Documents
Poster
BOOSTING ZERO-SHOT NODE CLASSIFICATION VIA DEPENDENCY CAPTURE AND DISCRIMINATIVE FEATURE LEARNING
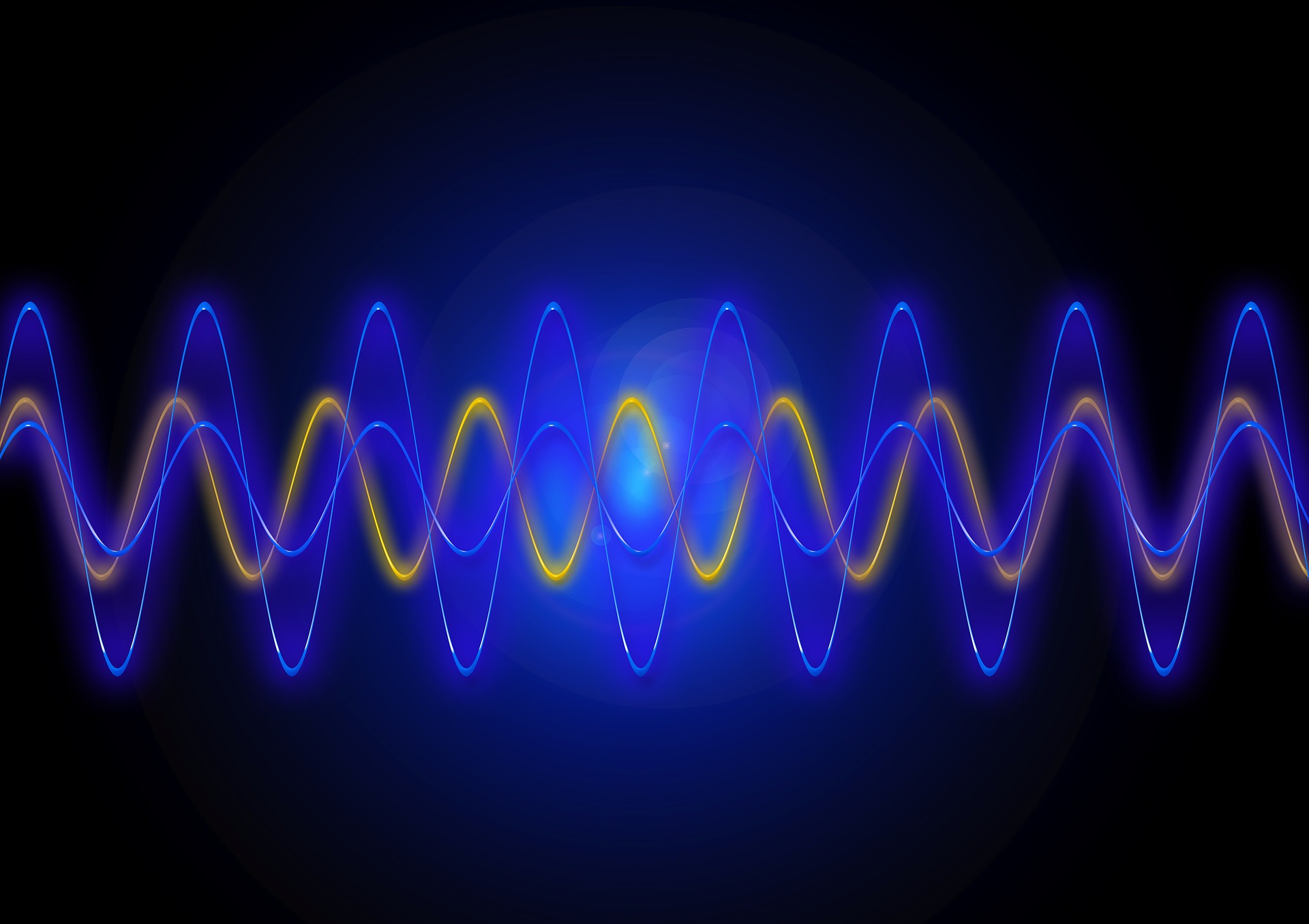
- DOI:
- 10.60864/ccha-4n66
- Citation Author(s):
- Submitted by:
- Zhiliang Hao
- Last updated:
- 6 June 2024 - 10:50am
- Document Type:
- Poster
- Categories:
- Keywords:
- Log in to post comments
Zero-shot node classification aims to predict nodes belonging to novel classes that have not been seen in the training. Existing studies focus on transferring knowledge from seen classes to unseen classes, which have achieved good performance in most cases. However, they do not fully leverage the relationships between nodes and overlook the issue of domain bias, affecting overall performance. In this paper, we propose a novel dependency capture and discriminative feature learning (DCDFL) model for zero-shot node classification. First, we address the issue of underutilized relationships between nodes by designing a relation-aware network to capture potentially long-range dependency, which can effectively utilize the available data information. Next, we introduce a domain-invariant adversarial loss to mitigate domain bias, encouraging the model to learn domain-insensitive feature representations. Furthermore, we refine and adapt the representation by leveraging inter-class separability within the metric space. Finally, extensive experiments on three well-known benchmark datasets demonstrate the superiority of our method over the strong baselines.