Documents
Presentation Slides
Deep attractor networks for speaker re-identification and blind source separation
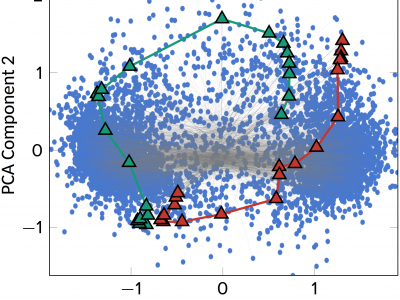
- Citation Author(s):
- Submitted by:
- Lukas Drude
- Last updated:
- 19 April 2018 - 7:00pm
- Document Type:
- Presentation Slides
- Document Year:
- 2018
- Event:
- Presenters:
- Lukas Drude
- Paper Code:
- 2012
- Categories:
- Log in to post comments
Deep Clustering (DC) and Deep Attractor Networks (DANs) are a data-driven way to monaural blind source separation.
Both approaches provide astonishing single channel performance but have not yet been generalized to block-online processing.
When separating speech in a continuous stream with a block-online algorithm, it needs to be determined in each block which of the output streams belongs to whom.
In this contribution we solve this block permutation problem by introducing an additional speaker identification embedding to the DAN model structure.
We motivate this model decision by analyzing the embedding topology of DC and DANs and show, that DC and DANs themselves are not sufficient for speaker identification.
This model structure (a) improves the SDR over a DAN baseline and (b) provides up to 61 % and up to 34 % relative reduction in permutation error rate and re-identification error rate compared to an i-vector baseline, respectively.