Documents
Presentation Slides
Alternating Constrained Minimization based Approximate Message Passing
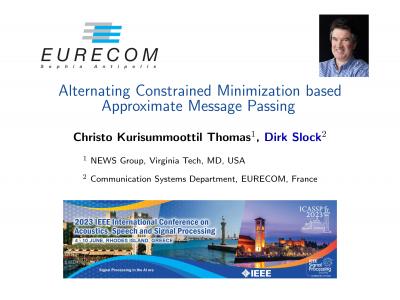
- Citation Author(s):
- Submitted by:
- Dirk Slock
- Last updated:
- 30 May 2023 - 5:37pm
- Document Type:
- Presentation Slides
- Document Year:
- 2023
- Event:
- Presenters:
- Dirk Slock
- Paper Code:
- SS-L24.5
- Categories:
- Log in to post comments
Generalized Approximate Message Passing (GAMP) allows for Bayesian inference in linear models with non-identically independently distributed (n.i.i.d.) priors and n.i.i.d. measurements of the linear mixture outputs. It represents an efficient technique for approximate inference, which becomes accurate when both rows and columns of the measurement matrix can be treated as sets of independent vectors and both dimensions become large. It has been shown that the fixed points of GAMP correspond to the extrema of a large system limit of the Bethe Free Energy (LSL-BFE), which represents a meaningful approximation optimization criterion regardless of whether the measurement matrix exhibits the independence properties. However, the convergence of (G)AMP can be notoriously problematic for certain measurement matrices, and the only sure fixes so far are damping (by a difficult-to-determine amount) or perform a double ADMM. In this paper, we revisit the GAMP algorithm (as e.g. for sparse Bayesian learning (SBL)) by more rigorously applying an alternating constrained minimization strategy to an appropriately reparameterized LSL BFE. This guarantees convergence, at least to a local optimum. We furthermore introduce a natural extension of the BFE to integrate the estimation of (the SBL)
hyperparameters via Variational Bayes, leading to Variational AMBGAMP or VAMBGAMP.