Documents
Poster
Counting Network for Learning from Majority Label
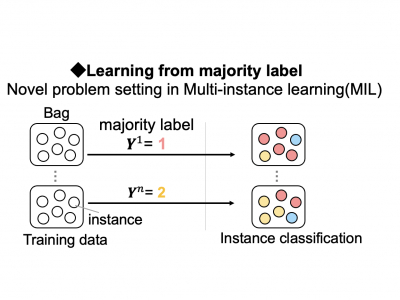
- DOI:
- 10.60864/35d1-7282
- Citation Author(s):
- Submitted by:
- Shiku Kaito
- Last updated:
- 6 June 2024 - 10:27am
- Document Type:
- Poster
- Document Year:
- 2024
- Presenters:
- Kaito Shiku
- Paper Code:
- MLSP-P38.5
- Categories:
- Keywords:
- Log in to post comments
The paper proposes a novel problem in multi-class Multiple-Instance Learning (MIL) called Learning from the Majority Label (LML). In LML, the majority class of instances in a bag is assigned as the bag's label. LML aims to classify instances using bag-level majority classes. This problem is valuable in various applications. Existing MIL methods are unsuitable for LML due to aggregating confidences, which may lead to inconsistency between the bag-level label and the label obtained by counting the number of instances for each class. This may lead to incorrect instance-level classification. We propose a counting network trained to produce the bag-level majority labels estimated by counting the number of instances for each class. This led to the consistency of the majority class between the network outputs and one obtained by counting the number of instances. Experimental results show that our counting network outperforms conventional MIL methods on four datasets.