Documents
Poster
A Comparison of Boosted Deep Neural Networks for Voice Activity Detection
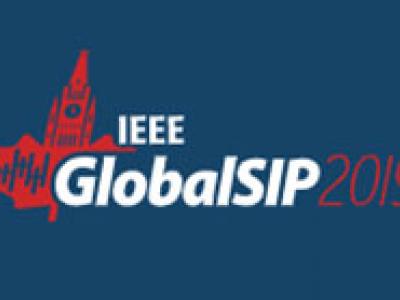
- Citation Author(s):
- Submitted by:
- Donald Williamson
- Last updated:
- 12 November 2019 - 10:09pm
- Document Type:
- Poster
- Document Year:
- 2019
- Event:
- Presenters:
- Donald S. Williamson
- Paper Code:
- 1570567217
- Categories:
- Log in to post comments
Voice activity detection (VAD) is an integral part of speech processing for real world problems, and a lot of work has been done to improve VAD performance. Of late, deep neural networks have been used to detect the presence of speech and this has offered tremendous gains. Unfortunately, these efforts have been either restricted to feed-forward neural networks that do not adequately capture frequency and temporal correlations, or the recurrent architectures have not been adequately tested in noisy environments. In this paper, we investigate different neural network configurations for voice activity detection. More specifically, we explore solutions that incorporate multi-resolution stacking and ensemble learning using convolutional, long short-term memory (LSTM), and dilated convolutional neural network architectures. We evaluate our approach using various speech signals that are captured in different amounts of noise. Our results show that a multi-resolution ensemble approach using LSTM recurrent neural networks performs best. This is demonstrated for seen and unseen testing scenarios.
williamson.pdf
