Documents
Poster
COMPARING THE INFLUENCE OF DEPTH AND WIDTH OF DEEP NEURAL NETWORK BASED ON FIXED NUMBER OF PARAMETERS FOR AUDIO EVENT DETECTION
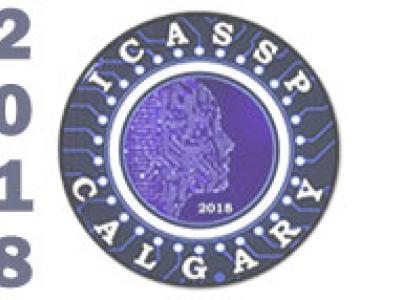
- Citation Author(s):
- Submitted by:
- Jun Wang
- Last updated:
- 19 April 2018 - 9:58pm
- Document Type:
- Poster
- Document Year:
- 2018
- Event:
- Paper Code:
- MLSP-P6.10
- Categories:
- Log in to post comments
Deep Neural Network (DNN) is a basic method used for the rare Acoustic Event Detection (AED) in synthesised audio. The structure of DNNs including Multi-Layer Perceptron (MLP) and Recurrent Neural Network (RNN) for AED tasks has rather fewer hidden layers compared with computer vision systems. This paper tries to demonstrate that a DNN with more hidden layers does not necessarily guarantee a better performance in AED tasks. Taking the rare AED in synthesised audio with MLPs as an example and simulating a fixed budget of memory in an embedded system, various structures of MLPs are tested with fixed number of parameters engaged. Comparing the importance of neuron numbers in a hidden layer (i.e. the width of DNNs) and the importance of layer numbers in DNNs (i.e. the depth of DNNs) for AED tasks, the performance of the candidate DNN systems are evaluated by the event-based error rate. The results illustrate that a shallower network may outperform a deeper network when enough parameters are engaged and a larger number of parameters introduces a better performance in general.