Documents
Poster
An algorithm for multi subject fMRI analysis based on the SVD and penalized rank-1 matrix approximation
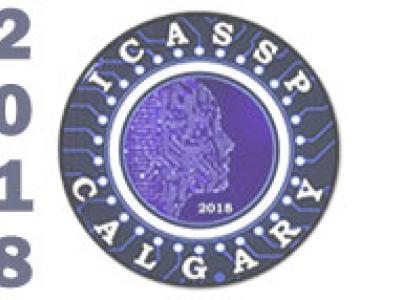
- Citation Author(s):
- Submitted by:
- Asif Iqbal
- Last updated:
- 14 April 2018 - 9:28pm
- Document Type:
- Poster
- Document Year:
- 2018
- Event:
- Presenters:
- Abd-Krim Seghouane
- Paper Code:
- 1970
- Categories:
- Keywords:
- Log in to post comments
In recent years, data driven methods have been successfully used for analyzing multi-subject functional magnetic resonance imaging (fMRI) datasets. These methods attempt to learn shared spatial activation maps (SM) or voxel time courses (TC) from temporally or spatially concatenated fMRI datasets respectively. Most of the methods proposed so far do not distinguish whether a particular SM/TC is a group level component or only present in a certain subject dataset. In this paper we present a new two stage algorithm which aims to separate the joint and sub-specific information from the temporally concatenated multi-subject datasets. The proposed method is based on the singular value decomposition (SVD) and penalized rank-one matrix approximation. Simulation experiments are used to demonstrate this ability of the proposed algorithm followed by validation on real experimental task-fMRI datasets.