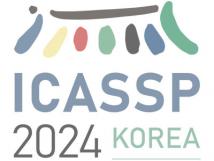
IEEE ICASSP 2024 - IEEE International Conference on Acoustics, Speech and Signal Processing (ICASSP) is the world’s largest and most comprehensive technical conference focused on signal processing and its applications. The IEEE ICASSP 2024 conference will feature world-class presentations by internationally renowned speakers, cutting-edge session topics and provide a fantastic opportunity to network with like-minded professionals from around the world. Visit the website.
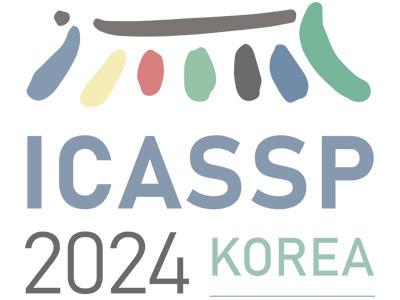
- Read more about [Poster] A Variable Smoothing for Nonconvexly Constrained Nonsmooth Optimization with Application to Sparse Spectral Clustering
- Log in to post comments
We propose a variable smoothing algorithm for solving nonconvexly constrained nonsmooth optimization problems. The target problem has two issues that need to be addressed: (i) the nonconvex constraint and (ii) the nonsmooth term. To handle the nonconvex constraint, we translate the target problem into an unconstrained problem by parameterizing the nonconvex constraint in terms of a Euclidean space. We show that under a certain condition, these problems are equivalent in view of finding a stationary point.
- Categories:
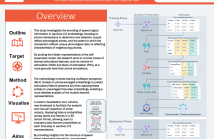
- Read more about FOLLOWING THE EMBEDDING: IDENTIFYING TRANSITION PHENOMENA IN WAV2VEC 2.0 REPRESENTATIONS OF SPEECH AUDIO
- Log in to post comments
Although transformer-based models have improved the state-of-the-art in speech recognition, it is still not well understood what information from the speech signal these models encode in their latent representations. This study investigates the potential of using labelled data (TIMIT) to probe wav2vec 2.0 embeddings for insights into the encoding and visualisation of speech signal information at phone boundaries. Our experiment involves training probing models to detect phone-specific articulatory features in the hidden layers based on IPA classifications.
- Categories:
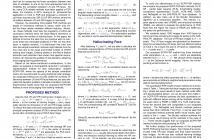
- Read more about LEARNING SPECTRAL CANONICAL F-CORRELATION REPRESENTATION FOR FACE SUPER-RESOLUTION
- Log in to post comments
Face super-resolution (FSR) is a powerful technique for restoring high-resolution face images from the captured low-resolution ones with the assistance of prior information. Existing FSR methods based on explicit or implicit covariance matrices are difficult to reveal complex nonlinear relationships between features, as conventional covariance computation is essentially a linear operation process. Besides, the limited number of training samples and noise disturbance lead to the deviation of sample covariance matrices.
- Categories:

Optimal power flow is a critical optimization problem that allocates power to the generators in order to satisfy the demand at a minimum cost. This is a non-convex problem shown to be NP-hard. We use a graph neural network to learn a nonlinear function between the power demanded and the corresponding allocation. We learn the solution in an unsupervised manner, minimizing the cost directly. To consider the power system constraints, we propose a novel barrier method that is differentiable and works on initially infeasible points.
- Categories:
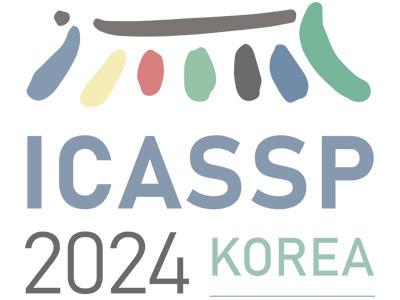
- Read more about Investigating End-to-end ASR Architectures for Long form Audio Transcription
- Log in to post comments
This paper presents an overview and evaluation of some of the end-to-end ASR models on long-form audios. We study three categories of Automatic Speech Recognition(ASR) models based on their core architecture: (1) convolutional, (2) convolutional with squeeze-and-excitation and (3) convolutional models with attention. We selected one ASR model from each category and evaluated Word Error Rate, maximum audio length and real-time factor for each model on a variety of long audio benchmarks: Earnings-21 and 22, CORAAL, and TED-LIUM3.
- Categories:
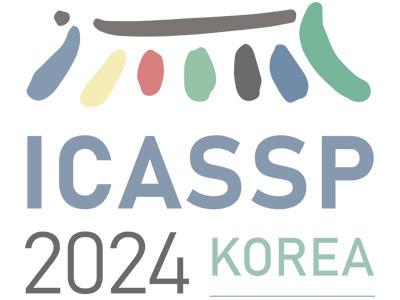
- Read more about DEMUCS for data-driven RF signal denoising
- Log in to post comments
In this paper, we present our radio frequency signal denoising approach, RFDEMUCS, for the 2024 IEEE ICASSP RF Signal Separation Challenge. Our approach is based on the DEMUCS architecture [1], and has a U-Net structure with a bidirectional LSTM bottleneck. For the task of estimating the underlying bit-sequence message, we also propose an extension of the DEMUCS that directly estimates the bits. Evaluations of the presented methods on the challenge test dataset yield MSE and BER scores of −118.71 and −81, respectively, according to the evaluation metrics defined in the challenge.
- Categories:
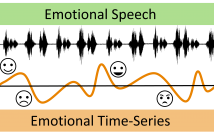
- Read more about Dynamic Speech Emotion Recognition using a Conditional Neural Process
- Log in to post comments
The problem of predicting emotional attributes from speech has often focused on predicting a single value from a sentence or short speaking turn. These methods often ignore that natural emotions are both dynamic and dependent on context. To model the dynamic nature of emotions, we can treat the prediction of emotion from speech as a time-series problem. We refer to the problem of predicting these emotional traces as dynamic speech emotion recognition. Previous studies in this area have used models that treat all emotional traces as coming from the same underlying distribution.
- Categories:
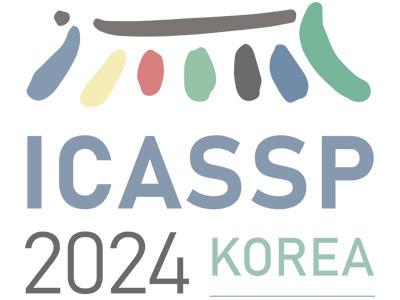
- Read more about FEATURE-CONSTRAINED AND ATTENTION-CONDITIONED DISTILLATION LEARNING FOR VISUAL ANOMALY DETECTION
- Log in to post comments
Visual anomaly detection in computer vision is an essential one-class classification and segmentation problem. The student-teacher (S-T) approach has proven effective in addressing this challenge. However, previous studies based on S-T underutilize the feature representations learned by the teacher network, which restricts anomaly detection performance.
- Categories:
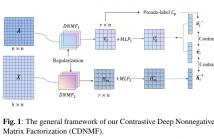
- Read more about [Poster] Contrastive Deep Nonnegative Matrix Factorization For Community Detection
- Log in to post comments
Recently, nonnegative matrix factorization (NMF) has been widely adopted for community detection, because of its better interpretability. However, the existing NMF-based methods have the following three problems: 1) they directly transform the original network into community membership space, so it is difficult for them to capture the hierarchical information; 2) they often only pay attention to the topology of the network and ignore its node attributes; 3) it is hard for them to learn the global structure information necessary for community detection.
- Categories:
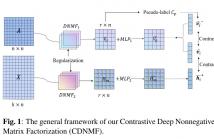
- Read more about [Poster] Contrastive Deep Nonnegative Matrix Factorization For Community Detection
- Log in to post comments
Recently, nonnegative matrix factorization (NMF) has been widely adopted for community detection, because of its better interpretability. However, the existing NMF-based methods have the following three problems: 1) they directly transform the original network into community membership space, so it is difficult for them to capture the hierarchical information; 2) they often only pay attention to the topology of the network and ignore its node attributes; 3) it is hard for them to learn the global structure information necessary for community detection.
ICASSP2024-Poster.pdf

- Categories: