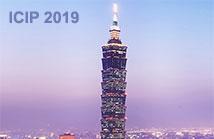
The International Conference on Image Processing (ICIP), sponsored by the IEEE Signal Processing Society, is the premier forum for the presentation of technological advances and research results in the fields of theoretical, experimental, and applied image and video processing. ICIP has been held annually since 1994, brings together leading engineers and scientists in image and video processing from around the world. Visit website.
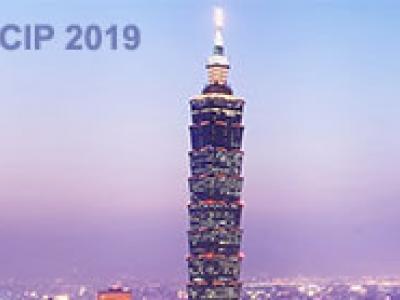
- Read more about Learning Lightweight Pedestrian Detector with Hierarchical Knowledge Distillation
- Log in to post comments
- Categories:
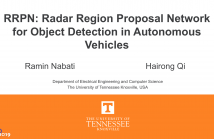
- Read more about RRPN: Radar Region Proposal Network for Object Detection in Autonomous Vehicles
- Log in to post comments
- Categories:
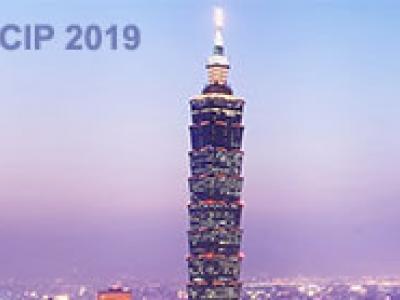
- Read more about When Spatially-Variant Filtering Meets Low-Rank Regularization: Exploiting Non-Local Similarity for Single Image Interpolation
- Log in to post comments
This paper combines spatially-variant filtering and non-local low-rank regularization (NLR) to exploit non-local similarity in natural images in addressing the problem of image interpolation. We propose to build a carefully designed spatially-variant, non-local filtering scheme to generate a reliable estimate of the interpolated image and utilize NLR to refine the estimation. Our work uses a simple, parallelizable algorithm without the need to solve complicated optimization problems.
- Categories:
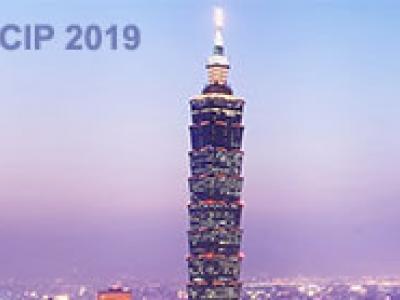
- Read more about LEVERAGING THE DISCRETE COSINE BASIS FOR BETTER MOTION MODELLING IN HIGHLY TEXTURED VIDEO SEQUENCES
- Log in to post comments
Motion modelling plays a central role in video compression. This role is even more critical in highly textured video sequences, whereby a small error can produce large residuals that are costly to compress. While the translational motion model employed by existing coding standards, such as HEVC, is sufficient in most cases, using higher order models is beneficial; for this reason, the upcoming video coding standard, VVC, employs a 4-parameter affine model.
- Categories:
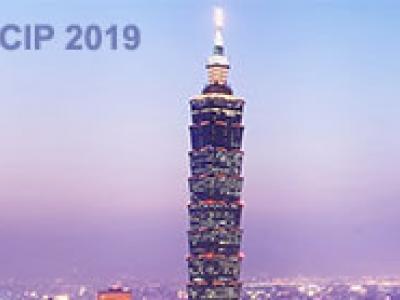
- Read more about TOWARDS MODELLING OF VISUAL SALIENCY IN POINT CLOUDS FOR IMMERSIVE APPLICATIONS
- Log in to post comments
Modelling human visual attention is of great importance in the field of computer vision and has been widely explored for 3D imaging. Yet, in the absence of ground truth data, it is unclear whether such predictions are in alignment with the actual human viewing behavior in virtual reality environments. In this study, we work towards solving this problem by conducting an eye-tracking experiment in an immersive 3D scene that offers 6 degrees of freedom. A wide range of static point cloud models is inspected by human subjects, while their gaze is captured in real-time.
- Categories:
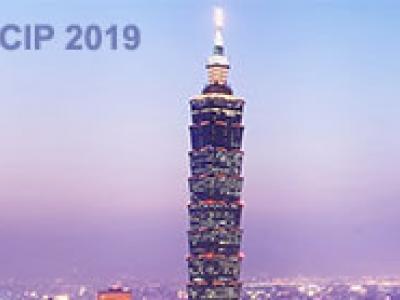
- Read more about STEADIFACE: REAL-TIME FACE-CENTRIC STABILIZATION ON MOBILE PHONES
- Log in to post comments
We present Steadiface, a new real-time face-centric video stabilization method that simultaneously removes hand shake and keeps subject's head stable. We use a CNN to estimate the face landmarks and use them to optimize a stabilized head center. We then formulate an optimization problem to find a virtual camera pose that locates the face to the stabilized head center while retains smooth rotation and translation transitions across frames. We test the proposed method on fieldtest videos and show it stabilizes both the head motion and background.
- Categories:
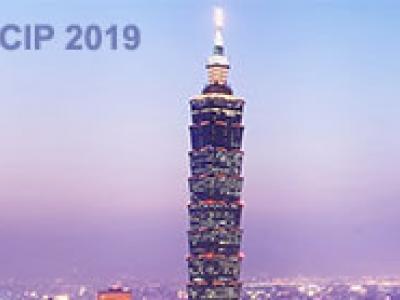
- Read more about VARIATIONAL REGULARIZED TRANSMISSION REFINEMENT FOR IMAGE DEHAZING
- Log in to post comments
High-quality dehazing performance is highly dependent upon the accurate estimation of transmission map. In this work, the coarse estimation version is first obtained by weightedly fusing two different transmission maps, which are generated from foreground and sky regions, respectively. A hybrid variational model with promoted regularization terms is then proposed to assisting in refining transmission map. The resulting complicated optimization problem is effectively solved via an alternating direction algorithm.
- Categories:
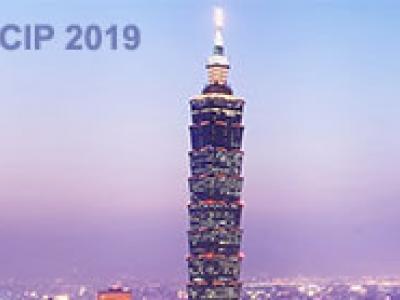
- Read more about Saliency Driven Perceptual Quality Metric for Omnidirectional Visual Content - Slides
- Log in to post comments
The problem of objectively measuring perceptual quality of omnidirectional visual content arises in many immersive imaging applications and particularly in compression. The interactive nature of this type of content limits the performance of earlier methods designed for static images or for video with a predefined dynamic. The non-deterministic impact must be addressed using statistical approach. One of the ways to describe, analyze and predict viewer interactions in omnidirectional imaging is through estimation of visual attention.
- Categories:
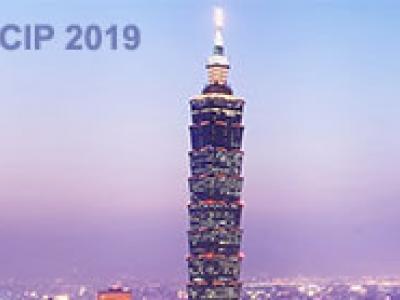
- Read more about Dataset Culling: Towards Efficient Training of Distillation-based Domain Specific Models
- Log in to post comments
- Categories:
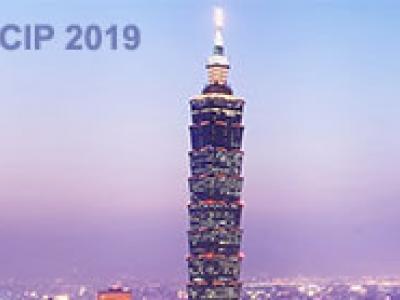
- Read more about Sinogram Image Completion for Limited Angle Tomography with Generative Adversarial Networks
- Log in to post comments
- Categories: