Documents
Presentation Slides
CENET: CONTENT-AWARE ENHANCED NETWORK FOR PRACTICAL SCENE PARSING
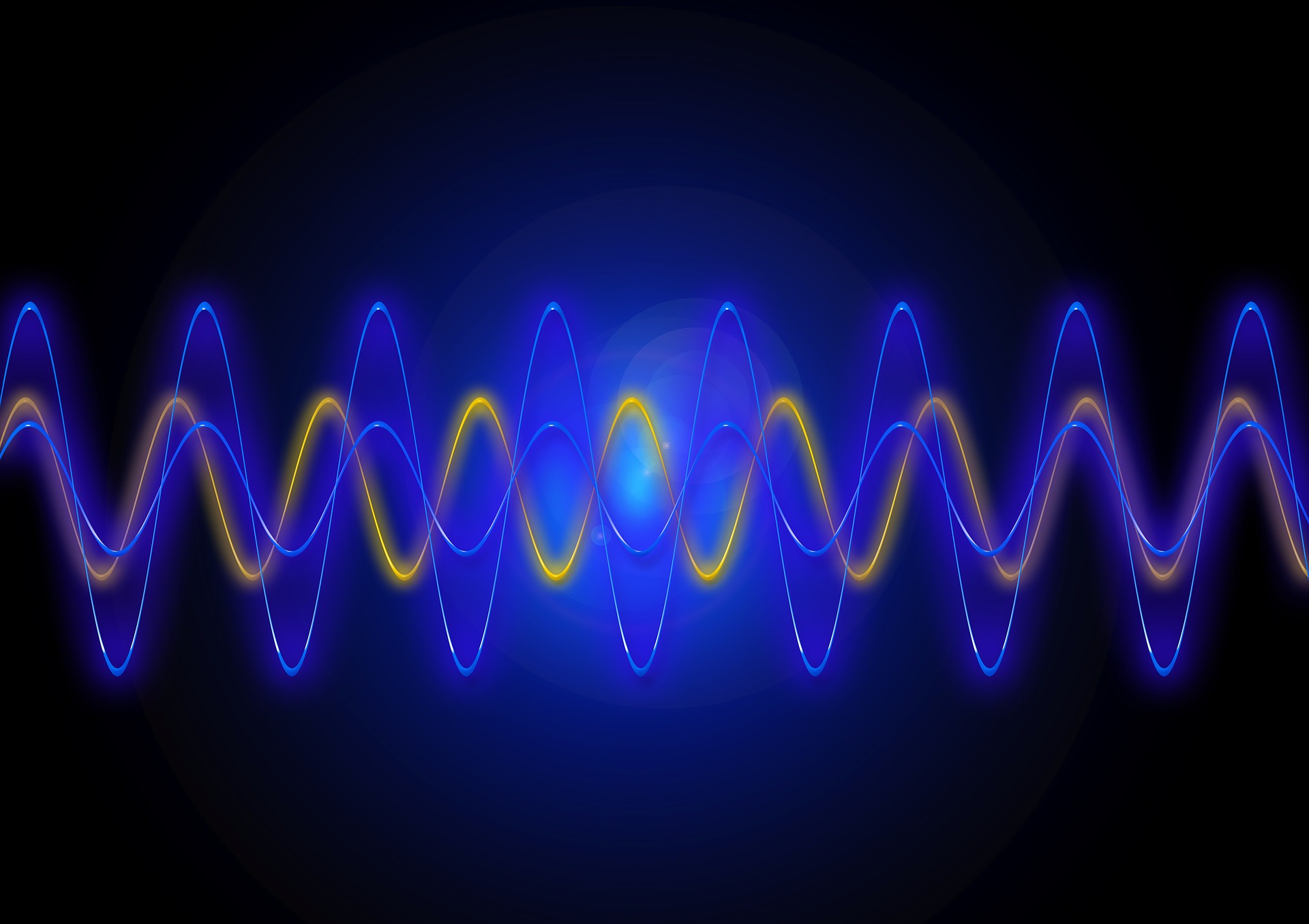
- DOI:
- 10.60864/899e-f739
- Citation Author(s):
- Submitted by:
- Yuan Zheng
- Last updated:
- 6 June 2024 - 10:24am
- Document Type:
- Presentation Slides
- Categories:
- Log in to post comments
Attention mechanisms are widely adopted in existing scene parsing methods due to their excellent performance, especially spatial self-attention. However, spatial self-attention suffers from high computational complexity, which limits the practical applications of the scene parsing methods on mobile devices with limited resources. In view of this, we propose a simple yet effective spatial attention module, namely Content-Aware Attention Module (CAAM). CAAM is a lightweight spatial attention module that consists of several convolution and pooling operations, compared to various spatial self-attention modules. Moreover, it is able to adaptively select spatial pixel information which is helpful for scene parsing task. With CAAM, we present a Content-aware Enhanced Network for scene parsing (CENet), where CAAM is introduced into the lateral connections at four different scales, resulting in a semantic alignment at adjacent scales and an effective semantic propagation. To validate the performance of the proposed CAAM and CENet, we conduct extensive experiments and achieve consistently improved performances on three popular benchmarks. Furthermore, we verify their generalization ability when using different baseline models and backbone networks. Code is available at https://github.com/ZY-IMU-CV/CENET_SK_2023.