Documents
Poster
DEEP FACTORIZATION FOR SPEECH SIGNAL
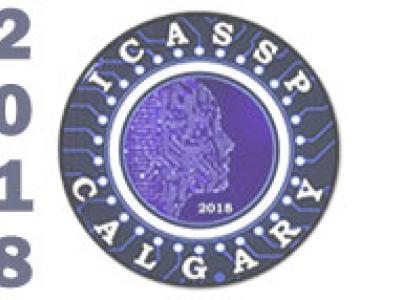
- Citation Author(s):
- Submitted by:
- Lantian Li
- Last updated:
- 20 April 2018 - 7:42am
- Document Type:
- Poster
- Document Year:
- 2018
- Event:
- Presenters:
- Miao Zhang
- Paper Code:
- 2969
- Categories:
- Log in to post comments
Various informative factors mixed in speech signals, leading to great difficulty when decoding any of the factors. An intuitive idea is to factorize each speech frame into individual informative factors, though it turns out to be highly difficult. Recently, we found that speaker traits, which were assumed to be long-term distributional properties, are actually short-time patterns, and can be learned by a carefully designed deep neural network (DNN). This discovery motivated a cascade deep factorization (CDF) framework that will be presented in this paper. The proposed framework infers speech factors in a sequential way, where factors previously inferred are used as conditional variables when inferring other factors. We will show that this approach can effectively factorize speech signals, and using these factors, the original speech spectrum can be recovered with a high accuracy. This factorization and reconstruction approach provides potential values for many speech processing tasks, e.g., speaker recognition and emotion recognition, as will be demonstrated in the paper.