Documents
Presentation Slides
Attention Boosted Deep Networks for Video Classficaition
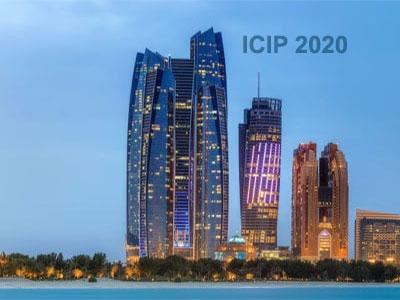
- Citation Author(s):
- Submitted by:
- Junyong You
- Last updated:
- 2 November 2020 - 10:39am
- Document Type:
- Presentation Slides
- Document Year:
- 2020
- Event:
- Presenters:
- Junyong You
- Paper Code:
- https://github.com/junyongyou/Attention-boosted-deep-networks-for-video-classification
- Categories:
- Log in to post comments
Video classification can be performed by summarizing image contents of individual frames into one class by deep neural networks, e.g., CNN and LSTM. Human interpretation of video content is influenced by the attention mechanism. In other words, video class can be more attentively decided by certain information than others. In this paper, we propose to integrate the attention mechanism into deep networks for video classification. The proposed framework employs 2D CNN networks with ImageNet pretrained weights to extract features of video frames that are then fed to a bidirectional LSTM network for video classification. An attention block has been developed that can be added after the LSTM network in the proposed framework. Several different 2D CNN architectures have been tested in the experiments. The results with respect to two publicly available datasets have demonstrated that integrating attention can boost the performance of deep networks in video classification compared to not applying the attention block. We also found out that applying attention to the LSTM outputs on the VGG19 architecture provides the highest classification accuracy in the proposed framework.