Documents
Presentation Slides
Confidence Estimation for Black Box Automatic Speech Recognition Systems Using Lattice Recurrent Neural Networks
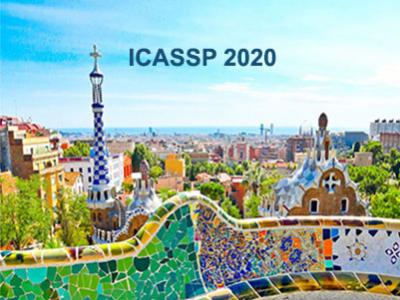
- Citation Author(s):
- Submitted by:
- Alexandros Kastanos
- Last updated:
- 14 May 2020 - 4:29pm
- Document Type:
- Presentation Slides
- Document Year:
- 2020
- Event:
- Presenters:
- Alexandros Kastanos
- Paper Code:
- 2710
- Categories:
- Log in to post comments
Recently, there has been growth in providers of speech transcription services enabling others to leverage technology they would not normally be able to use. As a result, speech-enabled solutions have become commonplace. Their success critically relies on the quality, accuracy, and reliability of the underlying speech transcription systems. Those black box systems, however, offer limited means for quality control as only word sequences are typically available. This paper examines this limited resource scenario for confidence estimation, a measure commonly used to assess transcription reliability. In particular, it explores what other sources of word and sub-word level information available in the transcription process could be used to improve confidence scores. To encode all such information this paper extends lattice recurrent neural networks to handle sub-words. Experimental results using the IARPA OpenKWS 2016 evaluation system show that the use of additional information yields significant gains in confidence estimation accuracy. The implementation for this model can be found online.