Documents
Presentation Slides
Curriculum learning for speech emotion recognition from crowdsourced labels
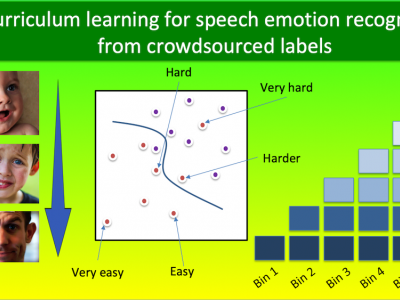
- Citation Author(s):
- Submitted by:
- Carlos Busso
- Last updated:
- 20 May 2020 - 9:43am
- Document Type:
- Presentation Slides
- Document Year:
- 2020
- Event:
- Presenters:
- Reza Lotfian
- Categories:
- Log in to post comments
This study introduces a method to design a curriculum for machine-learning to maximize the efficiency during the training process of deep neural networks (DNNs) for speech emotion recognition. Previous studies in other machine-learning problems have shown the benefits of training a classifier following a curriculum where samples are gradually presented in increasing level of difficulty. For speech emotion recognition, the challenge is to establish a natural order of difficulty in the training set to create the curriculum. We address this problem by assuming that ambiguous samples for humans are also ambiguous for computers. Speech samples are often annotated by multiple evaluators to account for differences in emotion perception across individuals. While some sentences with clear emotional content are consistently annotated, sentences with more ambiguous emotional content present important disagreement between individual evaluations. We propose to use the disagree- ment between evaluators as a measure of difficulty for the classification task. We propose metrics that quantify the inter- evaluation agreement to define the curriculum for regression problems and binary and multi-class classification problems. The experimental results consistently show that relying on a curriculum based on agreement between human judgments leads to statistically significant improvements over baselines trained without a curriculum.