Documents
Supplementary material
ATAC-NET: ZOOMED VIEW WORKS BETTER FOR ANOMALY DETECTION
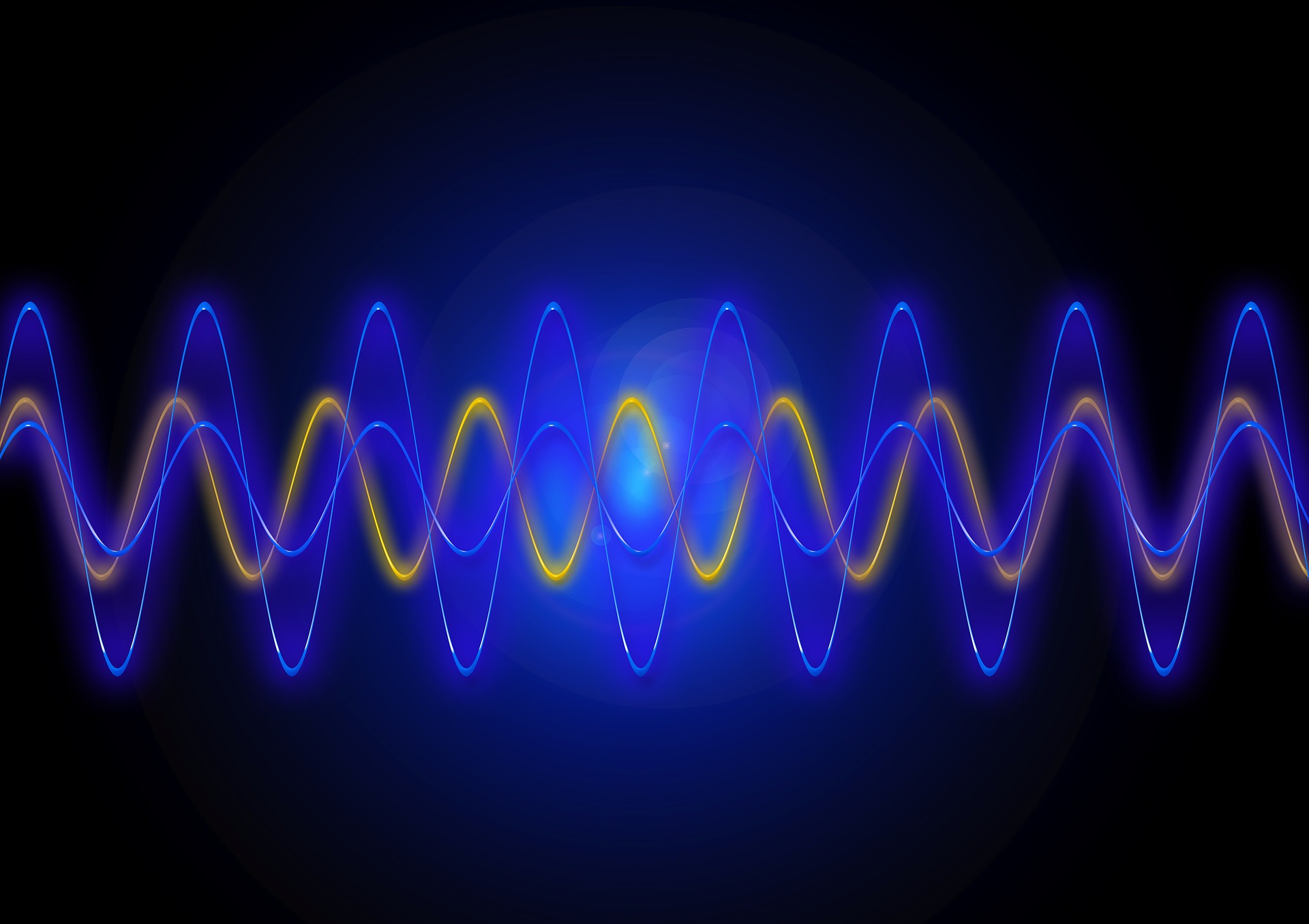
- Citation Author(s):
- Submitted by:
- Shaurya Gupta
- Last updated:
- 6 February 2024 - 2:52pm
- Document Type:
- Supplementary material
- Document Year:
- 2024
- Presenters:
- Shaurya Gupta
- Paper Code:
- 2078
- Categories:
- Log in to post comments
Supplementary material for the paper: ATAC-NET: ZOOMED VIEW WORKS BETTER FOR ANOMALY DETECTION
Abstract: The application of deep learning in visual anomaly detection has gained widespread popularity due to its potential use in quality control and manufacturing. Current standard methods are Unsupervised, where a clean dataset is utilised to detect deviations and flag anomalies during testing. However, incorporating a few samples when the type of anomalies is known beforehand can significantly enhance performance. Thus, we propose ATAC-Net, a framework that trains to detect anomalies from a minimal set of known prior anomalies. Furthermore, we introduce attention-guided cropping, which provides a closer view of suspect regions during the training phase. Our framework is a reliable and easy-to-understand system for detecting anomalies, and we substantiate its superiority to some of the current state-of-the-art techniques in a comparable setting.
Comments
ATAC-Net Paper Supplementary Material
Supplementary material for the paper containing ablation study and training experiments. Please open the above link to view the same