Documents
Presentation Slides
ColorFlow_ICASSP2024
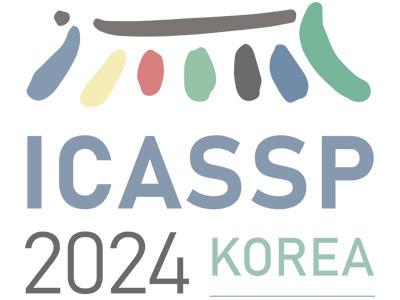
- Citation Author(s):
- Submitted by:
- Wang Yin
- Last updated:
- 15 April 2024 - 7:39pm
- Document Type:
- Presentation Slides
- Document Year:
- 2024
- Event:
- Presenters:
- Wang Yin
- Paper Code:
- IVMSP-L6.6
- Categories:
- Log in to post comments
Image colorization is an ill-posed task, as objects within grayscale images can correspond to multiple colors, motivating researchers to establish a one-to-many relationship between objects and colors. Previous work mostly could only create an insufficient deterministic relationship. Normalizing flow can fully capture the color diversity from natural image manifold. However, classical flow often overlooks the color correlations between different objects, resulting in generating unrealistic color. To solve this issue, we propose a conditional normalizing flow, named ColorFlow, to jointly learn the one-to-many relationships between objects and colors, and the color correlations between different objects within image. To represent these color correlations in flow, we design a color distribution predictor to estimate the global color histogram of grayscale image as global tones, which is utilized as the mean value of flow's latent variables. Experiments results show that ColorFlow outperforms state-of-the-art methods.
Comments
This is a great work.
This is a great work.