Documents
Presentation Slides
Acute Lymphoblastic Leukemia detection based on adaptive unsharpening and Deep Learning
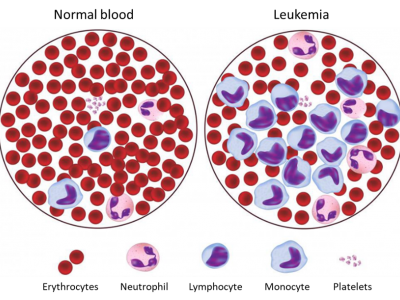
- Citation Author(s):
- Submitted by:
- Angelo Genovese
- Last updated:
- 22 June 2021 - 1:54am
- Document Type:
- Presentation Slides
- Document Year:
- 2021
- Event:
- Presenters:
- Angelo Genovese
- Paper Code:
- BIO-8.6
- Categories:
- Log in to post comments
Computer Aided Diagnosis (CAD) systems are increasingly utilizing image analysis and Deep Learning (DL) techniques, due to their high accuracy in several medical imaging fields, including the detection of Acute Lymphoblastic (or Lymphocytic) Leukemia (ALL) from peripheral blood samples. However, no method in the literature has specifically analyzed the focus quality of ALL images or proposed a technique for sharpening the samples in an adaptive way for the purpose of classification. To address this issue, in this paper we propose the first machine learning-based approach able to enhance blood sample images by an adaptive unsharpening method. The method uses image processing techniques and DL to normalize the radius of the cell, estimate the focus quality, adaptively improve the sharpness of the images, and then perform the classification. We evaluated the methodology on a public database of ALL images, considering several state-of-the-art CNNs to perform the classification, with results showing the validity of the proposed approach. For a complete reproducibility of the work, the source code is available at: http://iebil.di.unimi.it/cnnALL/index.htm.