Documents
Poster
Counting dense objects in remote sensing images
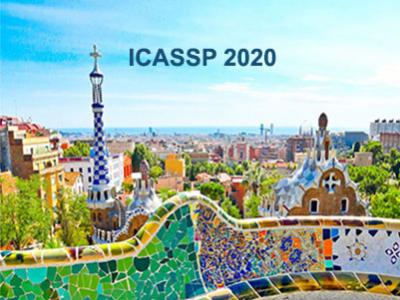
- Citation Author(s):
- Submitted by:
- Guangshuai Gao
- Last updated:
- 10 February 2020 - 10:31pm
- Document Type:
- Poster
- Document Year:
- 2020
- Event:
- Presenters:
- Guangshuai Gao
- Paper Code:
- 4709
- Categories:
- Log in to post comments
Estimating accurate number of interested objects from a given image is a challenging yet important task. Significant efforts have been made to address this problem and achieve great progress, yet counting number of ground objects from remote sensing images is barely studied. In this paper, we are interested in counting dense objects from remote sensing images. Compared with object counting in natural scene, this task is challenging in following factors: large scale variation, complex cluttered background and orientation arbitrariness. More importantly, the scarcity of data severely limits the development of research in this field. To address these issues, we first construct a large-scale object counting dataset based on remote sensing images, which contains four kinds of objects: buildings, crowded ships in harbor, large-vehicles and small-vehicles in parking lot. We then benchmark the dataset by designing a novel neural network which can generate density map of an input image. The proposed network consists of three parts namely convolution block attention module (CBAM), scale pyramid module (SPM) and deformable convolution module (DCM). Experiments on the proposed dataset and comparisons with state of the art methods demonstrate the challenging of the proposed dataset, and superiority and effectiveness of our method.