Documents
Poster
Automatic neural network search method for Open Set Recognition
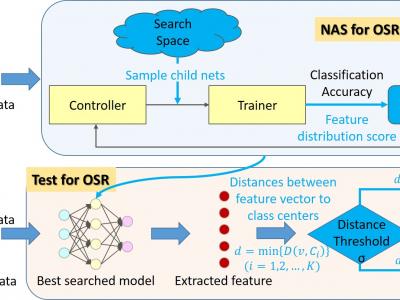
- Citation Author(s):
- Submitted by:
- Li Sun
- Last updated:
- 17 September 2019 - 4:35am
- Document Type:
- Poster
- Document Year:
- 2019
- Event:
- Presenters:
- Li Sun
- Paper Code:
- 3142
- Categories:
- Keywords:
- Log in to post comments
Real-world recognition or classification tasks in computer vision are not apparent in controlled environments and often get involved in open set. Previous research work on real-world recognition problem is knowledge- and labor-intensive to pursue good performance for there are numbers of task domains. Auto Machine Learning (AutoML) approaches supply an easier way to apply advanced machine learning technologies, reduce the demand for experienced human experts and improve classification performance on close set. This paper proposes an automatic neural network search method for designing effective convolution neural network (CNN) models for open set recognition (OSR). Feature distribution information is explicitly incorporated into the main objective. So during the search process, the sampled models will enlarge inter-class differences and reduce intra-class variations. We design a flexible search space based on classic CNN models to diversify neural architectures and also add some search principles to limit the size of the search space. Experimental results on CIFAR-10 and Dunhuang historical Chinese datasets show that our approach improves performances on both close and open set. Comparing with the other two OSR algorithms, our method also achieves the best performance.