Documents
Presentation Slides
An Accurate Evaluation of MSD Log-likelihood and its Application in Human Action Recognition
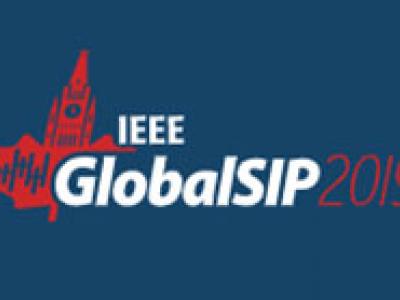
- Citation Author(s):
- Submitted by:
- Nuha Zamzami
- Last updated:
- 9 November 2019 - 7:08am
- Document Type:
- Presentation Slides
- Document Year:
- 2019
- Event:
- Presenters:
- Nizar Bouguila
- Paper Code:
- 1570561537
- Categories:
- Log in to post comments
In this paper, we examine the problem of modeling overdispersed frequency vectors that are naturally generated by several machine learning and computer vision applications.
We consider a statistical framework based on a mixture of Multinomial Scaled Dirichlet (MSD) distributions that we have previously proposed in [1]. Given that the likelihood function plays a key role in statistical inference, e.g. in maximum likelihood estimation and Fisher information matrix investigation, we propose to improve the efficiency of computing the MSD log-likelihood by approximating its function based on Bernoulli polynomials. As compared to [1], the log-likelihood function is computed using the proposed mesh algorithm and a model selection approach is seamlessly integrated with the parameters estimation. The improved clustering framework offers a good compromise between other techniques and improves the approach used before for the same model. The merits of the proposed approach are validated via a challenging application that involves human action recognition.