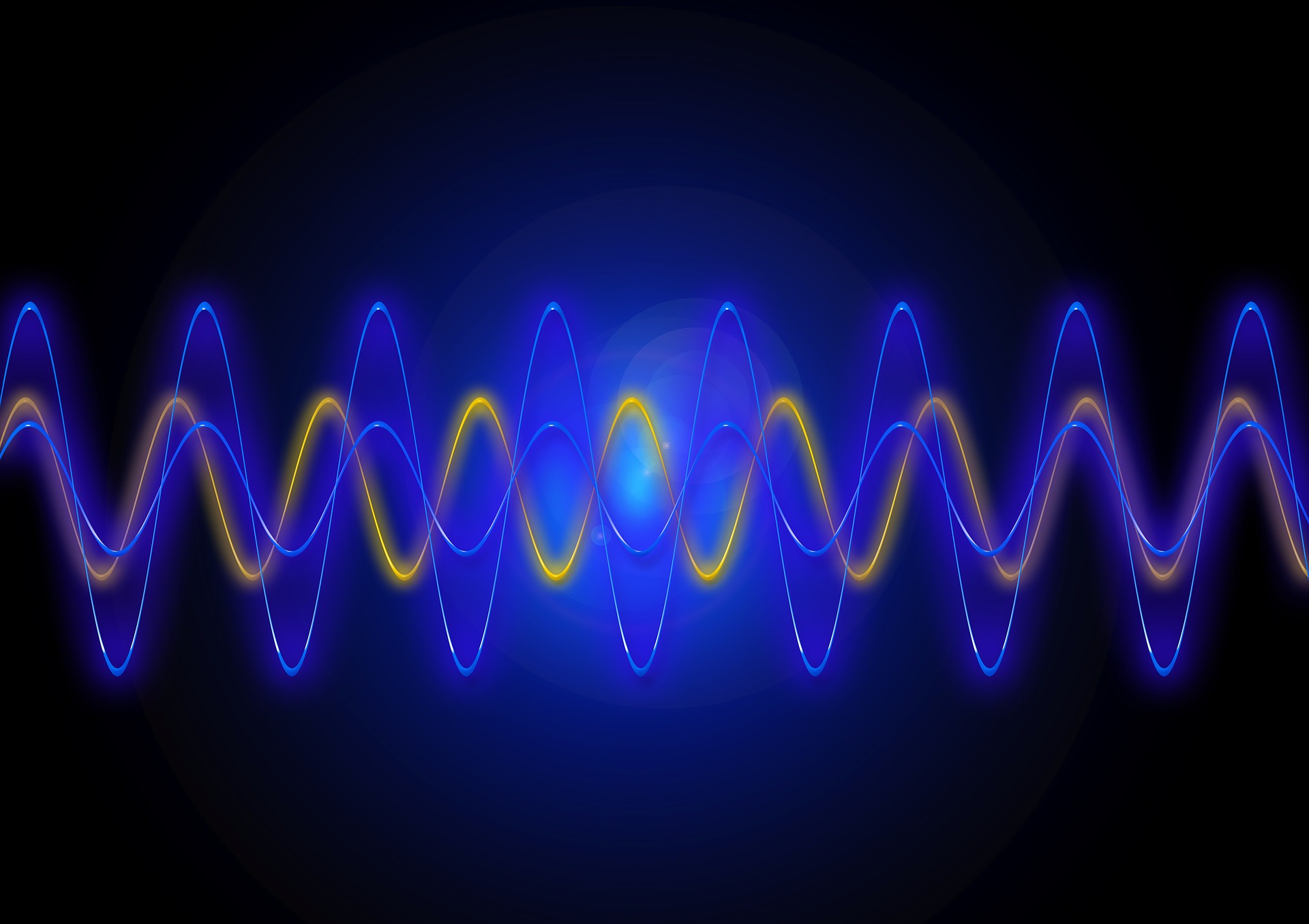
- Read more about MERTNet: Multi scale Efficient Residual Network encoder Transformer decoder Network Supplementary Materials
- Log in to post comments
Due to the page limitations of the main paper, we provide more visualizations and dataset test results here.
- Categories:
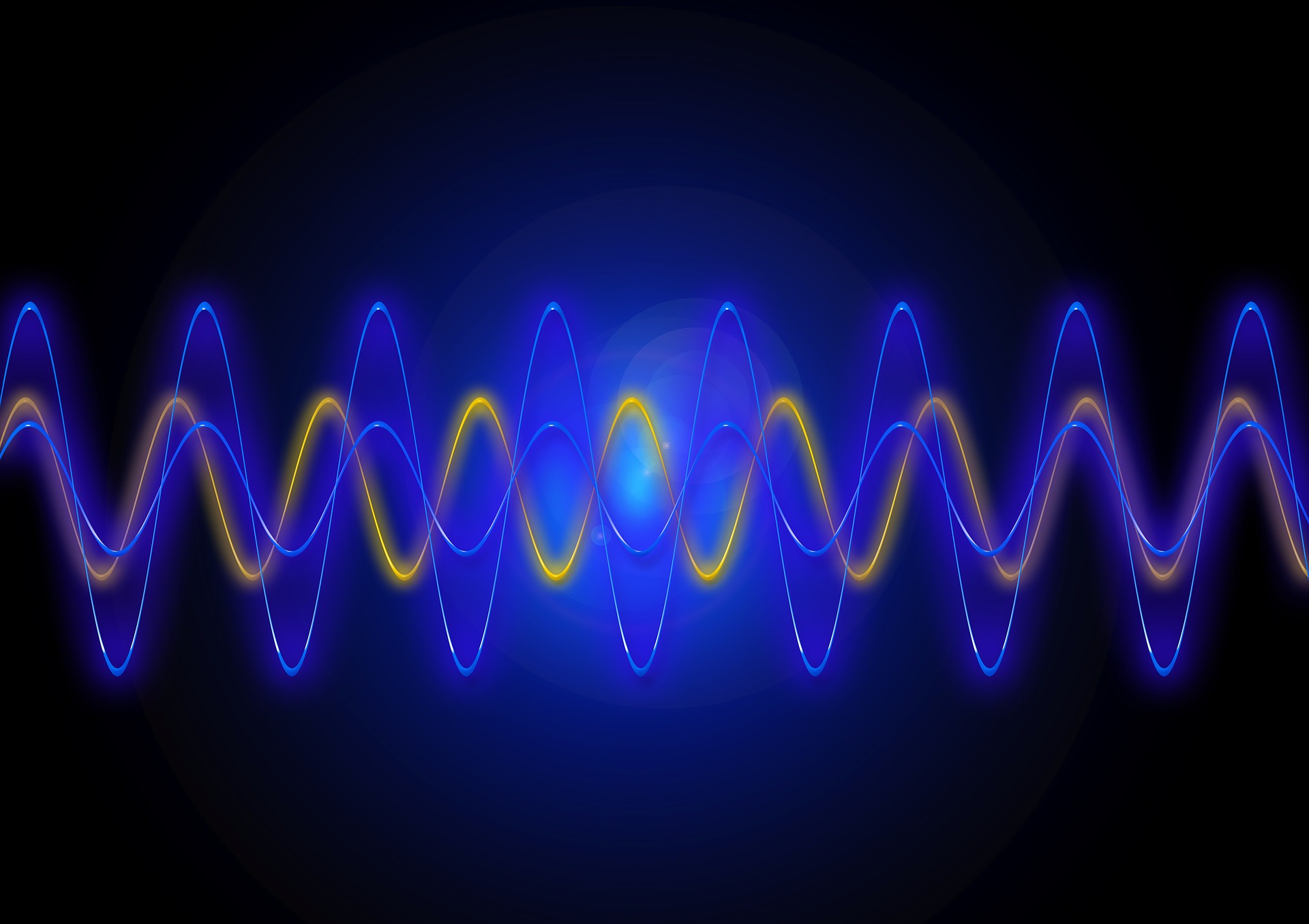
- Read more about Supplementary Material of Optimal Camera Placement for Dynamic Scenes via Reinforcement Learning in Virtual Environments
- Log in to post comments
In this supplementary material of our work, we provide additional experimental evaluation of the proposed Reinforcement Learning (RL) framework. For this purpose, a simple virtual environment of a four-walled room is exploited.
- Categories:
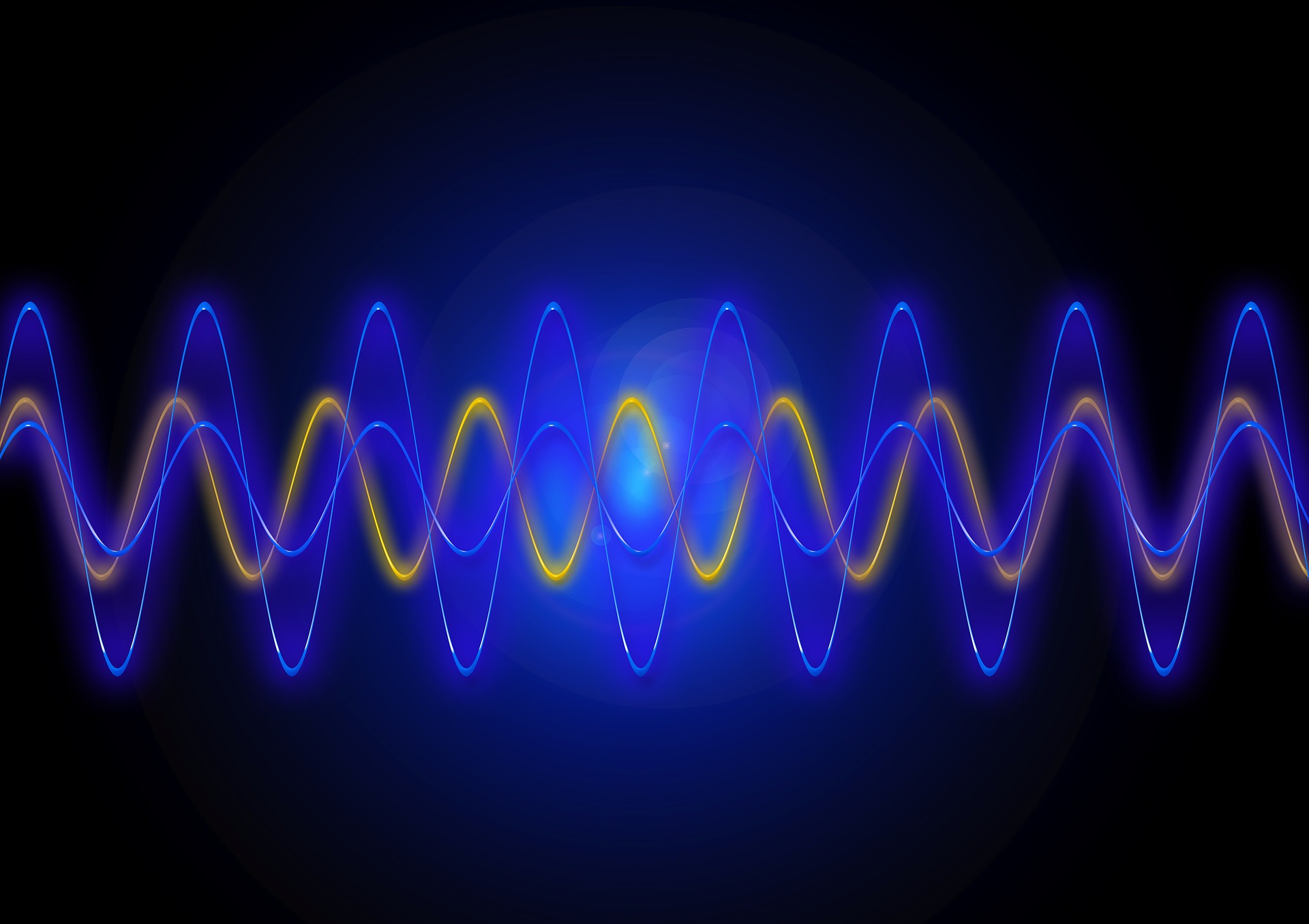
- Read more about Domain Generalization for 6D Pose Estimation Through NeRF-based Image Synthesis - Supplementary Material
- Log in to post comments
This work introduces a novel augmentation method that increases the diversity of a train set to improve the generalization abilities of a 6D pose estimation network. For this purpose, a Neural Radiance Field is trained on synthetic images and exploited to generate an augmented set. Our method enriches the initial set by enabling the synthesis of images with (i) unseen viewpoints, (ii) rich illumination conditions through appearance extrapolation, and (iii) randomized textures.
- Categories:
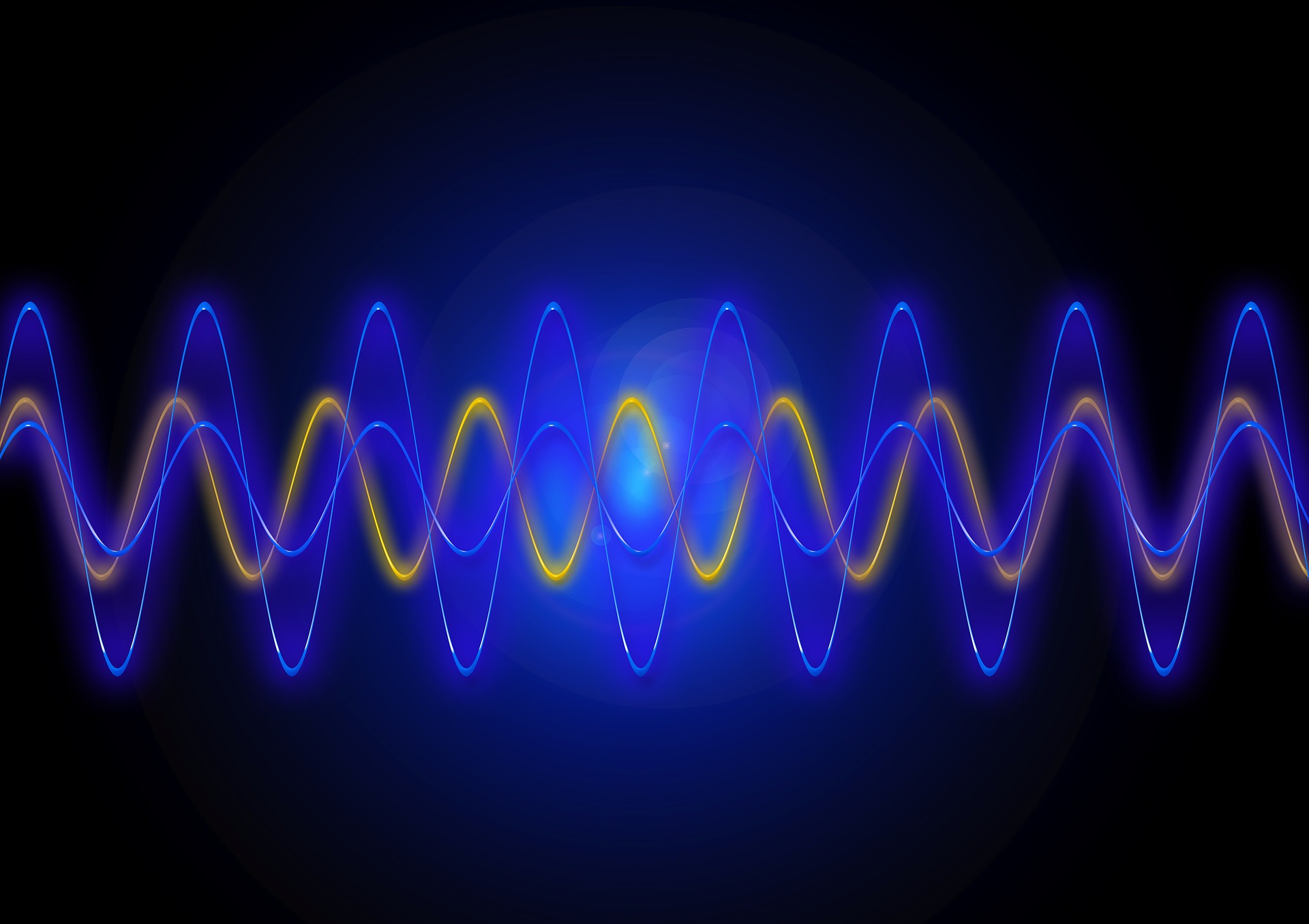
- Read more about Supplementary Material - Capture Stage Environments: A Guide to Better Matting
- Log in to post comments
Capture stages are high-end sources of state-of-the-art recordings for downstream applications in movies, games, and other media. One crucial step in almost all pipelines is the matting of images to isolate the captured performances from the background. While common matting algorithms deliver remarkable performance in other applications like teleconferencing and mobile entertainment, we found that they struggle significantly with the peculiarities of capture stage content.
- Categories:
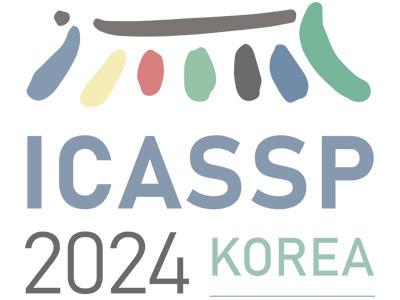
- Read more about SGT: SELF-GUIDED TRANSFORMER FOR FEW-SHOT SEMANTIC SEGMENTATION
- Log in to post comments
For the few-shot segmentation (FSS) task, existing methods
attempt to capture the diversity of new classes by fully uti-
lizing the limited support images, such as cross-attention and
prototype matching. However, they often overlook the fact
that there is variability in different regions of the same ob-
ject, and intra-image similarity is higher than inter-image sim-
ilarity.To address these limitations, a Self-Guided Trans-
former (SGT) is proposed by leveraging intra-image similar-
- Categories:
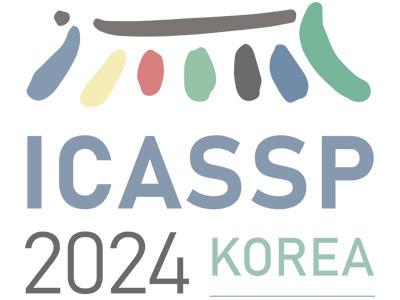
- Read more about Vision Transformer MST++: Efficient Hyperspectral Skin Reconstruction
- Log in to post comments
Channel reconstruction transforms a subsampled mutispectral image into hyperspectral, offering hyperspectral imaging benefits without a dedicated camera. MST++ is a
state of the art channel reconstruction technique, but it faces memory limitations for high spatial resolution images. In this context, we introduce VITMST++, a novel architecture in-
corporating Vision Transformer embedding and compression, multi-resolution image context and a channel-weighted loss. Developed for the ICASSP 2024 Hyperspectral Skin Chal-
- Categories:
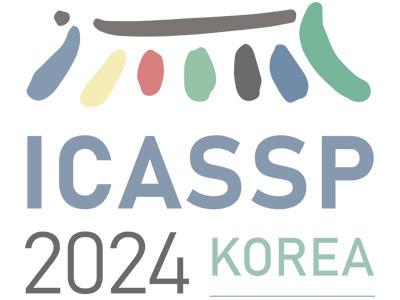
- Read more about JOINTLY LEARNING SELECTION MATRICES FOR TRANSMITTERS, RECEIVERS AND FOURIER COEFFICIENTS IN MULTICHANNEL IMAGING
- Log in to post comments
Strategic subsampling has become a focal point due to its effectiveness in compressing data, particularly in the Full Matrix Capture (FMC) approach in ultrasonic imaging. This paper introduces the Joint Deep Probabilistic Subsampling (J-DPS) method, which aims to learn optimal selection matrices simultaneously for transmitters, receivers, and Fourier coefficients. This task-based algorithm is realized by introducing a specialized measurement model and integrating a customized Complex Learned FISTA (CL-FISTA) network.
- Categories:
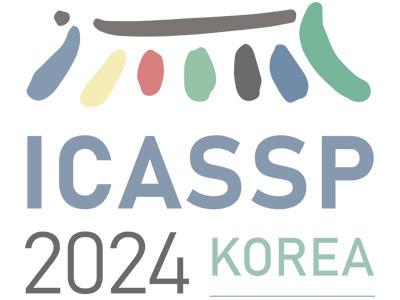
- Read more about Partially observable model-based learning for ISAC resource allocation
- Log in to post comments
This paper considers resource allocation problems for integrated sensing and communications (ISAC) systems operating in dynamic shared spectrum scenarios. Specifically, the paper proposes a new Model-Based Online Learning (MBOL) method that accounts for partial observability caused by noisy observations. First, the approach converts the partially observable Markov decision process (POMDP) to the equivalent belief state Markov decision process (MDP). Then, the state prediction model is learned from the sensor observations.
- Categories:
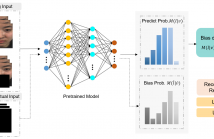
- Read more about CAUSALLY UNCOVERING BIAS IN VIDEO MICRO-EXPRESSION RECOGNITION
- Log in to post comments
Detecting microexpressions presents formidable challenges, primarily due to their fleeting nature and the limited diversity in existing datasets. Our studies find that these datasets exhibit a pronounced bias towards specific ethnicities and suffer from significant imbalances in terms of both class and gender representation among the samples. These disparities create fertile ground for various biases to permeate deep learning models, leading to skewed results and inadequate portrayal of specific demographic groups.
- Categories:
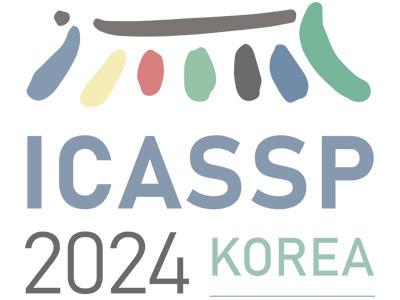
- Read more about Noisy-ArcMix: Additive Noisy Angular Margin Loss Combined With Mixup for Anomalous Sound Detection
- Log in to post comments
Unsupervised anomalous sound detection (ASD) aims to identify anomalous sounds by learning the features of normal operational sounds and sensing their deviations. Recent approaches have focused on the self-supervised task utilizing the classification of normal data, and advanced models have shown that securing representation space for anomalous data is important through representation learning yielding compact intra-class and well-separated intra-class distributions.
- Categories: