Documents
Presentation Slides
DE NOVO MOLECULE GENERATION WITH GRAPH LATENT DIFFUSION MODEL
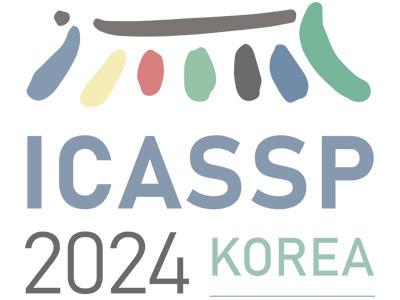
- DOI:
- 10.60864/qx45-kr37
- Citation Author(s):
- Submitted by:
- Conghao Wang
- Last updated:
- 6 June 2024 - 10:21am
- Document Type:
- Presentation Slides
- Document Year:
- 2024
- Event:
- Presenters:
- Yihao Chan
- Paper Code:
- BISP-L8.2
- Categories:
- Log in to post comments
De novo generation of molecules is a crucial task in drug discovery. The blossom of deep learning-based generative models, especially diffusion models, has brought forth promising advancements in de novo drug design by finding optimal molecules in a directed manner. However, due to the complexity of chemical space, existing approaches can only generate extremely small molecules. In this study, we propose a Graph Latent Diffusion Model (GLDM) that operates a diffusion model in the latent space modeled by a pretrained autoencoder. Applying diffusion processs on latent representations rather than original molecular graphs, GLDM improves training efficiency and enables generation of larger drug-like molecules. GLDM achieves state-of-the-art results on GuacaMol benchmarks.