Documents
Research Manuscript
Component-Wise Conditionally Unbiased Widely Linear MMSE Estimation
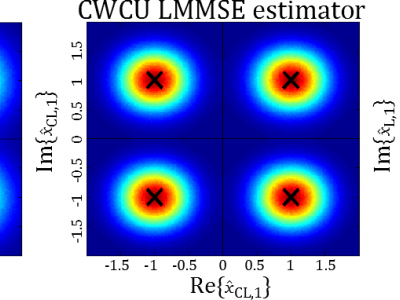
- Citation Author(s):
- Submitted by:
- Mario Huemer
- Last updated:
- 23 February 2016 - 1:44pm
- Document Type:
- Research Manuscript
- Document Year:
- 2016
- Categories:
- Log in to post comments
Biased estimators can outperform unbiased ones in terms of the mean square error (MSE). In this work we treat all estimators in the Bayesian framework, where the best linear unbiased estimator (BLUE) fulfills the so called global conditional unbiased constraint. Recently, component-wise conditionally unbiased linear minimum mean square error (CWCU LMMSE) estimators have been introduced. These estimators preserve a quite strong (namely the CWCU) unbiasedness condition which in effect sufficiently represents the intuitive view of unbiasedness, while in fact they are in general global conditionally biased. Overall, CWCU LMMSE estimators constitute an interesting compromise between the BLUE and the LMMSE estimator. We briefly recapitulate CWCU LMMSE estimation under linear model assumptions, and additionally derive the CWCU LMMSE estimator under the (only) assumption of jointly Gaussian parameters and measurements. The main intent of this work, however, is the extension of the theory of CWCU estimation to CWCU widely linear estimators. We derive the CWCU WLMMSE estimator for different model assumptions and address the analytical relationships between the CWCU WLMMSE and the WLMMSE estimators. The properties of CWCU WLMMSE estimators are deduced analytically, and compared to global conditionally unbiased as well as WLMMSE counterparts with the help of a parameter estimation application.