Documents
Poster
Adversarial variational Bayes methods for Tweedie compound Poisson mixed models
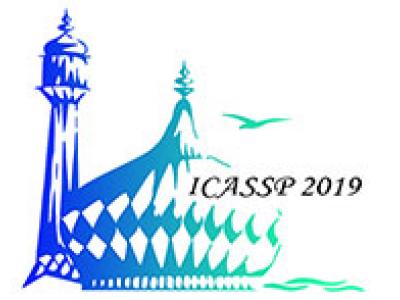
- Citation Author(s):
- Submitted by:
- Yuanyuan Liu
- Last updated:
- 10 May 2019 - 1:24pm
- Document Type:
- Poster
- Document Year:
- 2019
- Event:
- Presenters:
- Yuanyuan Liu
- Paper Code:
- 3968
- Categories:
- Log in to post comments
The Tweedie Compound Poisson-Gamma model is routinely used for modeling non-negative continuous data with a discrete probability mass at zero. Mixed models with random effects account for the covariance structure related to the grouping hierarchy in the data. An important application of Tweedie mixed models is pricing the insurance policies, e.g. car insurance. However, the intractable likelihood function, the unknown variance function, and the hierarchical structure of mixed effects have presented considerable challenges for drawing inferences on Tweedie. In this study, we tackle the Bayesian Tweedie mixed-effects models via variational inference approaches. In particular, we empower the posterior approximation by implicit models trained in an adversarial setting. To reduce the variance of gradients, we reparameterize random effects, and integrate out one local latent variable of Tweedie. We also employ a flexible hyper prior to ensure the richness of the approximation. Our method is evaluated on both simulated and real-world data. Results show that the proposed method has smaller estimation bias on the random effects compared to traditional inference methods including MCMC; it also achieves a state-of-the-art predictive performance, meanwhile offering a richer estimation of the variance function.
poster.pdf
