Documents
Presentation Slides
Approaching Optimal Embedding in Audio Steganography with GAN
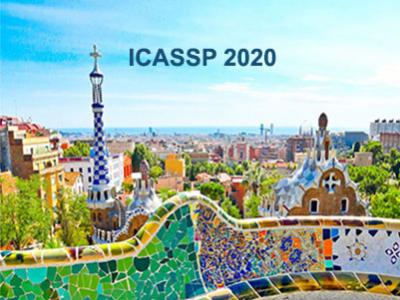
- Citation Author(s):
- Submitted by:
- Huilin Zheng
- Last updated:
- 28 May 2020 - 10:39pm
- Document Type:
- Presentation Slides
- Document Year:
- 2020
- Event:
- Presenters:
- Huilin Zheng
- Paper Code:
- 2093
- Categories:
- Log in to post comments
Audio steganography is a technology that embeds messages into audio without raising any suspicion from hearing it. Current steganography methods are based on heuristic cost designs. In this work, we proposed a framework based on Generative Adversarial Network (GAN) to approach optimal embedding for audio steganography in the temporal domain. This is the first attempt to approach optimal embedding with GAN and automatically learn the embedding probability/cost for audio steganography. The embedding framework consists of three parts: a U-Net based generator, an embedding simulator, and a discriminator. For practical applications, Syndrome-Trellis Coding (STC) is used to generate stego audio with the learned embedding probability. Experimental results on the UME-ERJ and WSJ speech datasets have shown that the proposed framework can automatically learn the adaptive embedding probabilities for audio steganogra- phy and has a considerable advantage in terms of resisting steganalyzers in comparison with the existing conventional method.