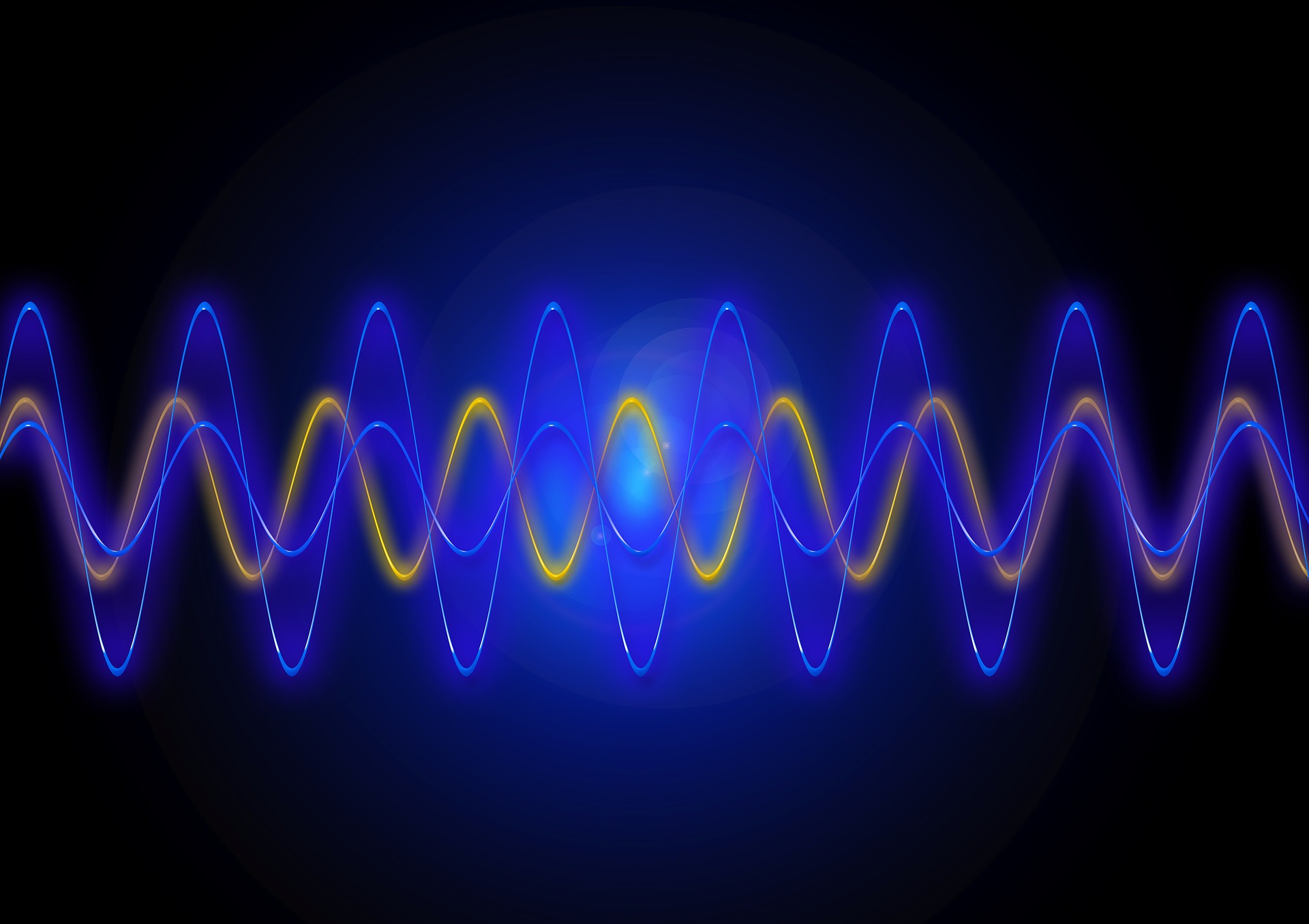
- Read more about Supplementary_Material
- Log in to post comments
In this paper, we propose a novel approach, HDNet-LE, designed to enhance low-light (LL) images in terms of contrast, noise removal, and other degradation kinds by leveraging the power of Wavelet transform (WT) and Fourier Transform (FT). HDNet-LE combines the strengths of Generative Adversarial Networks (GAN) with the multi-scale analysis capabilities of the Wavelet transform and the spatial frequency domain through the FT. Experimental results demonstrate the effectiveness of the proposed method in improving the visibility and quality of LL images.
- Categories:
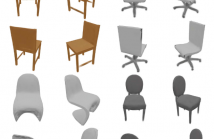
- Read more about DiGS-3D: Diffusion Transformer as unstructured 3D Gaussian Splatting Generator - Supplementary Material
- Log in to post comments
Generation of 3D Gaussian Splatting (3D GS) representation suffers from the challenge of maintaining the visual aspect while preserving the geometry of generated objects. We introduce DiGS-3D, an efficient method for generation of 3D Gaussian Splatting representations generation using a diffusion transformer. While current 3D GS generative models use proxy representation as a way to structure the point cloud before processing, our method preserves the original 3D structure of the 3D GS representation.
- Categories:
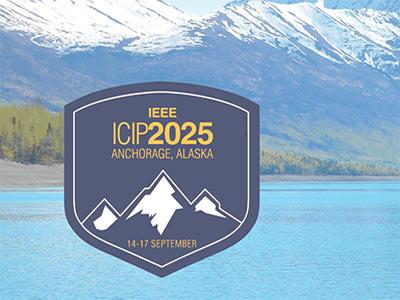
- Read more about HDNet-LE: A Hybrid Deep-Net Architecture for Low Light Image Enhancement
- Log in to post comments
In this paper, we propose a novel approach, HDNet-LE, designed to enhance low-light (LL) images in terms of contrast, noise removal, and other degradation kinds by leveraging the power of Wavelet transform (WT) and Fourier Transform (FT). HDNet-LE combines the strengths of Generative Adversarial Networks (GAN) with the multi-scale analysis capabilities of the Wavelet transform and the spatial frequency domain through the FT. Experimental results demonstrate the effectiveness of the proposed method in improving the visibility and quality of LL images.
- Categories: