Documents
Presentation Slides
Presentation Slides
Compressive Image Recovery using Recurrent Generative Model
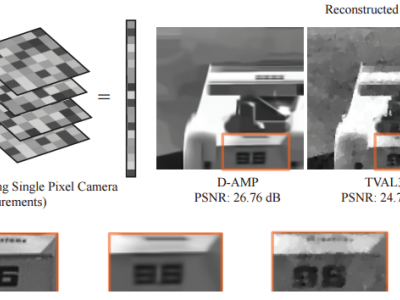
- Citation Author(s):
- Submitted by:
- Anil Kumar Vadathya
- Last updated:
- 26 September 2017 - 7:44am
- Document Type:
- Presentation Slides
- Document Year:
- 2017
- Event:
- Presenters:
- Anil Kumar Vadathya
- Paper Code:
- WP.L2
- Categories:
- Keywords:
- Log in to post comments
Reconstruction of signals from compressively sensed measurements is an ill-posed problem. In this paper, we leverage the recurrent generative model, RIDE, as an image prior for compressive image reconstruction. Recurrent networks can model long-range dependencies in images and hence are suitable to handle global multiplexing in reconstruction from compressive imaging. We perform MAP inference with RIDE using back-propagation to the inputs and projected gradient method. We propose an entropy thresholding based approach for preserving texture in images well. Our approach shows superior reconstructions compared to recent global reconstruction approaches like D-AMP and TVAL3 on both simulated and real data.