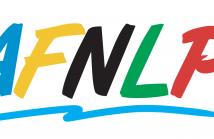
- Read more about Text-dependent Speaker Verification and RSR2015 Speech Corpus
- Log in to post comments
RSR2015 (Robust Speaker Recognition 2015) is the largest publicly available speech corpus for text-dependent robust speaker recognition. The current release includes 151 hours of short duration utterances spoken by 300 speakers. RSR2015 is developed by the Human Language Technology (HLT) department at Institute for Infocomm Research (I2R) in Singapore. This newsletter describes RSR2015 corpus that addresses the reviving interest of text-dependent speaker recognition.
RSR2015_v2.pdf

- Categories:
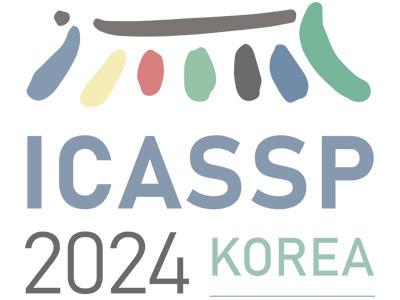
- Read more about TB-RESNET: BRIDGING THE GAP FROM TDNN TO RESNET IN AUTOMATIC SPEAKER VERIFICATION WITH TEMPORAL-BOTTLENECK ENHANCEMENT
- Log in to post comments
This paper focuses on the transition of automatic speaker verification systems from time delay neural networks (TDNN) to ResNet-based networks. TDNN-based systems use a statistics pooling layer to aggregate temporal information which is suitable for two-dimensional tensors. Even though ResNet-based models produce three-dimensional tensors, they continue to incorporate the statistics pooling layer.
- Categories:
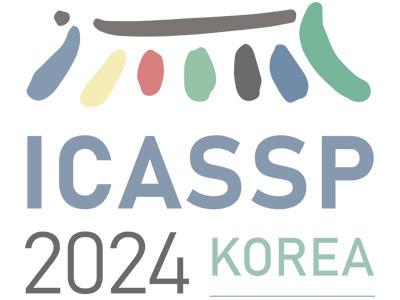
- Read more about SCORE CALIBRATION BASED ON CONSISTENCY MEASURE FACTOR FOR SPEAKER VERIFICATION
- Log in to post comments
This paper proposes a new scoring calibration method named ``Consistency-Aware Score Calibration", which introduces a Consistency Measure Factor (CMF) to measure the stability of audio voiceprints in similarity scores for speaker verification. The CMF is inspired by the limitations in segment scoring, where the segments with shorter length are not friendly to calculate the similarity score.
- Categories:
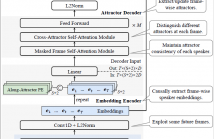
- Read more about Frame-wise streaming end-to-end speaker diarization with non-autoregressive self-attention-based attractors
- Log in to post comments
This work proposes a frame-wise online/streaming end-to-end neural diarization (FS-EEND) method in a frame-in-frame-out fashion. To frame-wisely detect a flexible number of speakers and extract/update their corresponding attractors, we propose to leverage a causal speaker embedding encoder and an online non-autoregressive self-attention-based attractor decoder. A look-ahead mechanism is adopted to allow leveraging some future frames for effectively detecting new speakers in real time and adaptively updating speaker attractors.
- Categories:
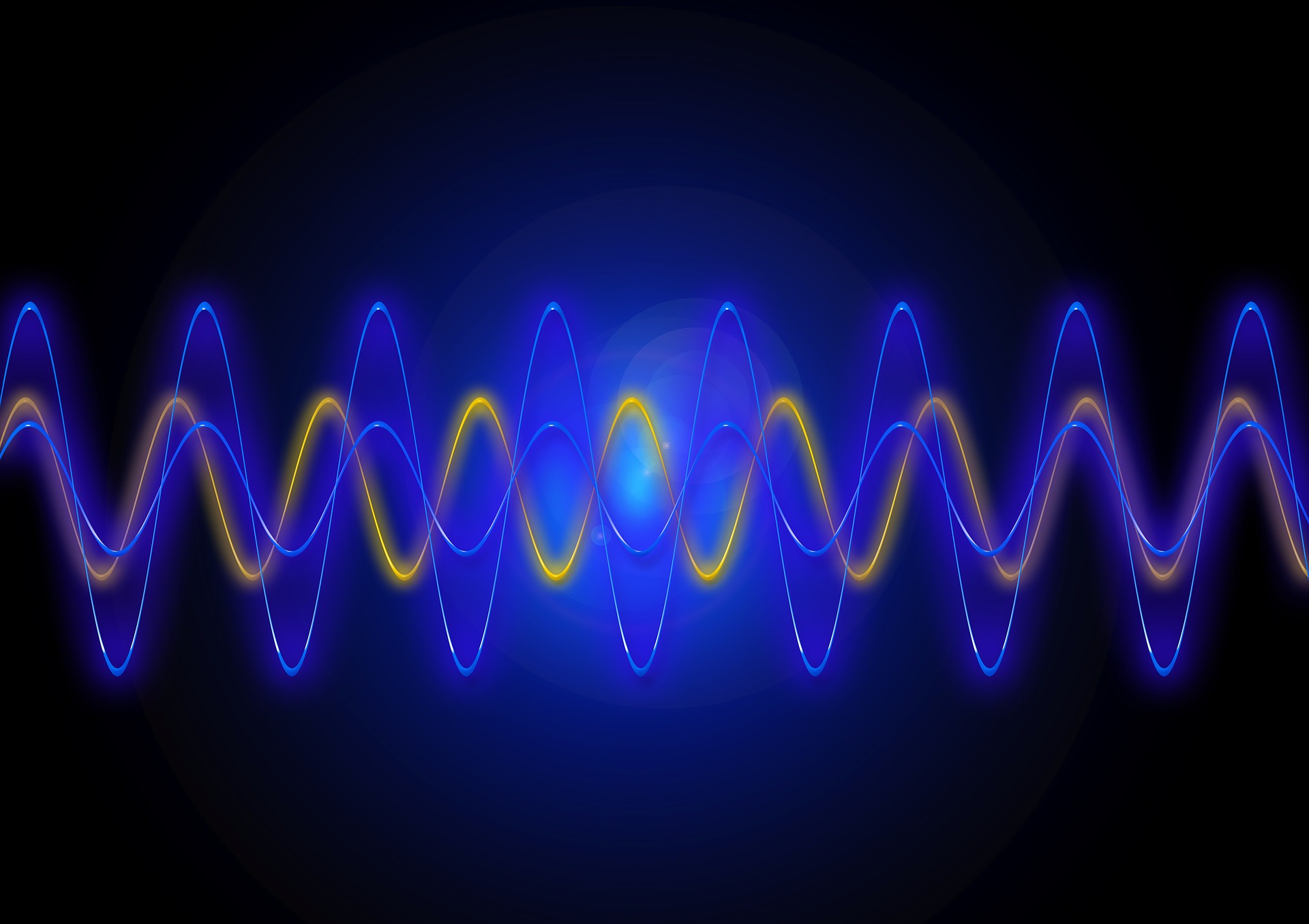
- Read more about CPAUG: REFINING COPY-PASTE AUGMENTATION FOR SPEECH ANTI-SPOOFING
- Log in to post comments
Conventional copy-paste augmentations generate new training instances by concatenating existing utterances to increase the amount of data for neural network training. However, the direct application of copy-paste augmentation for anti-spoofing is problematic. This paper refines the copy-paste augmentation for speech anti-spoofing, dubbed CpAug, to generate more training data with rich intra-class diversity. The CpAug employs two policies: concatenation to merge utterances with identical labels, and substitution to replace segments in an anchor utterance.
- Categories:
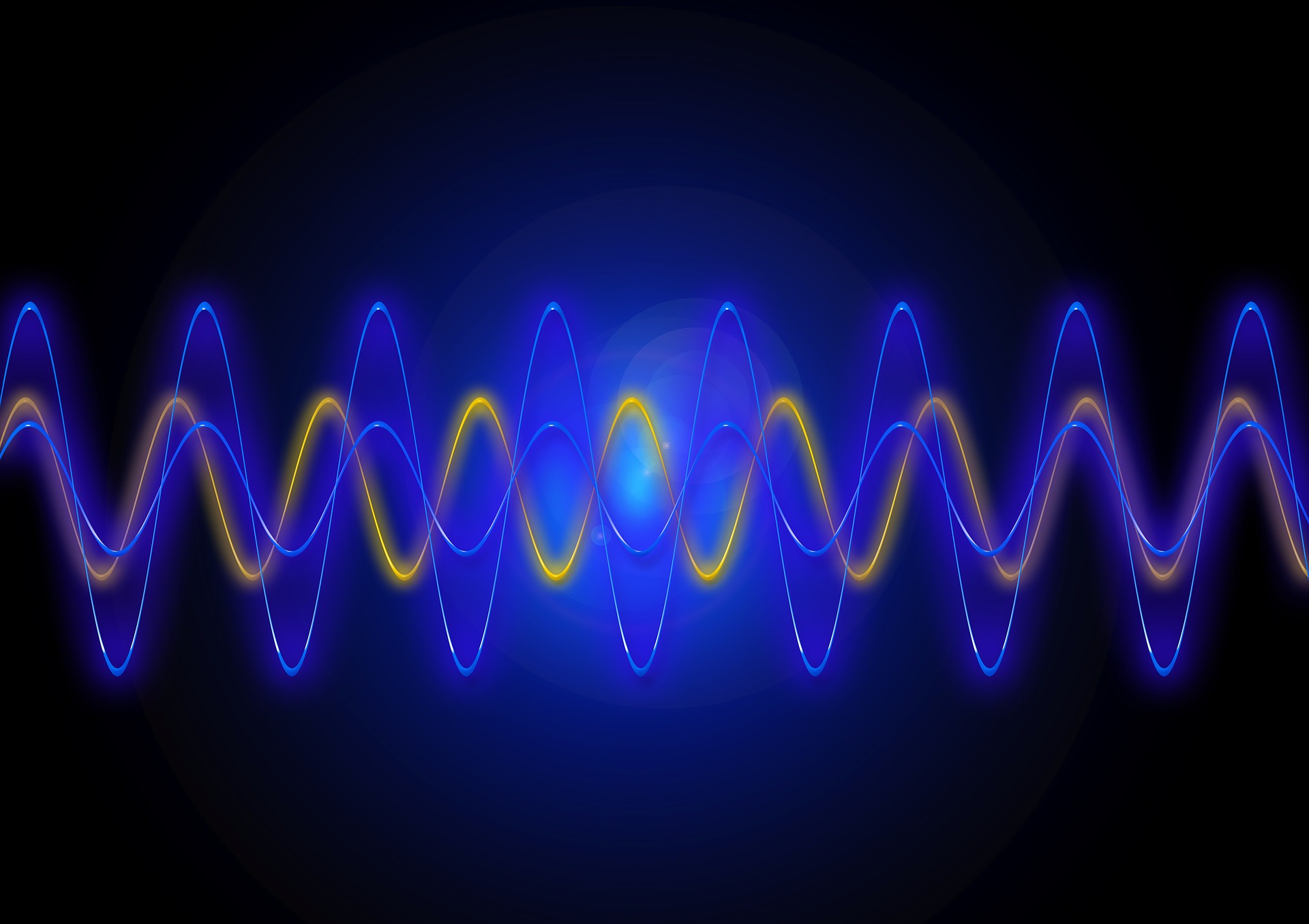
- Read more about CPAUG: REFINING COPY-PASTE AUGMENTATION FOR SPEECH ANTI-SPOOFING
- Log in to post comments
Conventional copy-paste augmentations generate new training instances by concatenating existing utterances to increase the amount of data for neural network training. However, the direct application of copy-paste augmentation for anti-spoofing is problematic. This paper refines the copy-paste augmentation for speech anti-spoofing, dubbed CpAug, to generate more training data with rich intra-class diversity. The CpAug employs two policies: concatenation to merge utterances with identical labels, and substitution to replace segments in an anchor utterance.
- Categories:
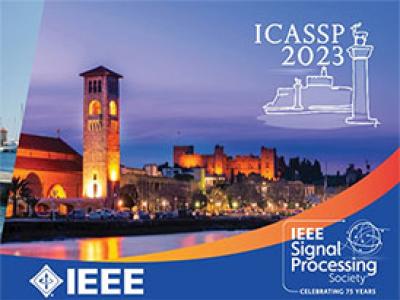
- Read more about Supervised Hierarchical Clustering Using Graph Neural Networks For Speaker Diarization
- Log in to post comments
- Categories:
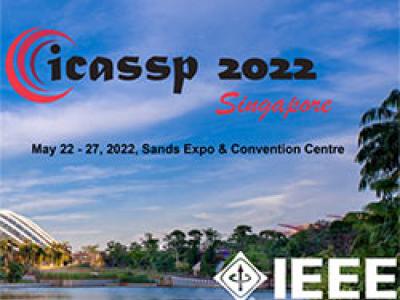
- Read more about Temporal Dynamic Convolutional Neural Network for Text-Independent Speaker Verification and Phonemic Analysis
- Log in to post comments
- Categories: