Documents
Presentation Slides
Collaborative Learning of Semi-Supervised Clustering and Classification for Labeling Uncurated Data
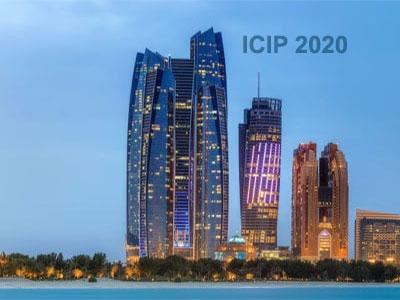
- Citation Author(s):
- Submitted by:
- Sara Mousavi
- Last updated:
- 2 November 2020 - 1:10pm
- Document Type:
- Presentation Slides
- Document Year:
- 2020
- Event:
- Presenters:
- Sara Mousavi
- Paper Code:
- ARS-12
- Categories:
- Keywords:
- Log in to post comments
Domain-specific image collections present potential value in various areas of science and business but are often not curated nor have any way to readily extract relevant content. To employ contemporary supervised image analysis methods on such image data, they must first be cleaned and organized, and then manually labeled for the nomenclature employed in the specific domain, which is a time consuming and expensive endeavor.
To address this issue, we designed and implemented the Plud system.
Plud provides an iterative semi-supervised workflow to minimize the effort spent by an expert and, due to the fact that it does not make any assumption about its input, can handle realistic large collections of images regardless of their size and type.
Plud is an iterative sequence of unsupervised clustering, human assistance, and supervised classification. With each iteration 1) the labeled dataset grows, 2) the generality of the classification method and its accuracy increases, and 3) manual effort is reduced.
We evaluated the effectiveness of our system, by applying it on over a million images documenting human decomposition. In our experiment comparing manual labeling with labeling conducted with the support of Plud, we found that it reduces the time needed to label data and produces highly accurate models for this new domain.
Comments
The full paper can be found
The full paper can be found at https://ieeexplore.ieee.org/document/9191300