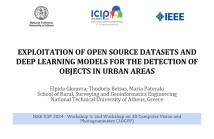
- Read more about EXPLOITATION OF OPEN SOURCE DATASETS AND DEEP LEARNING MODELS FOR THE DETECTION OF OBJECTS IN URBAN AREAS
- Log in to post comments
In this work we utilize different open-source datasets and deep learning models for detecting objects from image data captured by a mobile mapping system integrating the multi-camera Ladybug 5+ in an urban area. In our experiments
we exploit sets of pre-trained models and models trained via transfer learning techniques with available open source datasets for object detection, semantic-, instance-, and panoptic segmentation. Tests with the trained models are performed with image data from the Ladybug 5+ camera.
- Categories:
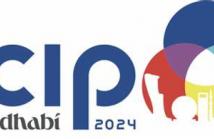
- Read more about LIGHTWEIGHT UNDERWATER IMAGE ENHANCEMENT VIA IMPULSE RESPONSE OF LOW-PASS FILTER BASED ATTENTION NETWORK
- Log in to post comments
In this paper, we propose an improved model of Shallow-UWnet for underwater image enhancement. In the proposed method, we enhance the learning process and solve the vanishing gradient problem by a skip connection, which concatenates the raw underwater image and the low-pass filter (LPF) impulse response into Shallow-UWnet. Additionally, we integrate the simple, parameter-free attention module (SimAM) into each Convolution Block to enhance the visual quality of images.
- Categories:
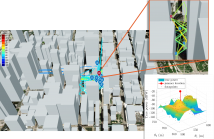
- Read more about JAMMING SOURCE LOCALIZATION USING AUGMENTED PHYSICS-BASED MODEL
- Log in to post comments
Monitoring interferences to satellite-based navigation systems is of paramount importance in order to reliably operate critical infrastructures, navigation systems, and a variety of applications relying on satellite-based positioning. This paper investigates the use of crowdsourced data to achieve such detection and monitoring at a central node that receives the data from an arbitrary number of agents in an area of interest. Under ideal conditions, the pathloss model is used to compute the Cramér-Rao Bound of accuracy as well as the corresponding maximum likelihood estimator.
- Categories:
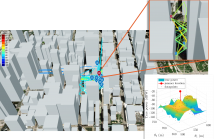
- Read more about JAMMING SOURCE LOCALIZATION USING AUGMENTED PHYSICS-BASED MODEL
- Log in to post comments
Monitoring interferences to satellite-based navigation systems is of paramount importance in order to reliably operate critical infrastructures, navigation systems, and a variety of applications relying on satellite-based positioning. This paper investigates the use of crowdsourced data to achieve such detection and monitoring at a central node that receives the data from an arbitrary number of agents in an area of interest. Under ideal conditions, the pathloss model is
- Categories:
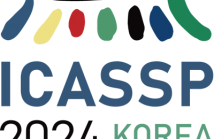
- Read more about PRE-ECHO REDUCTION IN TRANSFORM AUDIO CODING VIA TEMPORAL ENVELOPE CONTROL WITH MACHINE LEARNING BASED ESTIMATION
- Log in to post comments
This paper proposes a new method for pre-echo reduction in transform-based audio coding by controlling the temporal envelope of the waveform. The proposed method comprises two operating modes: temporal envelope flattening and temporal envelope correction of a target signal. The proposed method estimates signal levels with a low temporal resolution from side information using machine learning and converts them into a signal to be applied to the target signal to flatten and correct the temporal envelope.
- Categories:
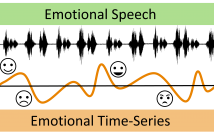
- Read more about Dynamic Speech Emotion Recognition using a Conditional Neural Process
- Log in to post comments
The problem of predicting emotional attributes from speech has often focused on predicting a single value from a sentence or short speaking turn. These methods often ignore that natural emotions are both dynamic and dependent on context. To model the dynamic nature of emotions, we can treat the prediction of emotion from speech as a time-series problem. We refer to the problem of predicting these emotional traces as dynamic speech emotion recognition. Previous studies in this area have used models that treat all emotional traces as coming from the same underlying distribution.
- Categories:
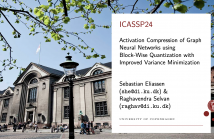
- Read more about MLSP-L18.6 presentation
- Log in to post comments
Efficient training of large-scale graph neural networks (GNNs) has been studied with a specific focus on reducing their memory consumption. Work by Liu et al. (2022) proposed extreme activation compression (EXACT) which demonstrated drastic reduction in memory consumption by performing quantization of the intermediate activation maps down to using INT2 precision. They showed little to no reduction in performance while achieving large reductions in GPU memory consumption.
- Categories:
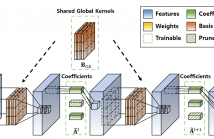
- Read more about G-SharP: Globally Shared Kernel with Pruning for Efficient CNNs
- Log in to post comments
Filter Decomposition (FD) methods have gained traction in compressing large neural networks by dividing weights into basis and coefficients. Recent advancements have focused on reducing weight redundancy by sharing either basis or coefficients stage-wise. However, traditional sharing approaches have overlooked the potential of sharing basis on a network-wide scale. In this study, we introduce an FD technique called G-SharP that elevates performance by using globally shared kernels throughout the network.
- Categories:
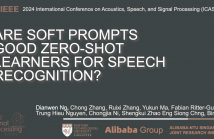
- Read more about Are Soft Prompts Good Zero-shot Learners for Speech Recognition?
- Log in to post comments
Large self-supervised pre-trained speech models require computationally expensive fine-tuning for downstream tasks. Soft prompt tuning offers a simple parameter-efficient alternative by utilizing minimal soft prompt guidance, enhancing portability while also maintaining competitive performance. However, not many people understand how and why this is so. In this study, we aim to deepen our understanding of this emerging method by investigating the role of soft prompts in automatic speech recognition (ASR).
- Categories:
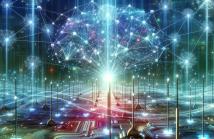
- Read more about State-Augmented Information Routing in Communication Systems with Graph Neural Networks
- Log in to post comments
We consider the problem of routing network packets in a large-scale communication system where the nodes have access to only local information. We formulate this problem as a constrained learning problem, which can be solved using a distributed optimization algorithm. We approach this distributed optimization using a novel state-augmentation (SA) strategy to maximize the aggregate information packets at different source nodes, leveraging dual variables corresponding to flow constraint violations.
- Categories: