Documents
Poster
CLASSIFIER CASCADE TO AID IN DETECTION OF EPILEPTIFORM TRANSIENTS IN INTERICTAL EEG
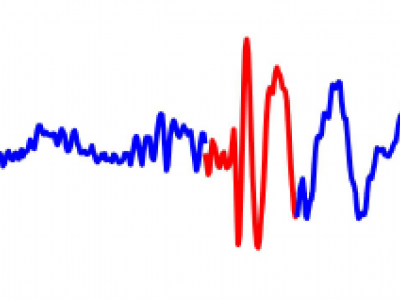
- Citation Author(s):
- Submitted by:
- Elham Bagheri
- Last updated:
- 13 April 2018 - 5:07pm
- Document Type:
- Poster
- Document Year:
- 2018
- Event:
- Presenters:
- Justin Dauwels
- Paper Code:
- 3275
- Categories:
- Log in to post comments
Presence of interictal epileptiform discharges (IED) in the electroencephalogram (EEG) is indicative of epilepsy. Automated
software for annotating EEGs of Patients with suspected epilepsy is substantial for diagnosis and management of epilepsy.
A large amount of data is needed for training and evaluating the performance of an effective IED detection system. IEDs occur
infrequently in the EEG of most patients, hence, interictal EEG recordings contain mostly background waveforms. As the first
step to detect IEDs automatically, we propose to eliminate most EEG background data using simple fast classifiers. This could
help us save computation time for an IED detection, and the remaining waveforms can be classified by more computationally
intensive methods.
We designed a cascade of thresholding steps, and employed it to eliminate background data in interictal EEG, while preserving
most IEDs. Several quick EEG features were considered. We rejected background waveforms by applying thresholds on these
features in consecutive steps.
We applied the propose algorithm on a dataset consisting of 156 subjects. We were able to eliminate 78.41% of background
waveforms while retaining 96.93% of IEDs on our cross-validated dataset. Furthermore, we applied classifiers to detect IEDs with
and without initial background rejection. The results show that rejecting background by our proposed method, speeds up the
classification by a factor ranging from 4.69 to 1.76 for the considered classifiers.