Documents
Supplementary Material
DEEP FEATURES BASED ON CONTRASTIVE FUSION OF TRANSFORMER AND CNN FOR SEMANTIC SEGMENTATION
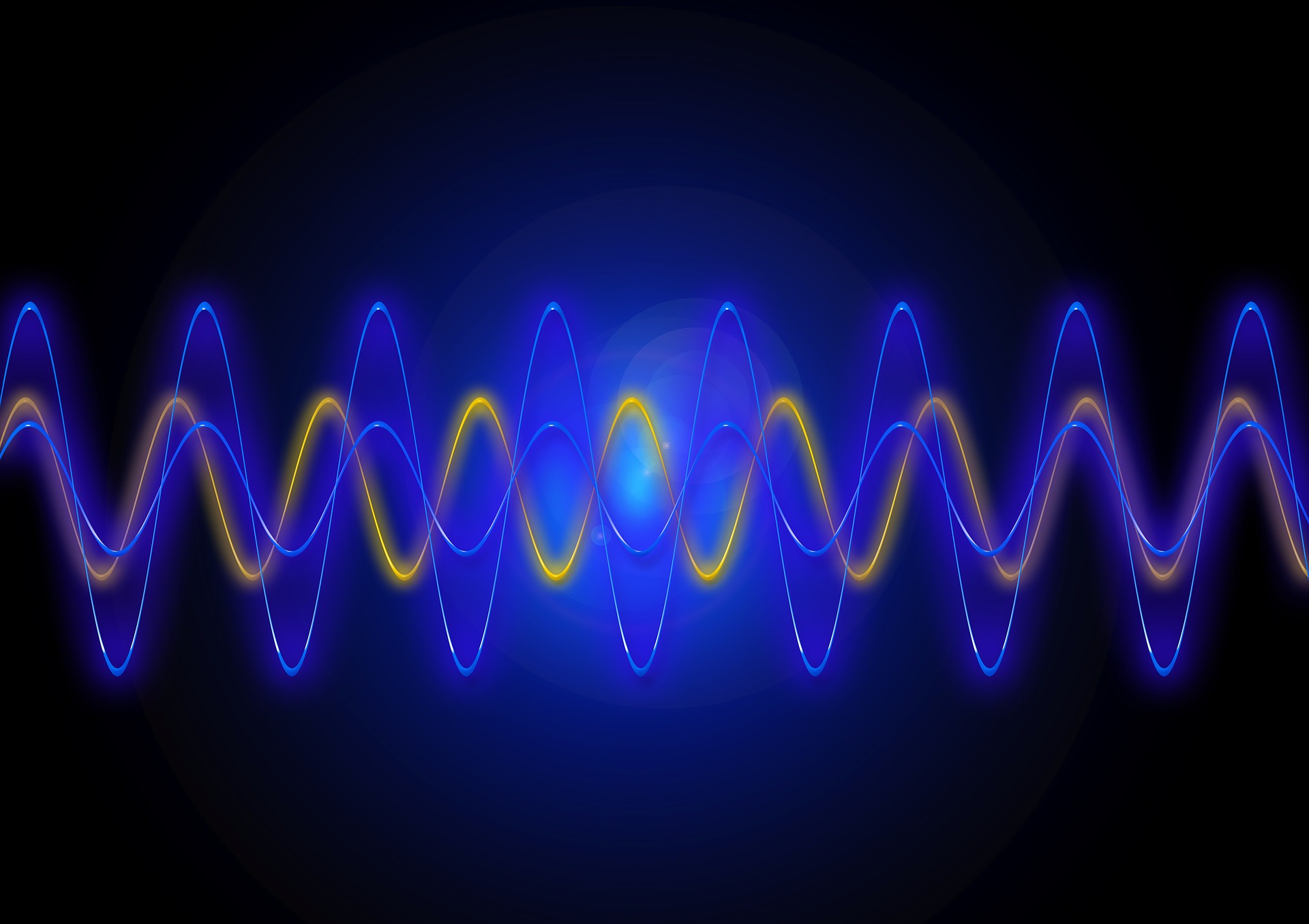
- DOI:
- 10.60864/8702-zg67
- Citation Author(s):
- Submitted by:
- Margi Pandya
- Last updated:
- 6 February 2025 - 1:16am
- Document Type:
- Supplementary Material
- Paper Code:
- 1739
- Categories:
- Log in to post comments
Image segmentation plays a crucial role in various computer vision applications for accurately identifying and extracting objects or regions of interest within an image. Despite significant advancements in state-of-the-art (SOTA) models for semantic segmentation, many still rely on additional and huge datasets to improve their performance. We introduce ContraFusionNet (CFN), a novel way of combining the spatial understanding property of Convolutional Neural Network (CNN) and deep feature extraction capability of attention in transformers for robust semantic segmentation even in limited data paradigms. It uses student-teacher learning with contrastive loss for improved feature assimilation. Notably, our model performs better than others when trained on the large Cityscape dataset without soliciting any external dataset. This is a significant step in reducing the data dependency for the segmentation task. We have also shown that when our proposed fusion strategy is applied to other SOTA methods, it leads to improving their performance.