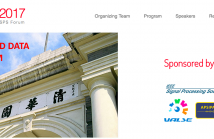
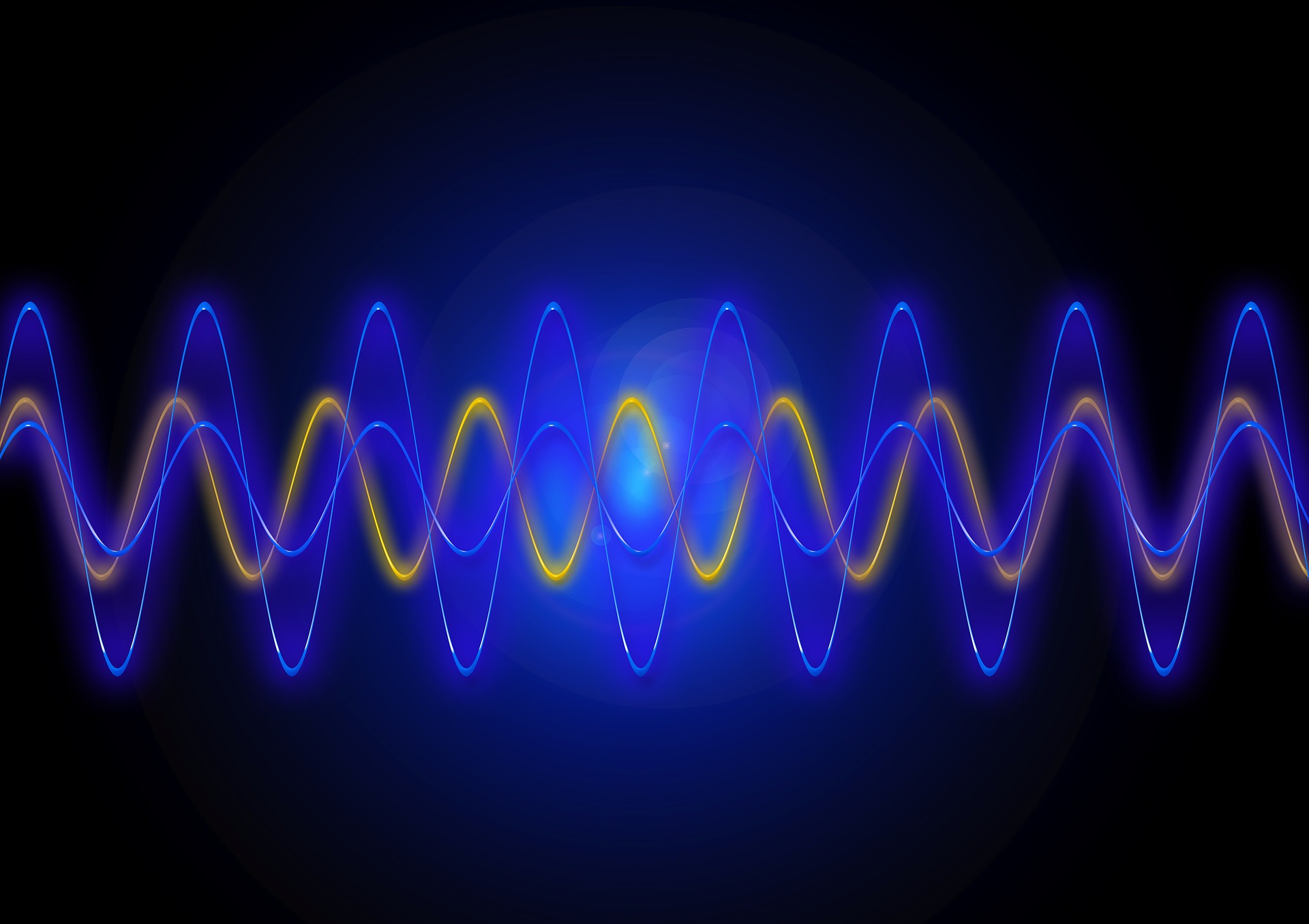
- Read more about GUIDED ADVERSARIAL WATERMARKS FOR PHYSICAL ATTACKS ON FACE RECOGNITION
- Log in to post comments
Supplementary Materials
- Categories:
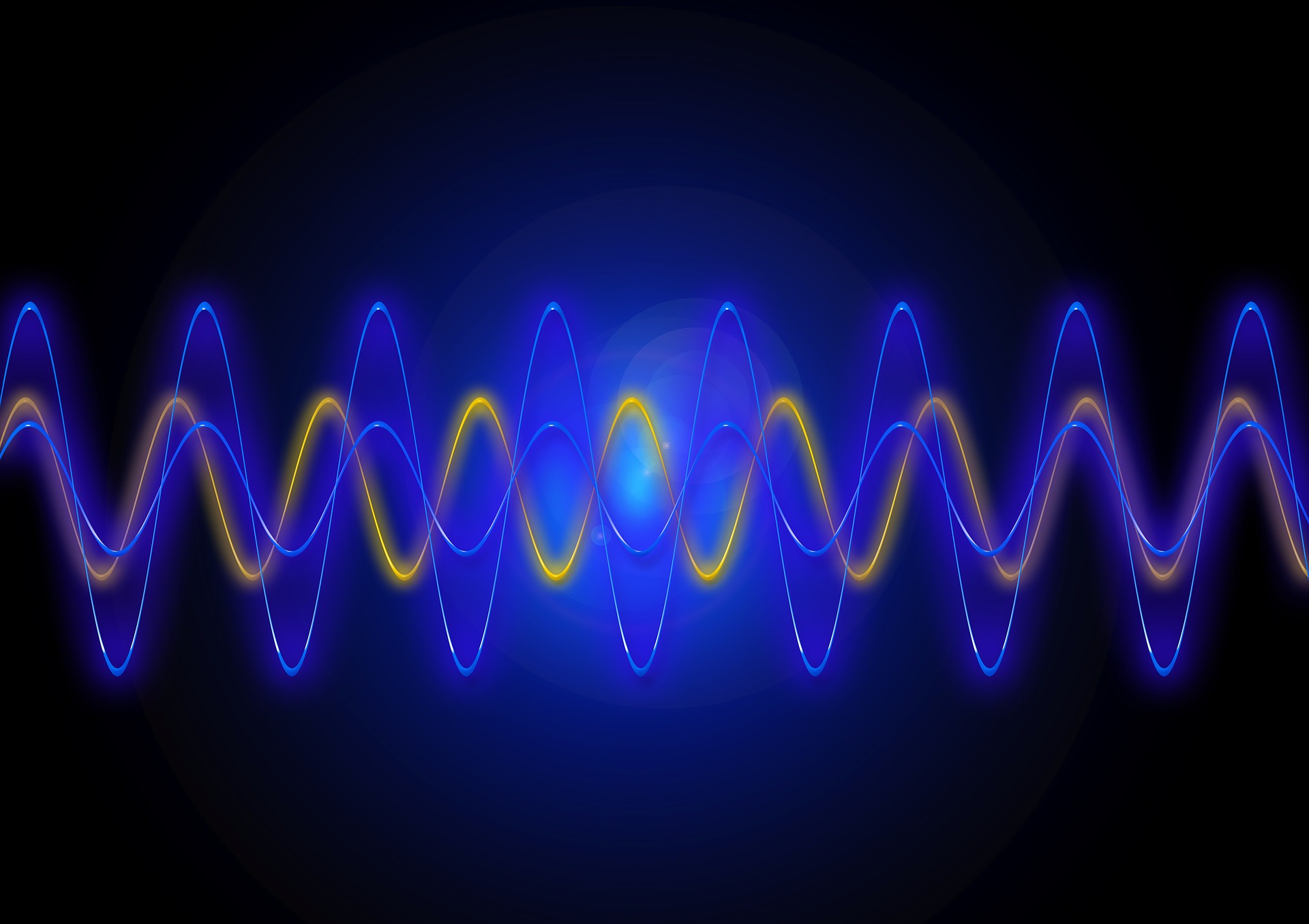
- Read more about ATAC-NET: ZOOMED VIEW WORKS BETTER FOR ANOMALY DETECTION
- 1 comment
- Log in to post comments
Supplementary material for the paper: ATAC-NET: ZOOMED VIEW WORKS BETTER FOR ANOMALY DETECTION
- Categories:
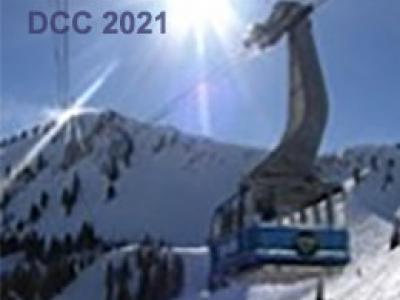
- Read more about Regularized Semi-Nonnegative Matrix Factorization Using L21-Norm for Data Compression
- Log in to post comments
Data reduction algorithms, including matrix factorization techniques, represent an essential component of many ML systems.One popular paradigm of matrix factorizations includes Non-Negative Matrix Factorization (NMF).
- Categories:
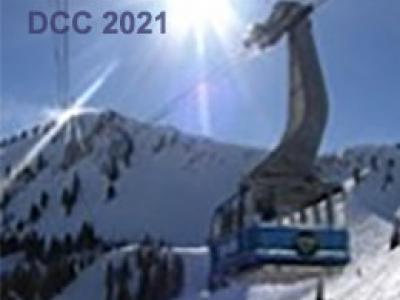
- Categories:
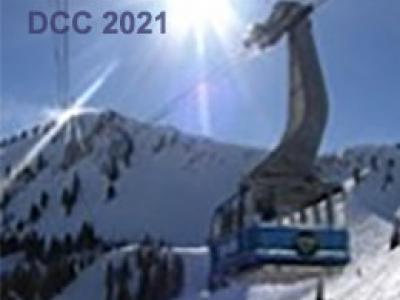
- Read more about Video Enhancement Network Based on Max-pooling and Hierarchical Feature Fusion
- Log in to post comments
In this paper, we propose an efficient convolution neural network to enhance the quality of video compressed by HEVC standard. The model is composed of a max-pooling module and a hierarchical feature fusion module. The max-pooling module extracts feature from different scales and enlarges the receptive field of the model without stacking too many convolution layers. And the hierarchical feature fusion module accurately aligns features from different scales and fuses them efficiently.
DCCPPT.pdf

- Categories:
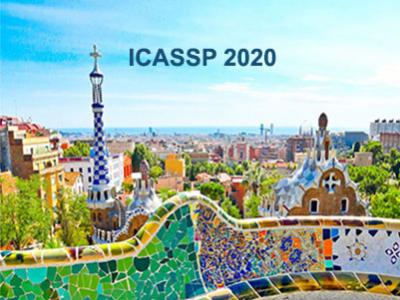
- Read more about Adversarial Networks for Secure Wireless Communications - Slides
- Log in to post comments
We propose a data-driven secure wireless communication scheme, in which the goal is to transmit a signal to a legitimate receiver with minimal distortion, while keeping some information about the signal private from an eavesdropping adversary. When the data distribution is known, the optimal trade-off between the reconstruction quality at the legitimate receiver and the leakage to the adversary can be characterised in the information theoretic asymptotic limit.
- Categories:
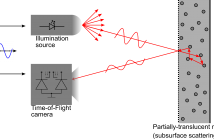
- Read more about A Single-Wavelength Real-Time Material-Sensing Camera Based on Time-of-Flight Measurements
- Log in to post comments
Time-of-Flight (ToF) cameras provide a fast and robust way of acquiring the 3D shape of real scenes. Dense depth images can be generated at tens of frame per second. 3D shapes can be then segmented and objects classified, but can we directly sense the objects’ material using just a ToF camera? This live demonstration proves the answer to be affirmative. This possibility has only very recently been unveiled and we are, to the best of our knowledge, the first providing a live demonstrator showing the feasibility of this approach.
- Categories:
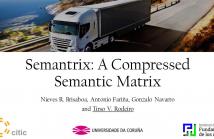
- Read more about Semantrix: A Compressed Semantic Matrix
- 3 comments
- Log in to post comments
We present a compact data structure to represent both the duration and length of homogeneous segments of trajectories from moving objects in a way that, as a data warehouse, it allows us to efficiently answer cumulative queries. The division of trajectories into relevant segments has been studied in the literature under the topic of Trajectory Segmentation. In this paper, we design a data structure to compactly represent them and the algorithms to answer the more relevant queries.
- Categories: