Documents
Poster
DEEP DECOMPOSITION OF CIRCULARLY SYMMETRIC GABOR WAVELET FOR ROTATION-INVARIANT TEXTURE IMAGE CLASSIFICATION
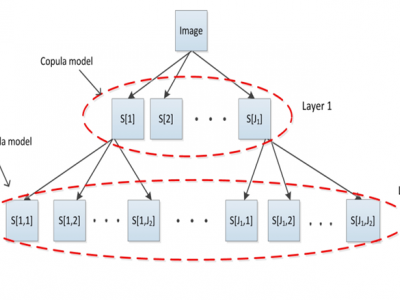
- Citation Author(s):
- Submitted by:
- li chaorong
- Last updated:
- 13 September 2017 - 10:06am
- Document Type:
- Poster
- Document Year:
- 2017
- Event:
- Paper Code:
- #3552
- Categories:
- Log in to post comments
We propose Deep Decomposition of Circularly Symmetric Gabor Wavelet (DD-CSGW) for rotation-invariant texture image classification. Circularly Symmetric Gabor Wavelet (CSGW) is rotation-invariant tool for image analysis. However, CSGW has an obvious shortcoming: it extracts less discriminative information from image due to lack of directional selectivity. We make two contributions to improve the performance of CSGW: (1) We propose deep decomposition approach of CSGW to obtain more discriminative image information; (2) Because strong scale dependencies exist in the domain of CSGW, we use copula model to capture these scale dependencies. For classification, the energies of DD-CSGW and the parameters of copula model based on DD-CSGW are used as the features of texture, and SVM is utilized as the classifier. Experiments show that DD-CSGW obviously improves the performance of CSGW, and it is effective compared with the state-of-the-art rotation-invariant methods.