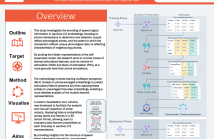
- Read more about FOLLOWING THE EMBEDDING: IDENTIFYING TRANSITION PHENOMENA IN WAV2VEC 2.0 REPRESENTATIONS OF SPEECH AUDIO
- Log in to post comments
Although transformer-based models have improved the state-of-the-art in speech recognition, it is still not well understood what information from the speech signal these models encode in their latent representations. This study investigates the potential of using labelled data (TIMIT) to probe wav2vec 2.0 embeddings for insights into the encoding and visualisation of speech signal information at phone boundaries. Our experiment involves training probing models to detect phone-specific articulatory features in the hidden layers based on IPA classifications.
- Categories:
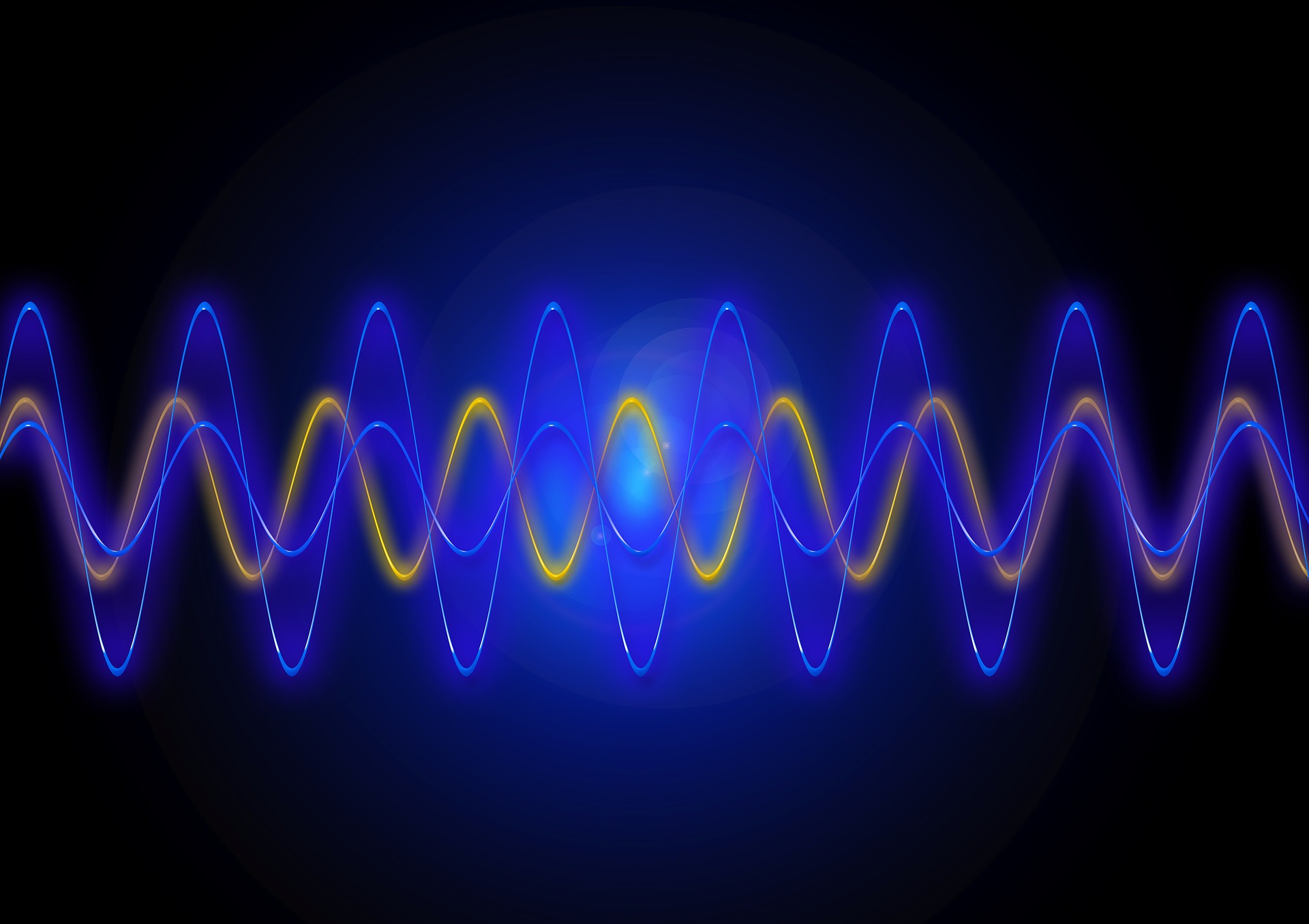
- Read more about BRAVEn: Improving Self-supervised Pre-training for Visual and Auditory Speech Recognition
- Log in to post comments
Self-supervision has recently shown great promise for learning visual and auditory speech representations from unlabelled data. In this work, we propose BRAVEn, an extension to the recent RAVEn method, which learns speech representations entirely from raw audio-visual data. Our modifications to RAVEn enable BRAVEn to achieve state-of-the-art results among self-supervised methods in various settings. Moreover, we observe favourable scaling behaviour by increasing the amount of unlabelled data well beyond other self-supervised works.
- Categories:
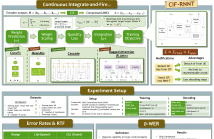
- Read more about CIF-RNNT: Streaming ASR via Acoustic Word Embeddings with Continuous Integrate-and-Fire and RNN-Transducers
- Log in to post comments
This paper introduces CIF-RNNT, a model that incorporates Continuous Integrate-and-Fire into RNN-Transducers (RNNTs) for streaming ASR via acoustic word embeddings (AWEs). CIF can dynamically compress long sequences into shorter ones, while RNNTs can produce multiple symbols given an input vector. We demonstrate that our model can not only streamingly segment acoustic information and produce AWEs, but also recover the represented word using a fixed set of output tokens with a shorter decoding time.
- Categories:
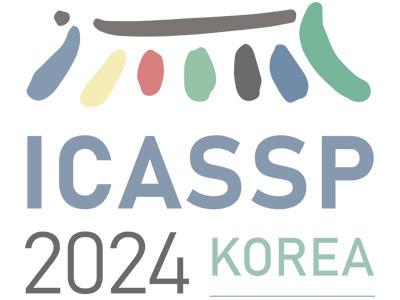
- Read more about INVESTIGATING THE CLUSTERS DISCOVERED BY PRE-TRAINED AV-HUBERT
- Log in to post comments
Self-supervised models, such as HuBERT and its audio-visual version AV-HuBERT, have demonstrated excellent performance on various tasks. The main factor for their success is the pre-training procedure, which requires only raw data without human transcription. During the self-supervised pre-training phase, HuBERT is trained to discover latent clusters in the training data, but these clusters are discarded, and only the last hidden layer is used by the conventional finetuning step.
- Categories:
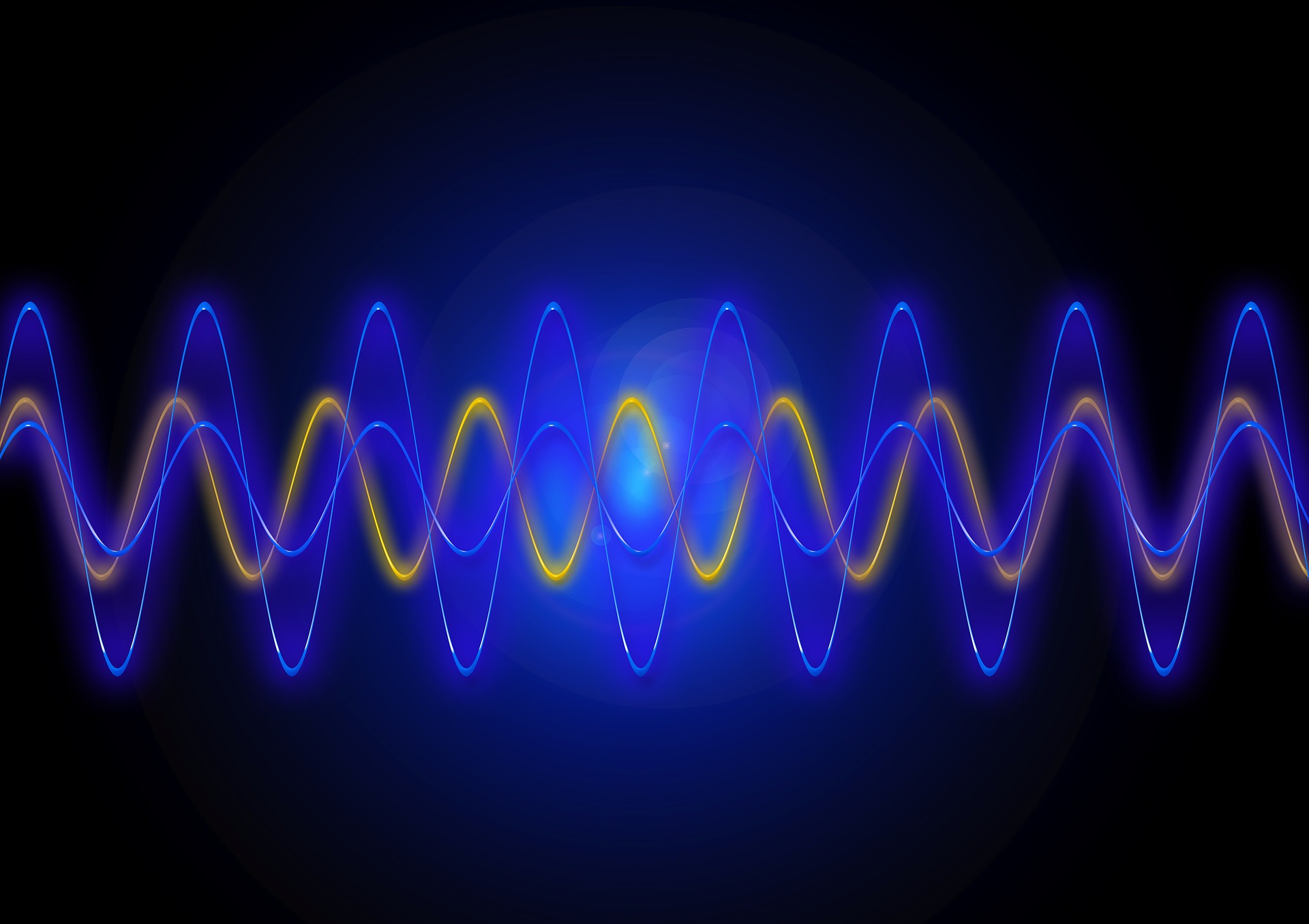
- Read more about USM-Lite: Quantization And Sparsity Aware Fine-Tuning For Speech Recognition With Universal Speech Models
- Log in to post comments
End-to-end automatic speech recognition (ASR) models have seen revolutionary quality gains with the recent development of large-scale universal speech models (USM). However, deploying these massive USMs is extremely expensive due to the enormous memory usage and computational cost. Therefore, model compression is an important research topic to fit USM-based ASR under budget in real-world scenarios.
- Categories:
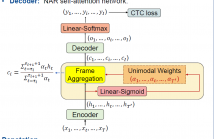
This paper works on non-autoregressive automatic speech recognition. A unimodal aggregation (UMA) is proposed to segment and integrate the feature frames that belong to the same text token, and thus to learn better feature representations for text tokens. The frame-wise features and weights are both derived from an encoder. Then, the feature frames with unimodal weights are integrated and further processed by a decoder. Connectionist temporal classification (CTC) loss is applied for training.
- Categories:
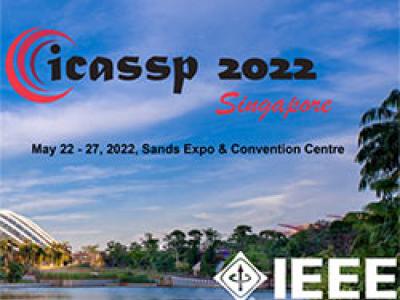
- Read more about ENABLING ON-DEVICE TRAINING OF SPEECH RECOGNITION MODELS WITH FEDERATED DROPOUT
- Log in to post comments
Federated learning can be used to train machine learning models on the edge on local data that never leave devices, providing privacy by default. This presents a challenge pertaining to the communication and computation costs associated with clients’ devices. These costs are strongly correlated with the size of the model being trained, and are significant for state-of-the-art automatic speech recognition models.We propose using federated dropout to reduce the size of client models while training a full-size model server-side.
- Categories:
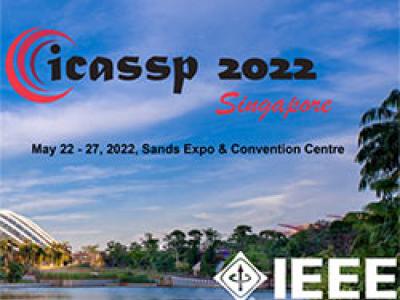
- Read more about ENABLING ON-DEVICE TRAINING OF SPEECH RECOGNITION MODELS WITH FEDERATED DROPOUT
- Log in to post comments
Federated learning can be used to train machine learning models on the edge on local data that never leave devices, providing privacy by default. This presents a challenge pertaining to the communication and computation costs associated with clients’ devices. These costs are strongly correlated with the size of the model being trained, and are significant for state-of-the-art automatic speech recognition models.We propose using federated dropout to reduce the size of client models while training a full-size model server-side.
- Categories:
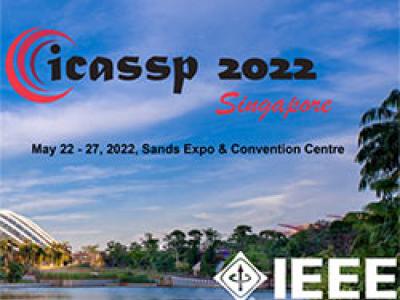
- Read more about SPE-89.4: UNSUPERVISED DATA SELECTION FOR SPEECH RECOGNITION WITH CONTRASTIVE LOSS RATIOS
- Log in to post comments
This paper proposes an unsupervised data selection method by using a submodular function based on contrastive loss ratios of target and training data sets. A model using a contrastive loss function is trained on both sets. Then the ratio of frame-level losses for each model is used by a submodular function. By using the submodular function, a training set for automatic speech recognition matching the target data set is selected.
- Categories:
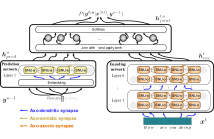
- Read more about Speech Recognition Using Biologically-Inspired Neural Networks
- Log in to post comments
Automatic speech recognition systems (ASR), such as the recurrent neural network transducer (RNN-T), have reached close to human-like performance and are deployed in commercial applications. However, their core operations depart from the powerful biological counterpart, the human brain. On the other hand, the current developments in biologically-inspired ASR models lag behind in terms of accuracy and focus primarily on small-scale applications.
- Categories: