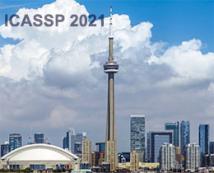
ICASSP 2021 - IEEE International Conference on Acoustics, Speech and Signal Processing is the world’s largest and most comprehensive technical conference focused on signal processing and its applications. The ICASSP 2021 conference will feature world-class presentations by internationally renowned speakers, cutting-edge session topics and provide a fantastic opportunity to network with like-minded professionals from around the world. Visit website.
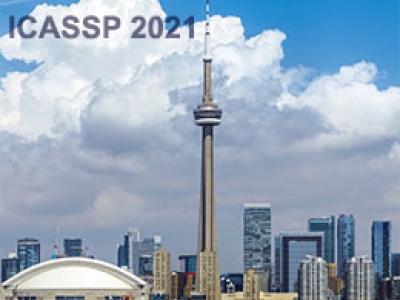
- Read more about [Poster] A Global Cayley Parametrization of Stiefel Manifold for Direct Utilization of Optimization Mechanisms Over Vector Spaces
- Log in to post comments
Optimization problem with orthogonality constraints, whose feasible region is called the Stiefel manifold, has rich applications in data sciences. The severe non-linearity of the Stiefel manifold has hindered the utilization of optimization mechanisms developed specially over a vector space for the problem. In this paper, we present a global parametrization of the Stiefel manifold entirely by a single fixed vector space with the Cayley transform, say Global Cayley Parametrization (G-CP), to solve the problem through optimization over a vector space.
- Categories:
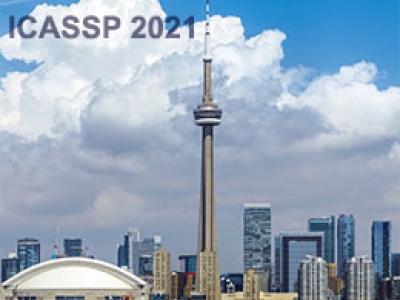
- Read more about Robust self-supervised speaker representation learning via instance mix regularization
- Log in to post comments
- Categories:
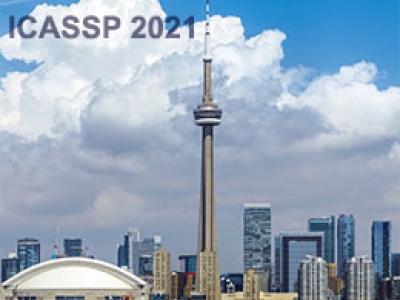
- Read more about COOPNET: MULTI-MODAL COOPERATIVE GENDER PREDICTION IN SOCIAL MEDIA USER PROFILING
- Log in to post comments
icassp poster.pdf

- Categories:
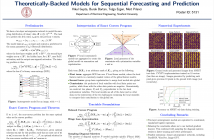
- Read more about Convex Neural Autoregressive Models: Towards Tractable, Expressive, and Theoretically-Backed Models for Sequential Forecasting and Prediction
- Log in to post comments
Three features are crucial for sequential forecasting and generation models: tractability, expressiveness, and theoretical backing. While neural autoregressive models are relatively tractable and offer powerful predictive and generative capabilities, they often have complex optimization landscapes, and their theoretical properties are not well understood. To address these issues, we present convex formulations of autoregressive models with one hidden layer.
- Categories:
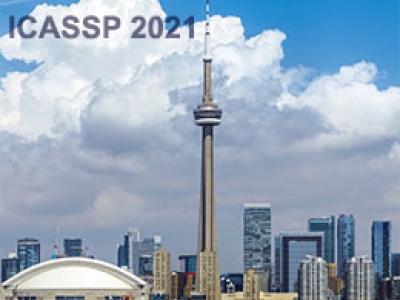
- Read more about SCALABLE REINFORCEMENT LEARNING FOR ROUTING IN AD-HOC NETWORKS BASED ON PHYSICAL-LAYER ATTRIBUTES
- Log in to post comments
This work proposes a novel and scalable reinforcement learning approach for routing in ad-hoc wireless networks. In most previous reinforcement learning based routing methods, the links in the network are assumed to be fixed, and a different agent is trained for
- Categories:
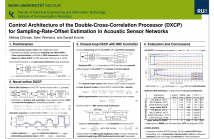
- Read more about CONTROL ARCHITECTURE OF THE DOUBLE-CROSS-CORRELATION PROCESSOR FOR SAMPLING-RATE-OFFSET ESTIMATION IN ACOUSTIC SENSOR NETWORKS
- Log in to post comments
Distributed hardware of acoustic sensor networks bears inconsistency of local sampling frequencies, which is detrimental to signal processing. Fundamentally, sampling rate offset (SRO) nonlinearly relates the discrete-time signals acquired by different sensor nodes. As such, retrieval of SRO from the available signals requires nonlinear estimation, like double-cross-correlation processing (DXCP), and frequently results in biased estimation. SRO compensation by asynchronous sampling rate conversion (ASRC) on the signals then leaves an unacceptable residual.
- Categories:
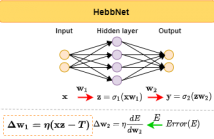
- Read more about HEBBNET: A SIMPLIFIED HEBBIAN LEARNING FRAMEWORK TO DO BIOLOGICALLY PLAUSIBLE LEARNING
- Log in to post comments
Backpropagation has revolutionized neural network training however, its biological plausibility remains questionable. Hebbian learning, a completely unsupervised and feedback free learning technique is a strong contender for a biologically plausible alternative. However, so far, it has neither achieved high accuracy performance vs. backprop, nor is the training procedure simple. In this work, we introduce a new Hebbian learning based neural network, called Hebb-Net.
Video_slides.pdf

- Categories:
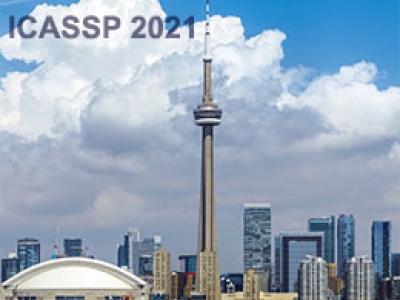
- Read more about MODIFIED ARCSINE LAW FOR ONE-BIT SAMPLED STATIONARY SIGNALS WITH TIME-VARYING THRESHOLDS
- Log in to post comments
One-bit quantization has attracted considerable attention in signal processing for communications and sensing. The arcsine law is a useful relation often used to estimate the normalized covariance matrix of zero-mean stationary input signals when they are sampled by one-bit analog-to-digital converters (ADCs)—practically comparing the signals with a given threshold level. This relation, however, only considers a zero threshold which can cause a remarkable information loss.
- Categories:
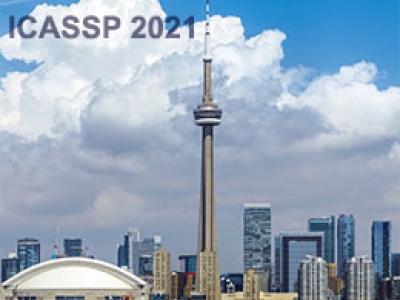
- Read more about Optimizing Short-Time Fourier Transform Parameters via Gradient Descent
- Log in to post comments
- Categories:
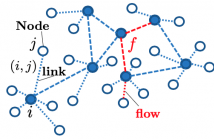
- Read more about Distributed Scheduling Using Graph Neural Networks
- Log in to post comments
A fundamental problem in the design of wireless networks is to efficiently schedule transmission in a distributed manner. The main challenge stems from the fact that optimal link scheduling involves solving a maximum weighted independent set (MWIS) problem, which is NP-hard. For practical link scheduling schemes, distributed greedy approaches are commonly used to approximate the solution of the MWIS problem. However, these greedy schemes mostly ignore important topological information of the wireless networks.
Zhao_ICASSP2021.pdf

- Categories: